The field of drug discovery is one of the most critical and challenging areas in medicine. The process of identifying potential therapeutic molecules is time-consuming, expensive, and fraught with uncertainty. However, in recent years, generative artificial intelligence (AI) has emerged as a transformative technology that has the potential to revolutionize drug discovery. By leveraging the power of machine learning algorithms, generative AI in drug discovery holds the promise of accelerating drug development, reducing costs, and, ultimately, saving lives.
What is generative AI? It is a subfield of artificial intelligence that focuses on creating new data instances that resemble existing data. It operates on the principles of unsupervised learning, where the AI model learns patterns from a dataset without explicit labels. This allows generative AI models to generate new samples, such as images, text, or in the context of drug discovery, molecular structures. For more information about generative AI, visit the related article.
Generative AI in drug discovery is becoming a lucrative sector. According to Precedence Research, By 2032, the worldwide market for generative AI in drug discovery market is expected to be worth roughly USD 1,417.83 million, up from its 2022 total of USD 126.07 million (a CAGR of 27.38 percent).
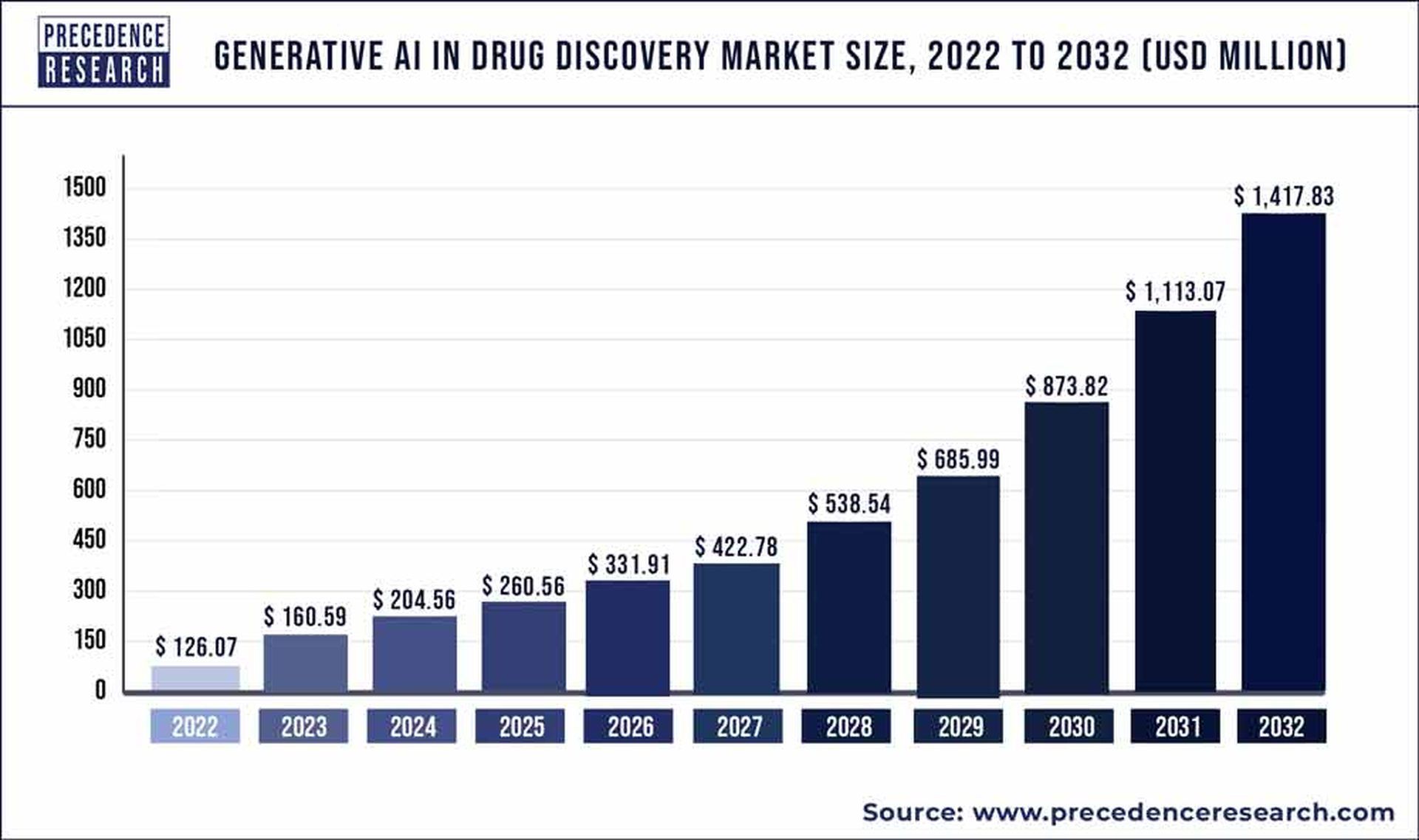
In this blog post, we will delve into the details of how generative AI is shaping the landscape of drug discovery, its applications, and the challenges it faces.
What is the role of generative AI in drug discovery?
The role of generative AI in drug discovery is to revolutionize and expedite the process of identifying potential therapeutic molecules. By leveraging machine learning algorithms such as generative adversarial networks (GANs), recurrent neural networks (RNNs), and graph neural networks (GNNs), generative AI can generate novel molecular structures, optimize drug designs, and facilitate de novo drug design. This capability allows researchers to explore a vast chemical space, identifying promising drug candidates that may have been overlooked using traditional methods.
Generative AI accelerates drug development, reduces costs, and offers the potential to improve patient outcomes by identifying effective and safe drugs in a shorter timeframe, making it a transformative tool in the field of drug discovery.
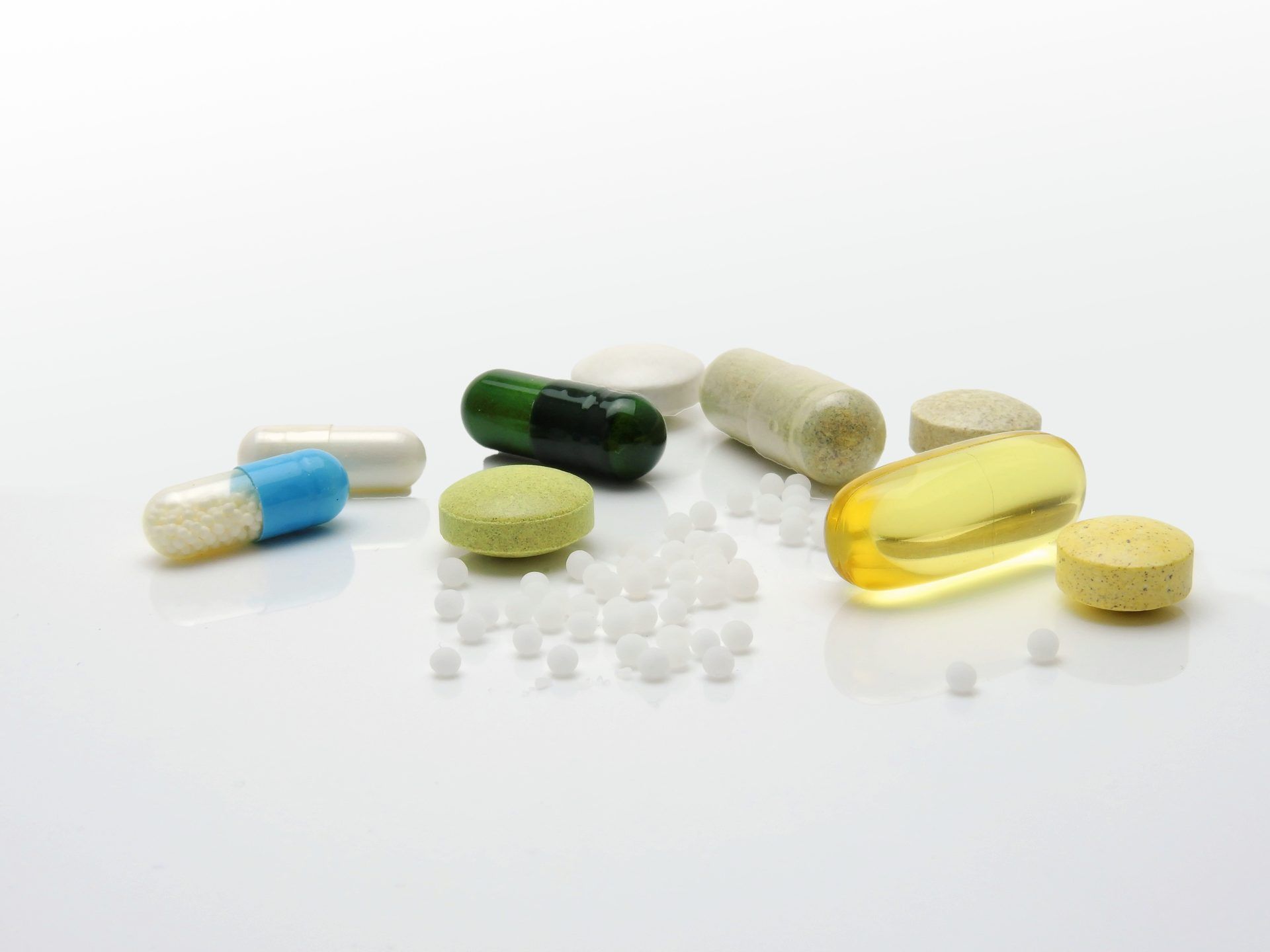
The conventional drug discovery process involves the identification of potential drug candidates through laborious trial-and-error experiments. Generative AI in drug discovery promises to revolutionize this process by augmenting traditional methods with computational efficiency and accuracy. Here’s how generative AI is being used in drug discovery:
- Molecule generation: Generative AI models, particularly those based on recurrent neural networks (RNNs) and generative adversarial networks (GANs), can generate novel molecular structures that adhere to specific criteria. This ability to generate a vast number of diverse molecules opens up new possibilities for identifying potential drug candidates that traditional methods may have overlooked.
- Drug design optimization: Generative AI can also assist in optimizing drug designs by generating modifications of existing compounds. By exploring variations in molecular structures, AI algorithms can identify modifications that enhance a drug’s efficacy, safety, and specificity, making the design process more efficient.
- De novo drug design: One of the most exciting applications of generative AI in drug discovery is de novo drug design. This involves designing entirely new molecules from scratch to target specific diseases. AI models can be trained on vast databases of known drugs and their properties, enabling them to predict molecular structures that are likely to exhibit desirable properties.
Popular techniques that use generative AI in drug discovery
Below are some key techniques used in AI-driven drug discovery, along with examples of their applications:
Generative Adversarial Networks (GANs)
GANs are a type of generative AI model that consists of two neural networks: a generator and a discriminator. The generator generates new data instances while the discriminator tries to distinguish between real and generated data. Through an adversarial training process, GANs can produce highly realistic and diverse samples. In drug discovery, GANs are employed for molecule generation, molecular optimization, and de novo drug design.
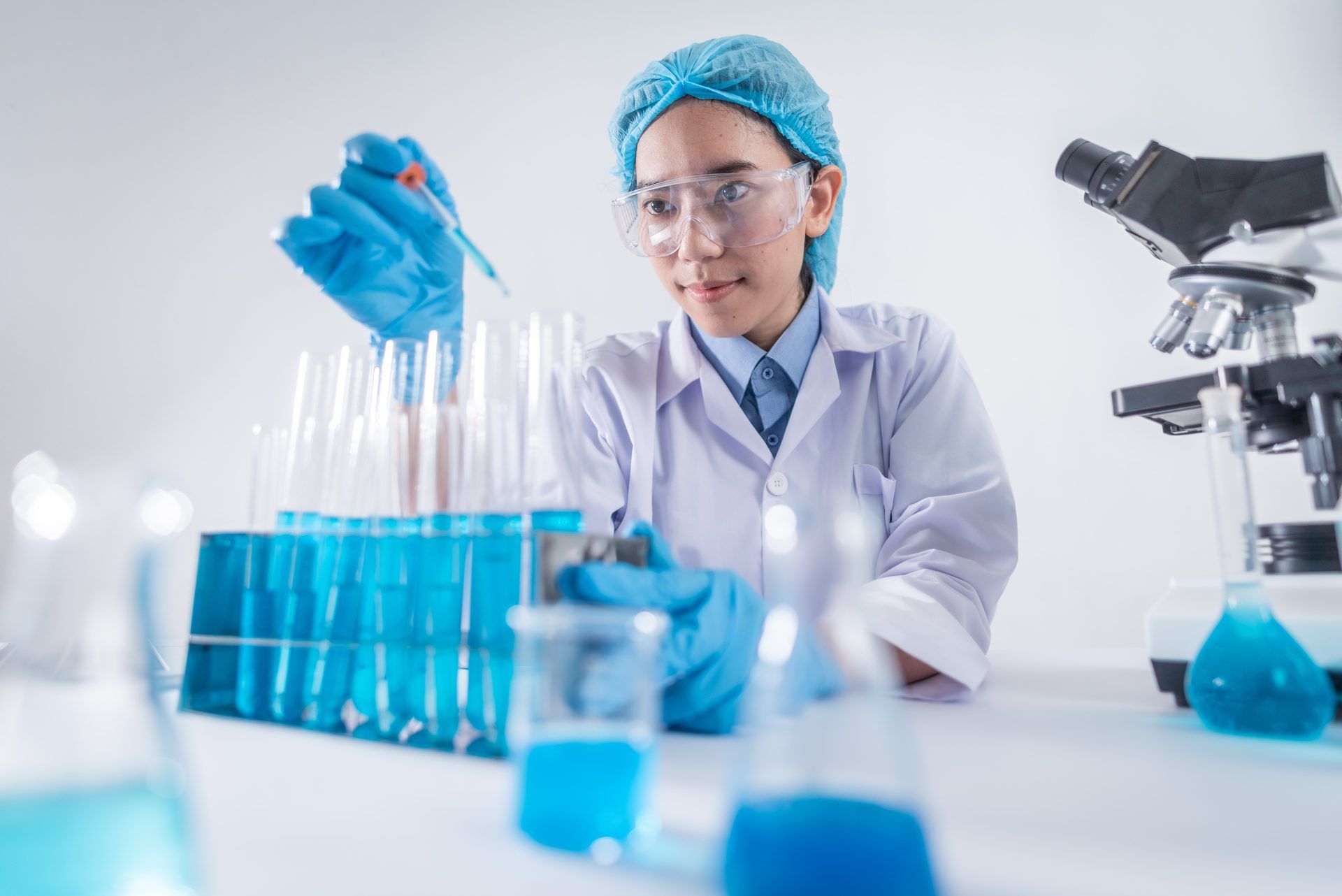
GANs have been used to generate novel small-molecule inhibitors of specific protein targets. Researchers at the University of North Carolina used GANs to generate potential inhibitors of the influenza A virus, leading to the identification of several promising candidates for further evaluation.
Reinforcement learning
Reinforcement learning is a type of machine learning in which an AI agent learns from interacting with an environment to achieve a specific goal. It receives feedback in the form of rewards or penalties, allowing it to learn which actions lead to favorable outcomes. In drug discovery, reinforcement learning is employed to optimize drug design and identify compounds with desired properties.
Researchers at the University of Cambridge used reinforcement learning to optimize drug candidates targeting Alzheimer’s disease. The AI agent learned to design molecules with improved target affinity and selectivity by receiving rewards for favorable molecular properties.
Graph Neural Networks (GNNs)
GNNs are a class of neural networks designed to work with graph-structured data, such as molecular graphs, where nodes represent atoms and edges represent chemical bonds. GNNs can learn patterns and interactions within molecular graphs, making them valuable for predicting molecular properties and activity.
Scientists at the University of California, San Francisco, used GNNs to predict the biological activity of small molecules against specific drug targets. By learning from large molecular databases, the GNN-based model accurately identified active compounds for various protein targets.
Transfer learning
Transfer learning is a technique where a model trained on one task is adapted or fine-tuned for a related task. It allows AI models to leverage knowledge from one domain to improve performance in another domain. In drug discovery, transfer learning is employed to transfer knowledge from datasets of known drug-target interactions to predict new interactions.
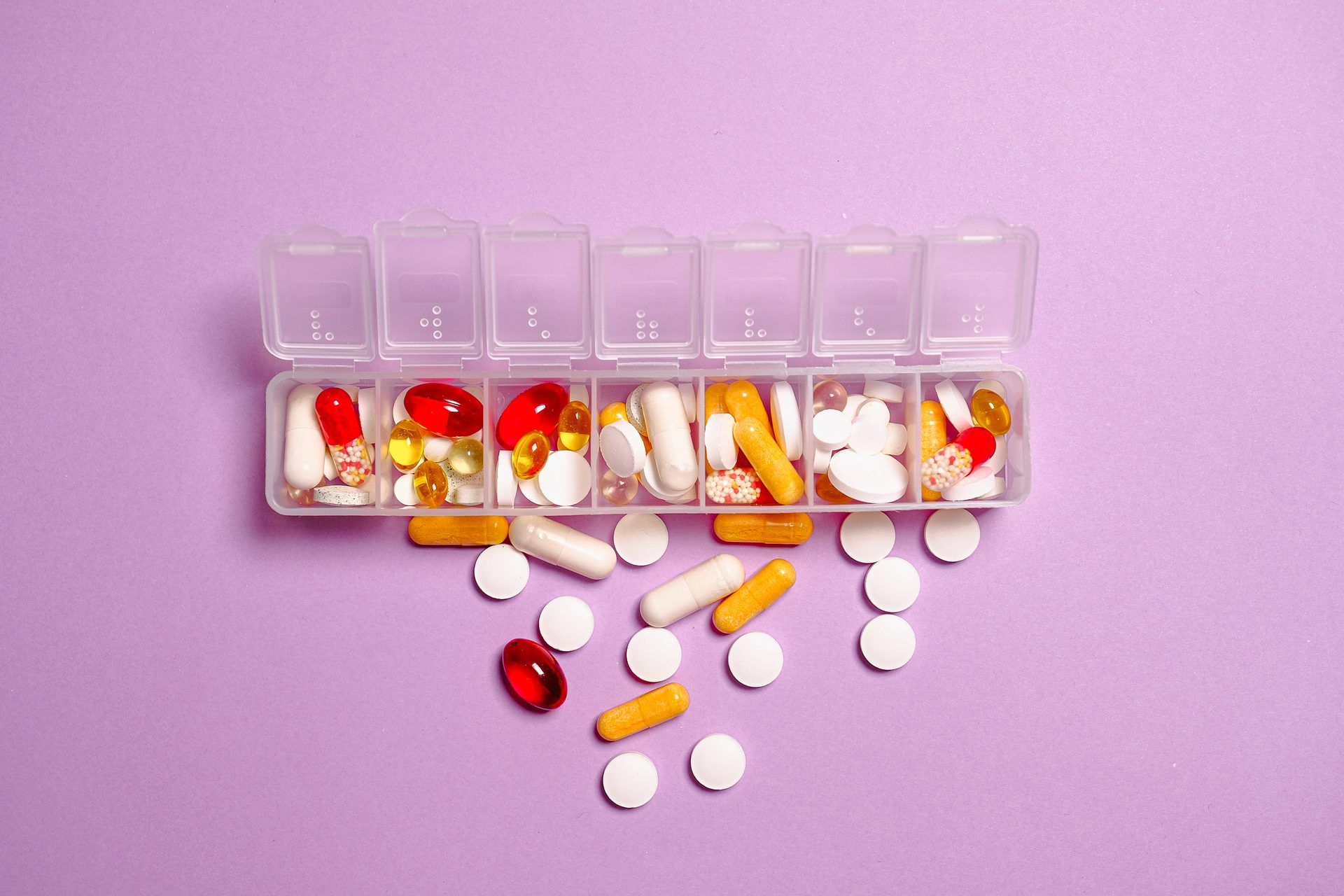
A team of researchers at Stanford University used transfer learning to predict potential drug-target interactions. They pre-trained a model on a large dataset of known interactions and then fine-tuned it on smaller datasets of specific drug targets, achieving improved prediction accuracy.
Molecular docking
Molecular docking is a computational technique used to predict the binding orientation and affinity between a small molecule (ligand) and a protein target. AI-driven molecular docking algorithms improve the efficiency and accuracy of this process, aiding in the identification of potential drug candidates.
AI-driven docking software, like AutoDock and Vina, has been successfully used to identify small molecule inhibitors for various disease targets, including HIV protease, SARS-CoV-2 main protease, and various cancer-related proteins.
Natural Language Processing (NLP)
NLP techniques enable AI models to extract and understand information from textual sources, such as scientific literature and patents. NLP is utilized in drug discovery to gather insights from vast amounts of published research, aiding in target identification, drug repurposing, and knowledge extraction.
AI-powered NLP platforms like IBM Watson for Drug Discovery can be used to analyze vast amounts of biomedical literature. These kinds of efforts help researchers discover new drug targets and repurpose existing drugs for different diseases.
AI-driven drug discovery is a rapidly evolving field, and these key techniques play a crucial role in its advancement. By leveraging the power of generative AI, reinforcement learning, GNNs, transfer learning, molecular docking, and NLP, researchers can efficiently explore the vast chemical space, identify potential drug candidates, and accelerate the drug development process.
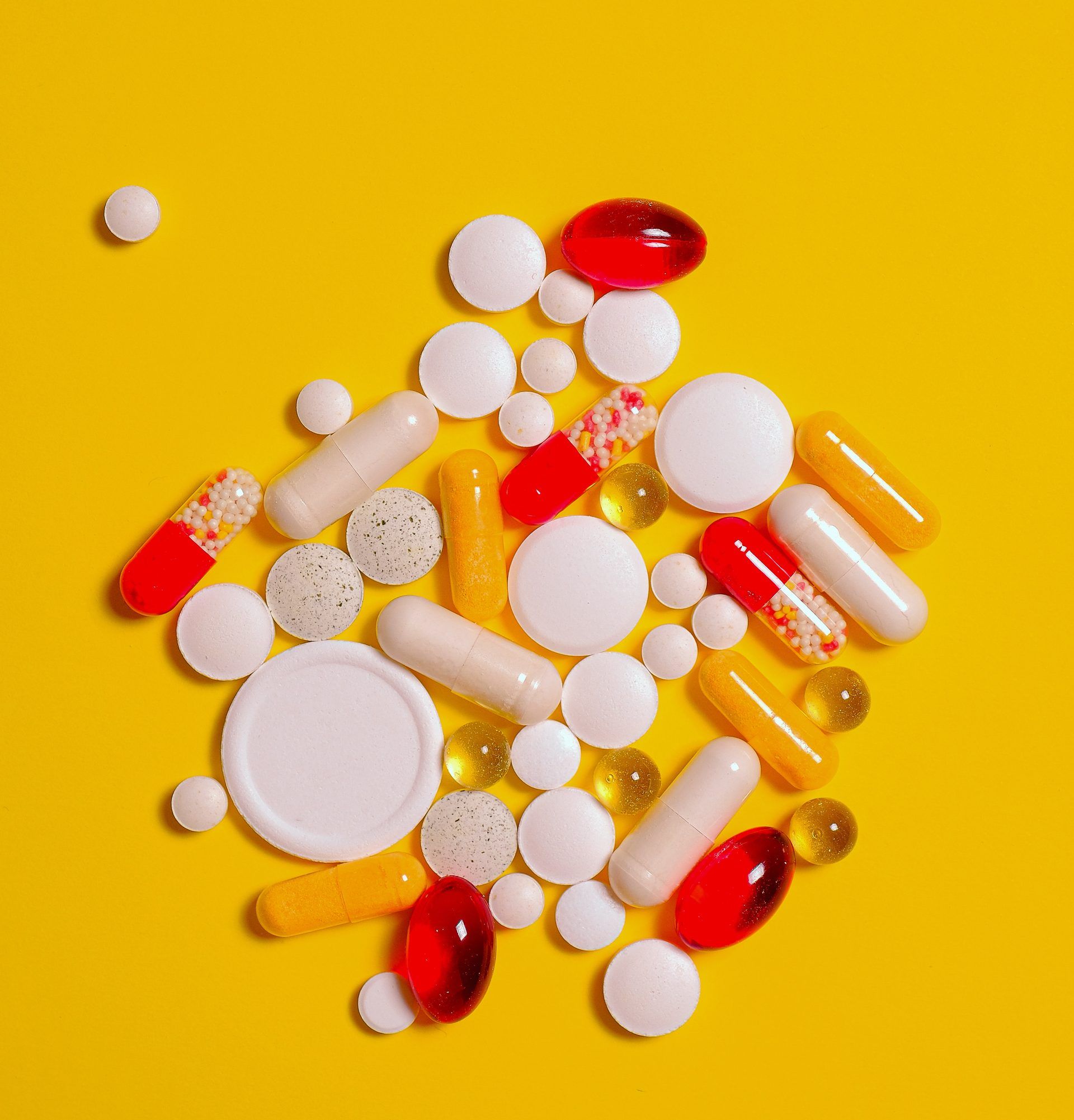
The synergistic combination of these techniques holds the potential to transform the pharmaceutical industry and contribute significantly to improving global healthcare outcomes. And it’s not the only advantage of it.
Machine learning applications in healthcare
Advantages of generative AI in drug discovery
The integration of generative AI in drug discovery offers several significant advantages:
- Accelerated drug discovery process: Generative AI in drug discovery drastically speeds up the process by enabling researchers to explore a vast chemical space in a short amount of time. This efficiency expedites the identification of potential drug candidates, shortening the time between initial discovery and clinical trials.
- Cost savings: Traditional drug discovery can cost billions of dollars due to high failure rates and time-consuming experiments. Generative AI in drug discovery reduces the need for expensive lab work and increases the likelihood of success, leading to substantial cost savings.
- Targeting rare diseases and personalized medicine: For rare diseases with limited data on potential drug targets, generative AI can help by extrapolating knowledge from existing datasets to propose potential therapeutic molecules. Additionally, the technology is well-suited for personalized medicine, tailoring treatments to individual patients based on their genetic makeup.
Disadvantages of generative AI in drug discovery
While generative AI holds immense promise for drug discovery, it also faces certain challenges and limitations:
- Data quality and bias The accuracy and reliability of generative AI models heavily depend on the quality and diversity of the training data. Biased or incomplete datasets can lead to biased AI models, which may result in the generation of suboptimal or unsafe drug candidates.
- Safety and toxicity prediction: Generative AI models often struggle to predict the safety and toxicity of newly generated molecules accurately. This is a critical concern as the ultimate goal is to identify drugs that are not only effective but also safe for human use.
- Intellectual property concerns: The use of generative AI in drug discovery raises intellectual property issues. If AI algorithms generate a new drug candidate, determining patent rights and ownership can become complicated.
- Ethical considerations: The use of AI in drug discovery brings up ethical questions about the responsibility and accountability of the technology. Ensuring transparency and adherence to ethical guidelines is crucial in adopting generative AI responsibly.
Conclusion
Generative AI represents a groundbreaking advancement in drug discovery, revolutionizing the process of identifying potential therapeutic molecules. By leveraging the power of machine learning algorithms, generative AI in drug discovery accelerates drug development, reduces costs, and has the potential to improve patient outcomes.
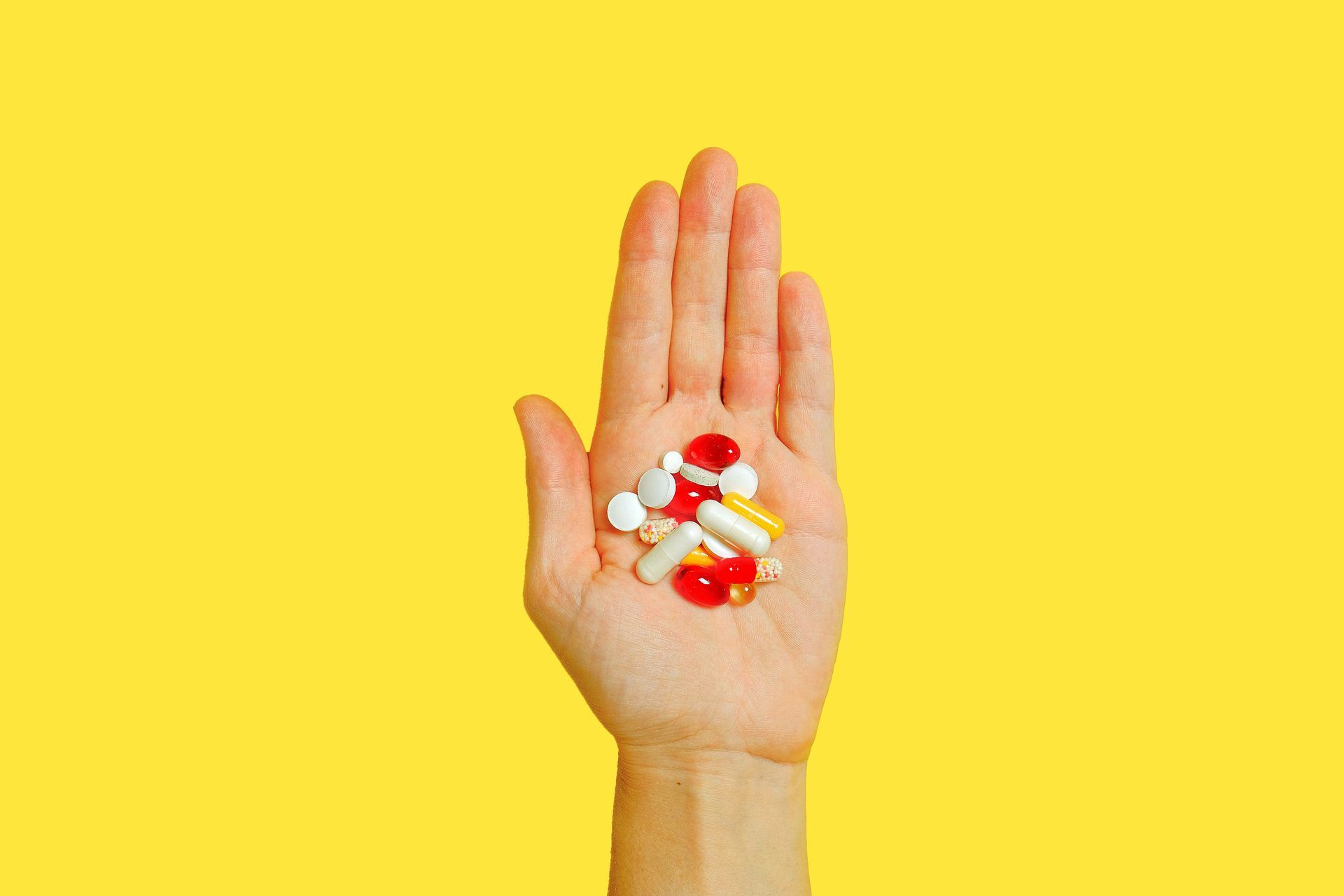
While there are challenges to be addressed, the role of generative AI in drug discovery is a promising and exciting development that holds the potential to transform the pharmaceutical industry and enhance global healthcare.
As research and technology continue to progress, we can expect generative AI to play an increasingly significant role in shaping the future of medicine.
Oh, are you new to AI, and everything seems too complicated? Keep reading…
AI 101
You can still get on the AI train! We have created a detailed AI glossary for the most commonly used artificial intelligence terms and explain the basics of artificial intelligence as well as the risks and benefits of AI. Feel free the use them. Learning how to use AI is a game changer! AI models will change the world.
In the next part, you can find the best AI tools to use to create AI-generated content and more.
AI tools we have reviewed
Almost every day, a new tool, model, or feature pops up and changes our lives, and we have already reviewed some of the best ones:
- Text-to-text AI tools
- Google Bard AI
- Chinchilla
- Notion AI
- Chai
- NovelAI
- Caktus AI
- AI Dungeon
- ChatGPT
- Snapchat My AI
- DuckAssist
- GrammarlyGO
- Jenni AI
- Microsoft 365 Copilot
- Tongyi Qianwen
- AutoGPT
- Janitor AI
- Character AI
- WordAi
- Venus Chub AI
- Crushon AI
- FreedomGPT
- Charstar AI
- Jasper AI
- WormGPT
- How to use WormGPT AI
- WormGPT download, here are the dangers waiting for you
- Llama 2
- Kajiwoto AI
- Harpy AI Chat
See this before login ChatGPT; you will need it. Do you want to learn how to use ChatGPT effectively? We have some tips and tricks for you without switching to ChatGPT Plus, like how to upload PDF to ChatGPT! However, When you want to use the AI tool, you can get errors like “ChatGPT is at capacity right now” and “too many requests in 1-hour try again later”. Yes, they are really annoying errors, but don’t worry; we know how to fix them. Is ChatGPT plagiarism free? It is a hard question to find a single answer. Is ChatGPT Plus worth it? Keep reading and find out!
- Text-to-image AI tools
- MyHeritage AI Time Machine
- Reface app
- Dawn AI
- Lensa AI
- Meitu AI Art
- Stable Diffusion
- DALL-E 2
- Google Muse AI
- Artbreeder AI
- Midjourney
- How to fix Midjourney invalid link
- Midjourney alternatives
- Midjourney AI tips
- Midjourney V5.2
- Midjourney video generation guide
- Where to look for the best Midjourney images?
- DreamBooth AI
- Wombo Dream
- NightCafe AI
- QQ Different Dimension Me
- Random face generators
- Visual ChatGPT
- Adobe Firefly AI
- Leonardo AI
- Hotpot AI
- DragGAN AI photo editor
- Freepik AI
- 3DFY.ai
- Photoleap
- Artguru
- Luma AI
- BlueWillow AI
- Scribble Diffusion
- Clipdrop AI
- Stable Doodle
While there are still some debates about artificial intelligence-generated images, people are still looking for the best AI art generators. Will AI replace designers? Keep reading and find out.
- AI video tools
- AI presentation tools
- AI search engines
- AI interior design tools
- Other AI tools
Do you want to explore more tools? Check out the bests of:
Feature image credit: Pexels