Data analytics and artificial intelligence are changing how businesses decide their paths today. All we hear about is data these days. We hear about how much is produced, how crucial it is, and how corporations use it to further their objectives and boost profits. Yet how? Yes, much of it is technologically based, but how will businesses keep up with the enormous amount of data generated daily? The answer is data science and artificial intelligence.
To extract hidden patterns from unstructured data, data scientists utilize a variety of instruments, algorithms, formulae, and machine learning techniques. These patterns can then be utilized to inform decision-making and improve understanding of various aspects. Data science reveals the “why” underlying your data, going beyond simple number crunching.
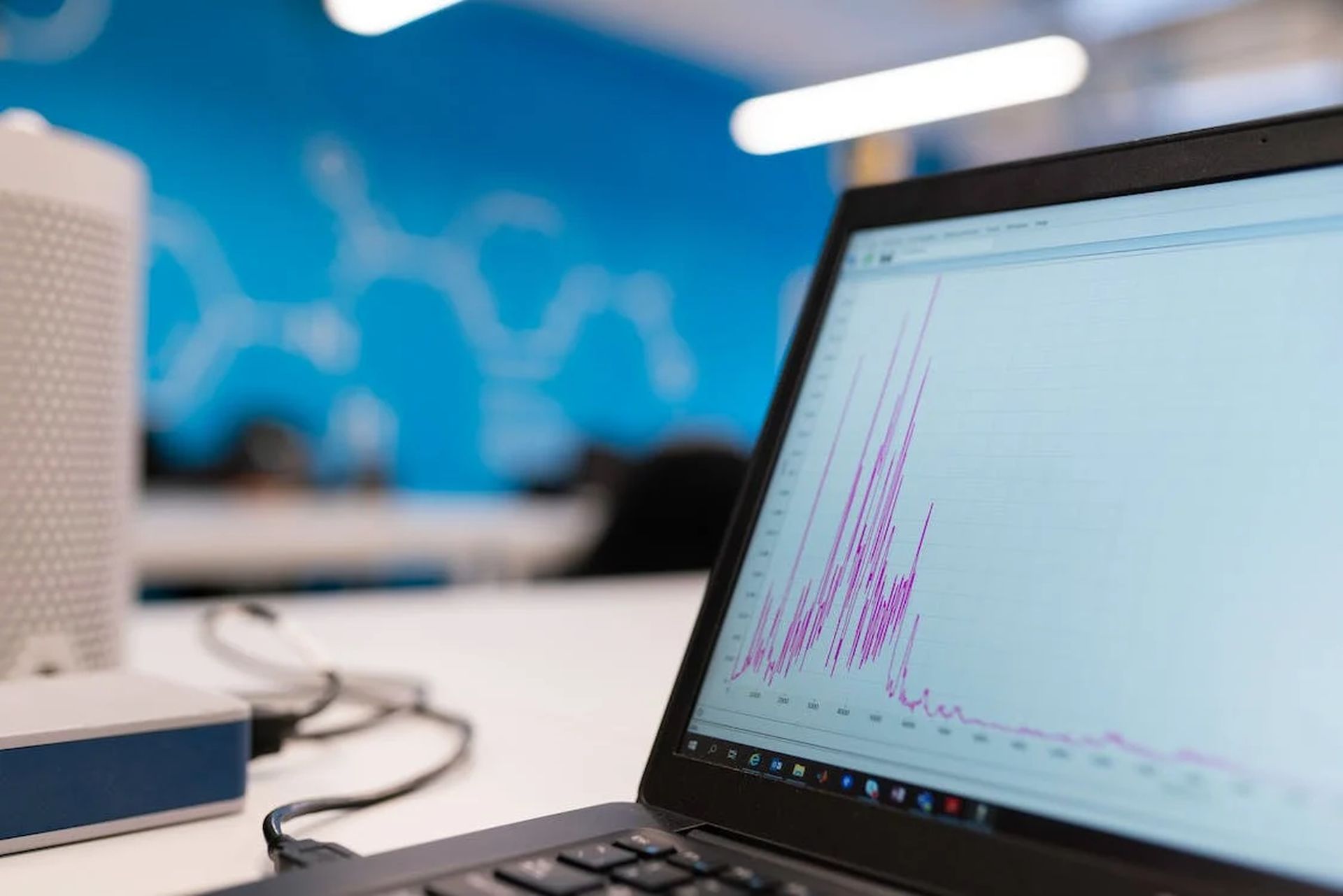
Data science is the secret to turning information into useful information by analyzing vast amounts of facts to predict actions and derive meaning from meaningfully connecting data. Data science assists businesses in maximizing innovation by assisting them in locating the best clients, setting the appropriate rates, effectively allocating expenditures, and decreasing work-in-progress and inventory.
A helping hand: Enterprise SEO tools are crucial for data analytics
Although data science technology and techniques have advanced significantly, no advancement was more significant than the advent of artificial intelligence (AI). AI is the capacity of computers to carry out tasks that were previously only performed by humans. AI previously required just human programming, but computers can now learn from data and hone their skills with machine learning. As a result, AI tools are now able to read, write, listen, chat, and even listen like a human – but at a scale and rate that is significantly greater than those of any one person. That is why data analytics and artificial intelligence are both crucial for the future of technology.
Data analytics and artificial intelligence definition
Let’s examine each term’s definition, beginning with a data analytics definition. Fundamentally, data analytics is the science of examining large data sets to identify patterns, provide answers to inquiries, and draw inferences. It’s an intricate and diverse field that frequently makes use of specialized software, automated processes, and algorithms.
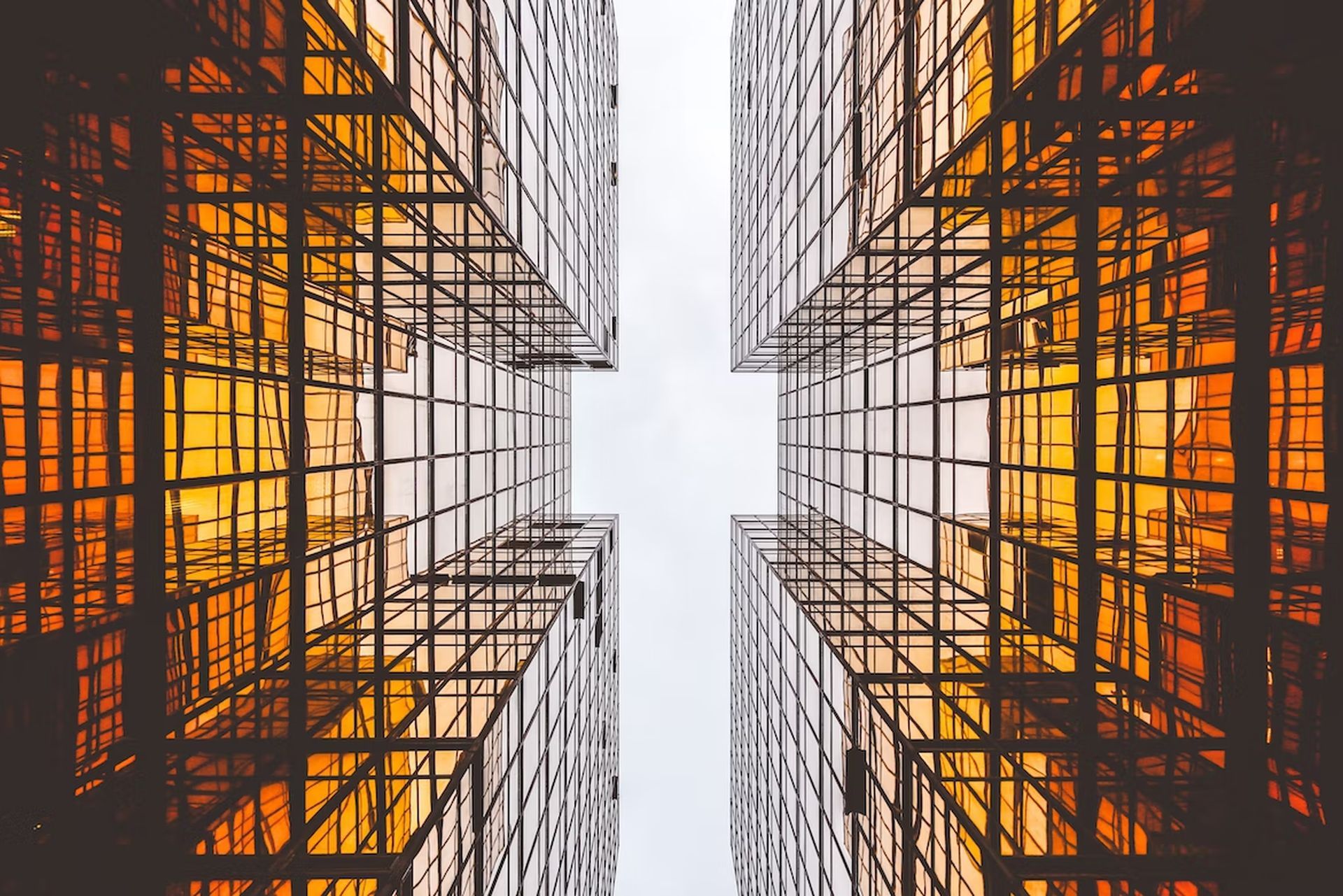
Nearly every industry can benefit from using data analytics and artificial intelligence in some way. Organizations of all types employ data analysts to assist them in making defensible judgments regarding various aspects of their company. Usually, historical data from events are analyzed, allowing for the identification of current trends.
Descriptive analytics, diagnostic analytics, predictive analytics, and prescriptive analytics are just a few of the several forms of data analytics.
The idea of artificial intelligence (AI) has been around for a long time. But it wasn’t until recently that we actually had the computing capacity to make it a reality. The capacity to enable computers to mimic human intelligence is the essence of artificial intelligence.
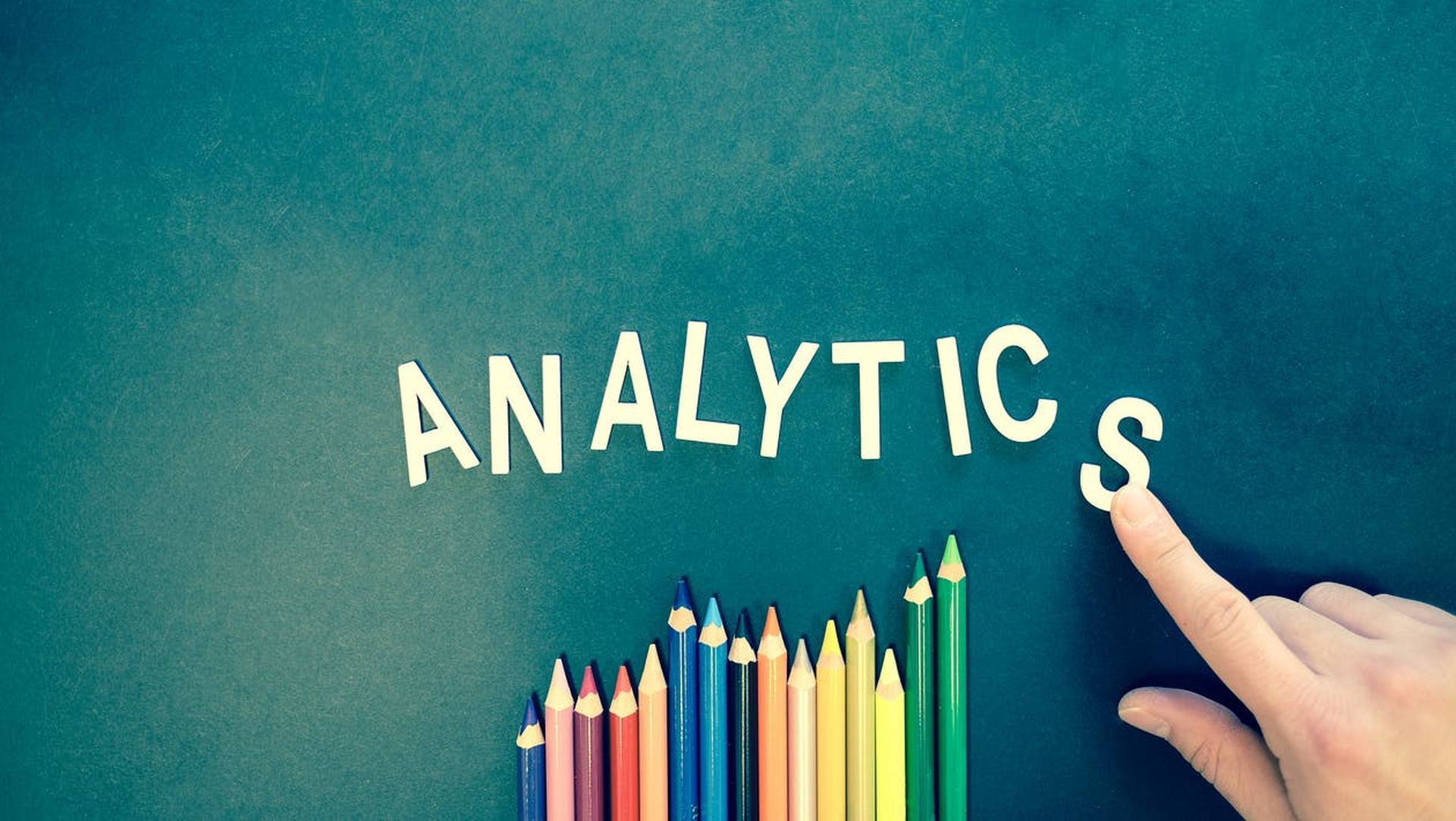
It is possible to teach computers through experience by building machines capable of learning. These artificial intelligence systems have three characteristics: intelligence, adaptability, and intentionality. These characteristics enable them to make decisions that ordinarily require a human degree of knowledge and experience.
Where do data analytics and artificial intelligence overlap?
So, as we’ve said, we have three different areas of competence. They are all quite separate fields with unique applications, sets, and specializations. There are undoubtedly certain locations where they overlap, as you may have already seen.
What is the future of data analytics?
In the future, data analytics and artificial intelligence are anticipated to alter how we live and conduct business. We already make a lot of decisions in our daily lives using the analytics built into our technology. We use these tools to find waste in corporate processes and learn how to drive from point A to point B while avoiding traffic jams.
Machine learning makes life easier for data scientists
It is obvious that while organizations are making efforts to turn data into insights, they are still having trouble with data quality and locating the resources necessary to convert these insights into real value and move toward a more data-driven approach.
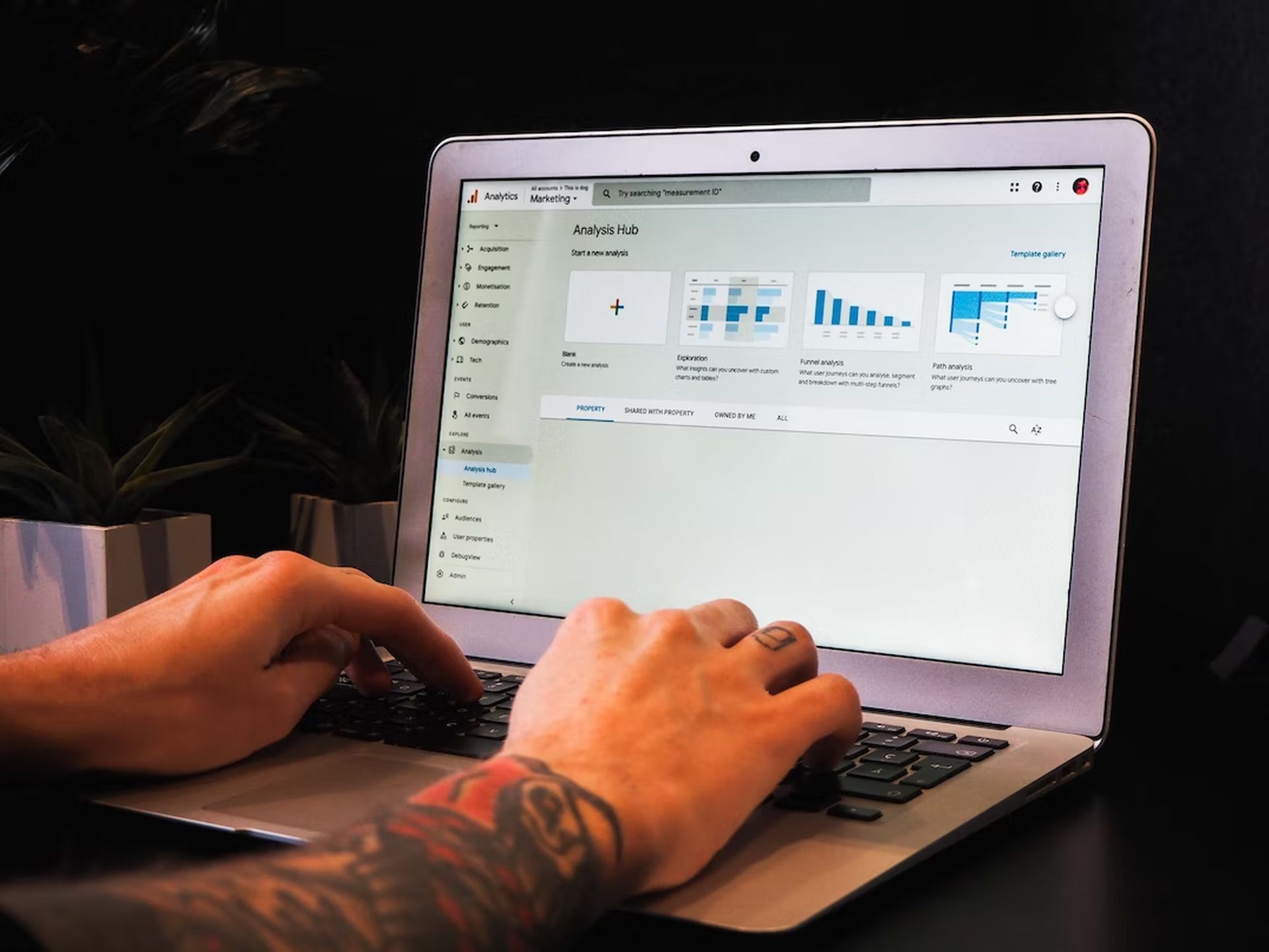
Although the data era is still in its infancy, expectations are that data analytics will enable the impossible. In essence, all businesses are increasingly making investments in data analytics capabilities to stay abreast of both known and unknowing trends and competition.
The decision-making process was initially “assisted” by data analytics, but it is now enabling “better” decisions than we can make on our own. Here, examples of using analytics to merge several data sources that yield new and better insights come to mind. For instance, you could combine sales, location, and weather data to determine why sales are increasing in certain stores and enhance the restocking process.
How is data analytics used in AI?
Of course, there are a lot of fields that have direct connections to data analytics and artificial intelligence. There are common approaches and technologies used in subjects as disparate as statistics, mathematics, computer science, and information science. Other related areas of specialization include some of the following:
- Robot science: The pinnacle of artificial intelligence is thought to be the creation and programming of robots that can function in actual environments. Here, machine learning is especially crucial since it enables computers to respond appropriately to visual and aural signals.
- Cloud services: Artificial intelligence and machine learning often demand a significant amount of processing power. That power may come from cloud computing, which is the technique of providing on-demand computing services through the internet.
- Big data analytics: The idea of big data is fundamental to many of these professions. Large datasets of organized and unstructured data that are challenging to process through conventional methods are referred to by this term.
- Data mining and statistics: Massive and intricate data sets are used in data mining. Some machine learning principles are applied to analyze this data and draw inferences and predictions from it.
Automating data analysis using artificial intelligence
With the aid of automation, the most recent developments in data analytics and artificial intelligence significantly contribute to increasing the effectiveness and power of commercial processes. Because of AI, analytics is also becoming more automated and accessible. Here are a few examples of how AI is advancing analytics:
- AI systems may automatically evaluate data and find hidden trends, patterns, and insights that can be used by staff to make more educated decisions. This is done with the aid of machine learning algorithms.
- By employing natural language generation, AI automates report creation and simplifies data.
- AI improves data literacy and frees up time for data scientists by enabling everyone in the business to intuitively find solutions and extract insights from data using natural language query (NLQ).
- By automating data analytics and generating insights and value more quickly, AI aids in the streamlining of BI.
Instead of using rule-based programs like traditional business intelligence (BI) did to generate static analytics reports from data, enhanced analytics makes use of AI tools like machine learning and natural language generation.
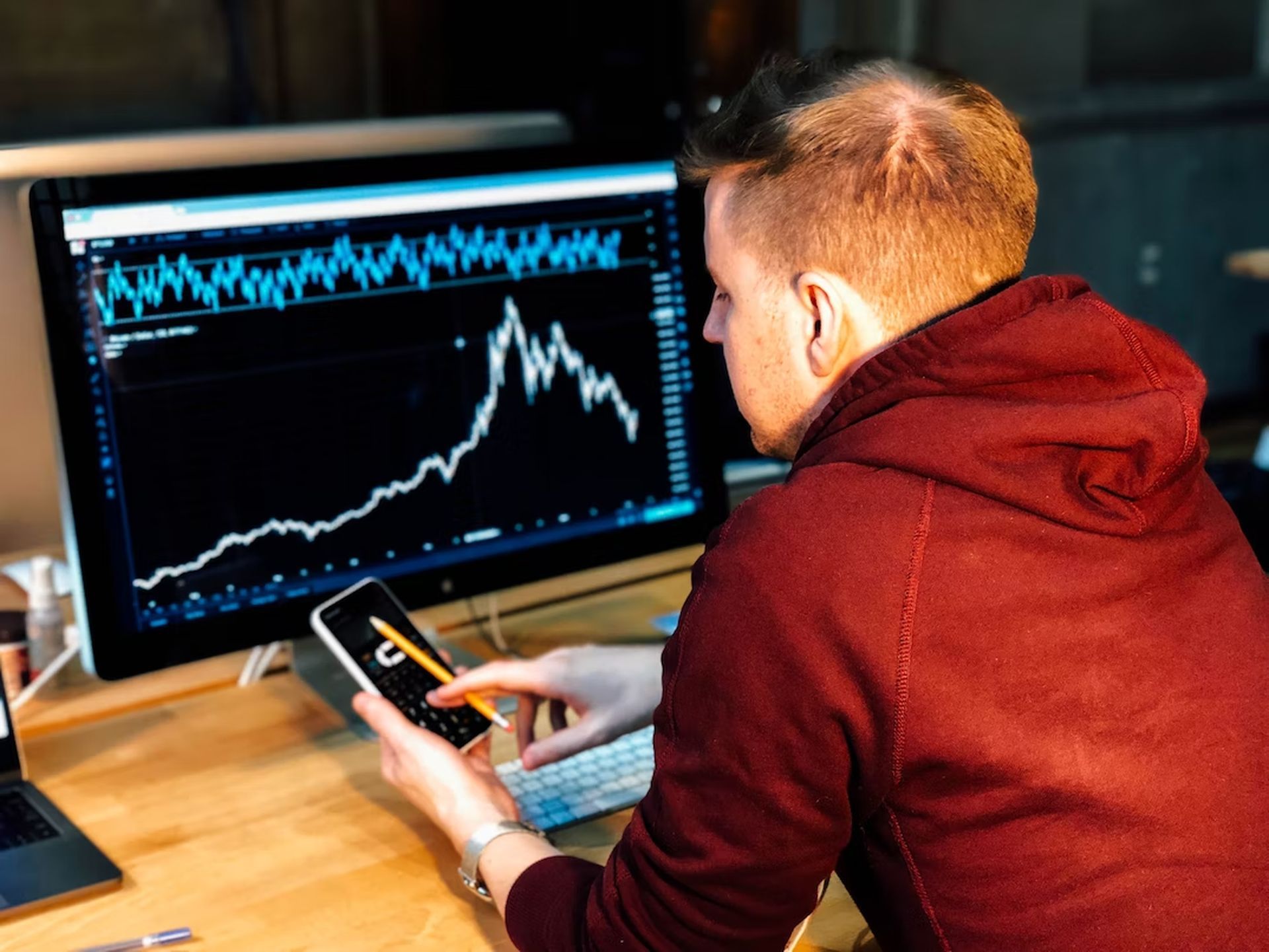
Machine learning extracts patterns, trends, and connections between data points by learning from the data itself. To adjust to changes and make adjustments based on the data, it might draw from past examples and experiences.
Using language, natural language generation transforms the results of machine learning data into understandable insights. All the insights are derived by machine learning, and NLG transforms them into a format that is legible by humans.”
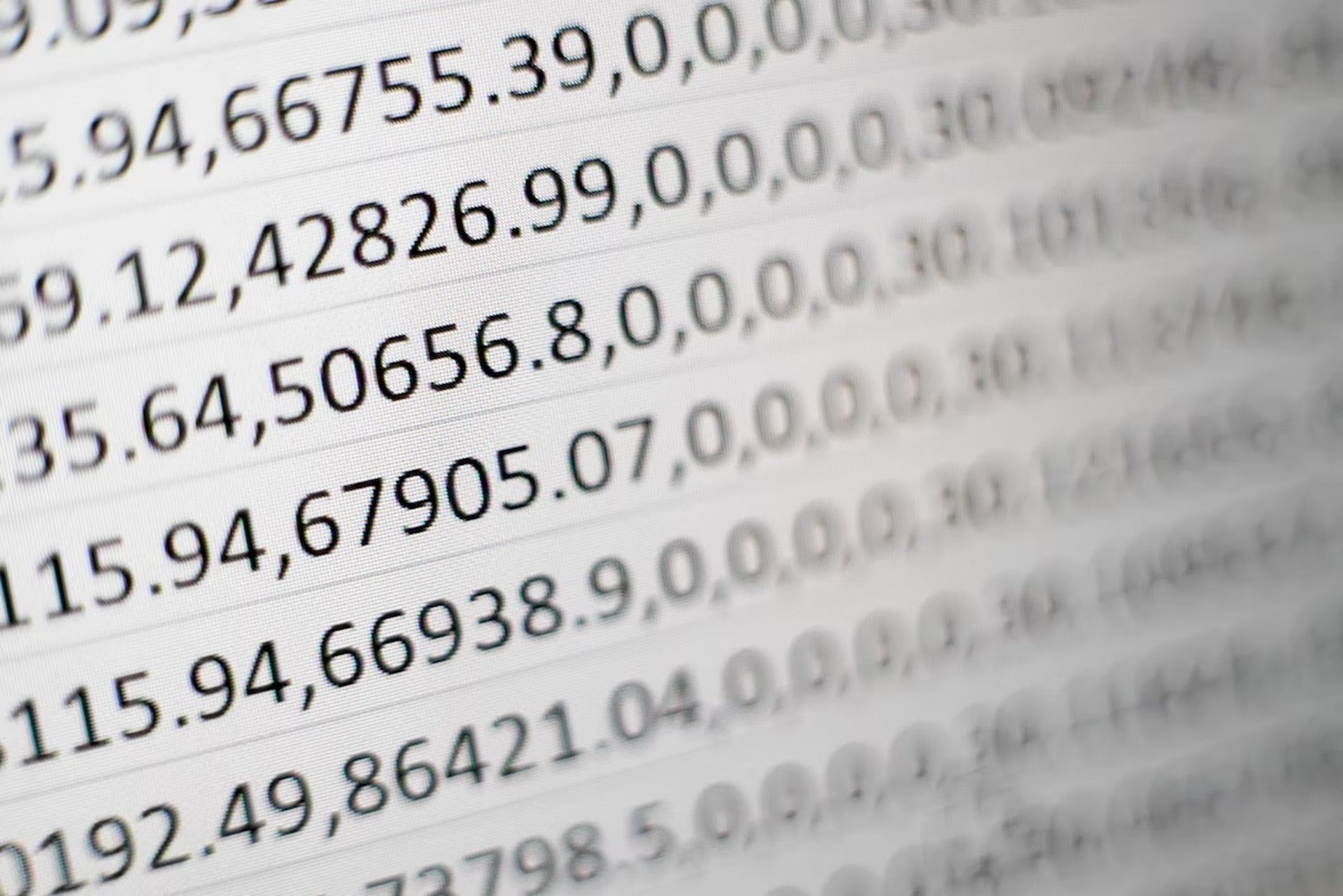
Users can ask questions and receive replies from augmented analytics in the form of text and images. Non-technical individuals may easily evaluate data and find insights because of the automated nature of the entire process of generating insights from data.
What is the role of machine learning in data analytics?
In essence, machine learning automates the process of data analysis and makes real-time predictions based on data without the need for human interaction. A data model is automatically created and then trained to provide predictions in real-time. The data science lifecycle is when machine learning algorithms are applied.
The standard machine learning process begins with you providing the data to be studied, followed by you defining the precise features of your model and the construction of a data model in accordance with those features. The original training dataset is then used to train the data model. The machine learning algorithm is prepared to make a prediction the next time you upload a fresh dataset once the model has been trained.
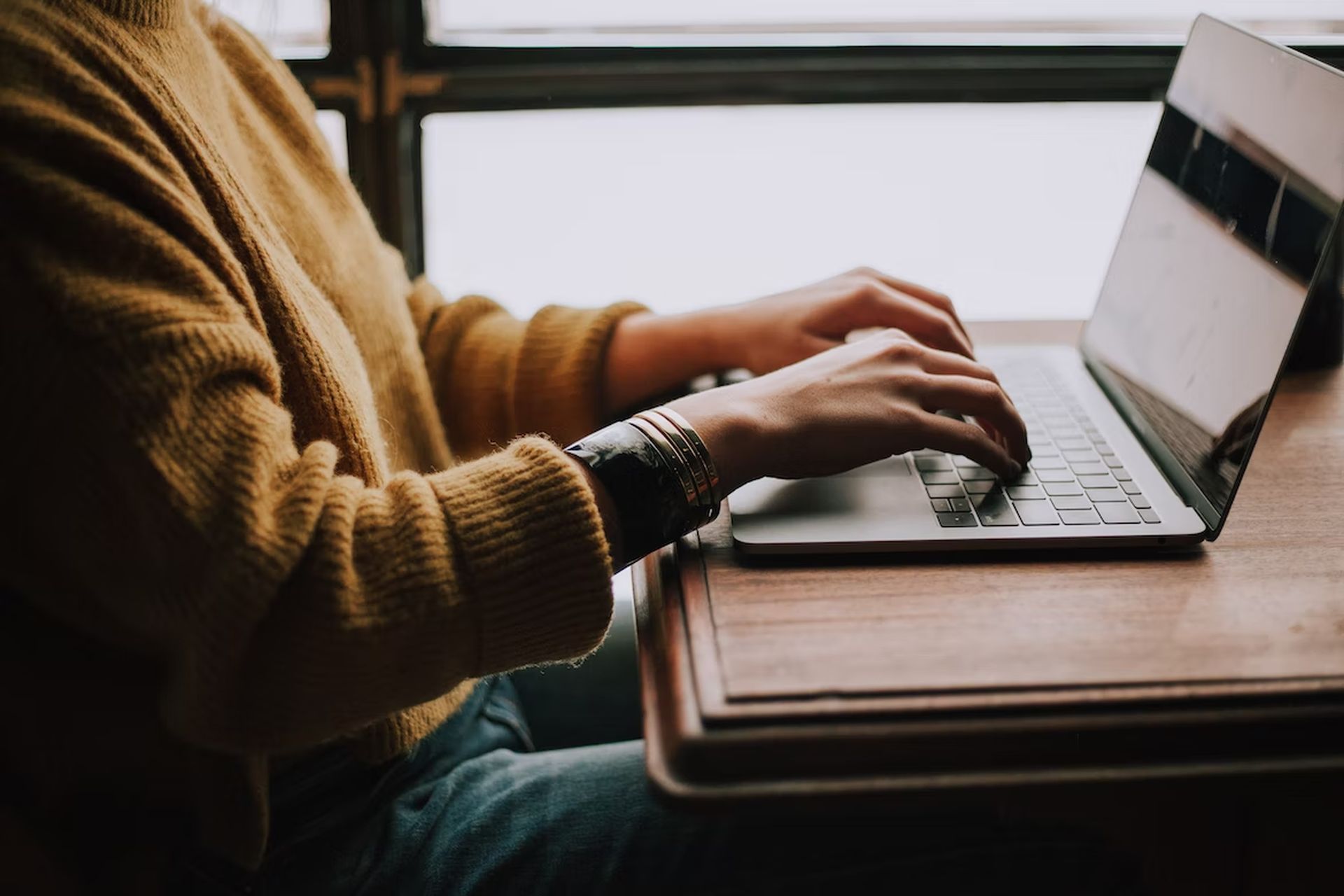
What is the difference between data analytics and artificial intelligence?
While AI involves data analysis, making assumptions, and seeking to make predictions that are beyond the capacity of humans, data analytics works by detecting patterns based on historical data to anticipate future events.
Finding patterns in the data is the goal of data analytics, but artificial intelligence (AI) tries to automate the process by giving robots human intelligence.
Will artificial intelligence replace data analytics?
Data analytics and artificial intelligence are co-living creatures. The requirement to handle and evaluate data as soon as someone in an organization comes into contact with it is great because today’s world is data-driven, and technology is evolving quickly. In order to deploy the abundance of information while still keeping it all secure and well-guarded, new tools and procedures must be deployed. In this current technology era, artificial intelligence and data science are the two most significant data managers.
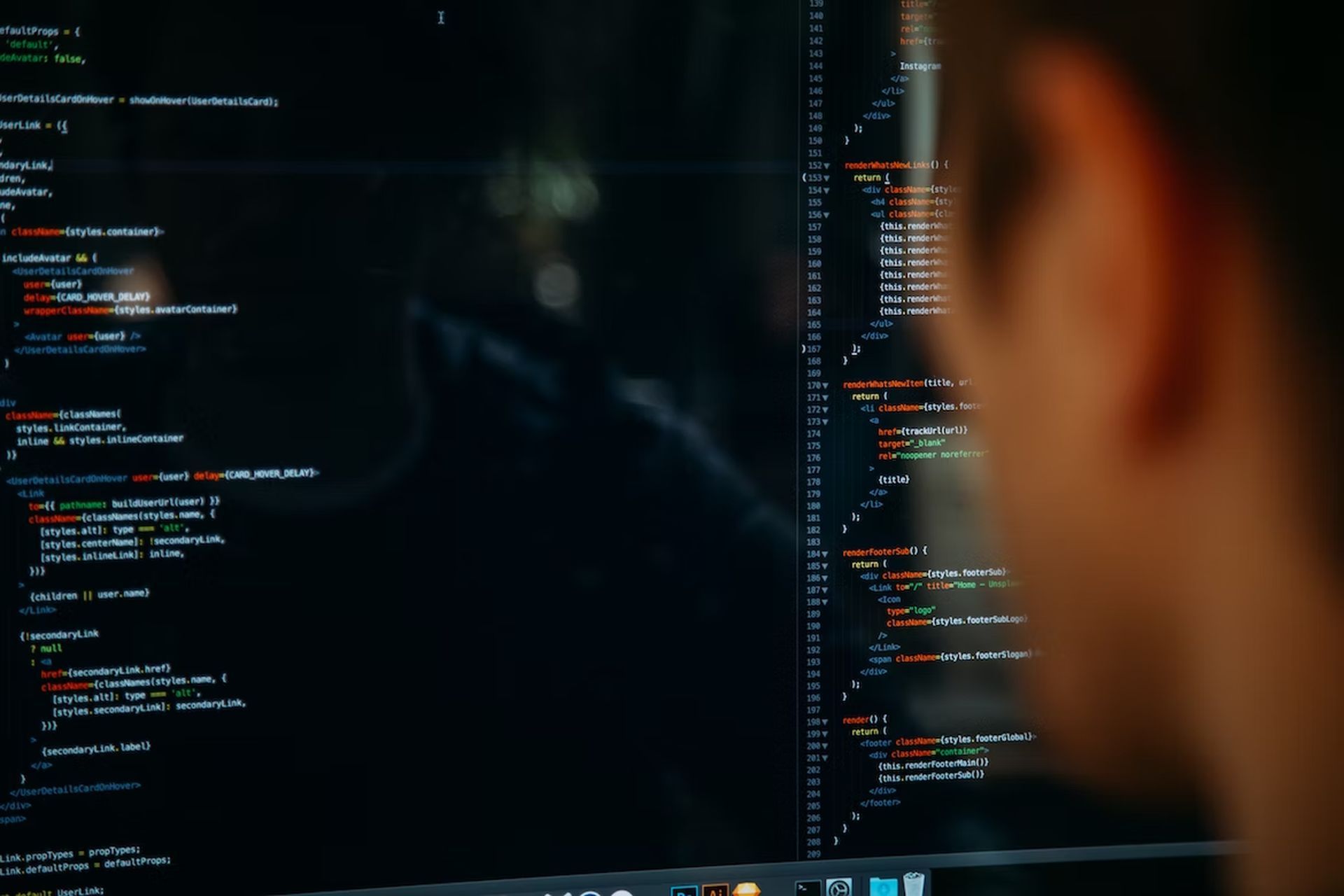
While most machines are replacing people, it is a known fact that artificial intelligence will never be able to replace data analysis. Instead, they complement one another to increase each other’s efficiency. This is true for a number of noteworthy reasons.
Every server may be connected by machines, increasing efficiency, but if one server is connected to the wrong network, everything could be irreparably damaged. This is mostly handled by data analytics, which has extensive knowledge of all networks and servers and carefully examines each system before making judgments on equipment.
New artificial intelligence can diagnose a patient using their speech
No matter how many computers enter the tech hub, they will never be able to replace the importance of expert data analytics because these tools offer critical evaluation and suggestions for improving outcomes in their respective fields. Yes, machines supply the relevant knowledge, and necessary facts needed to make informed decisions. Although data analytics has the knowledge to use these techniques, artificial intelligence has the right instruments.
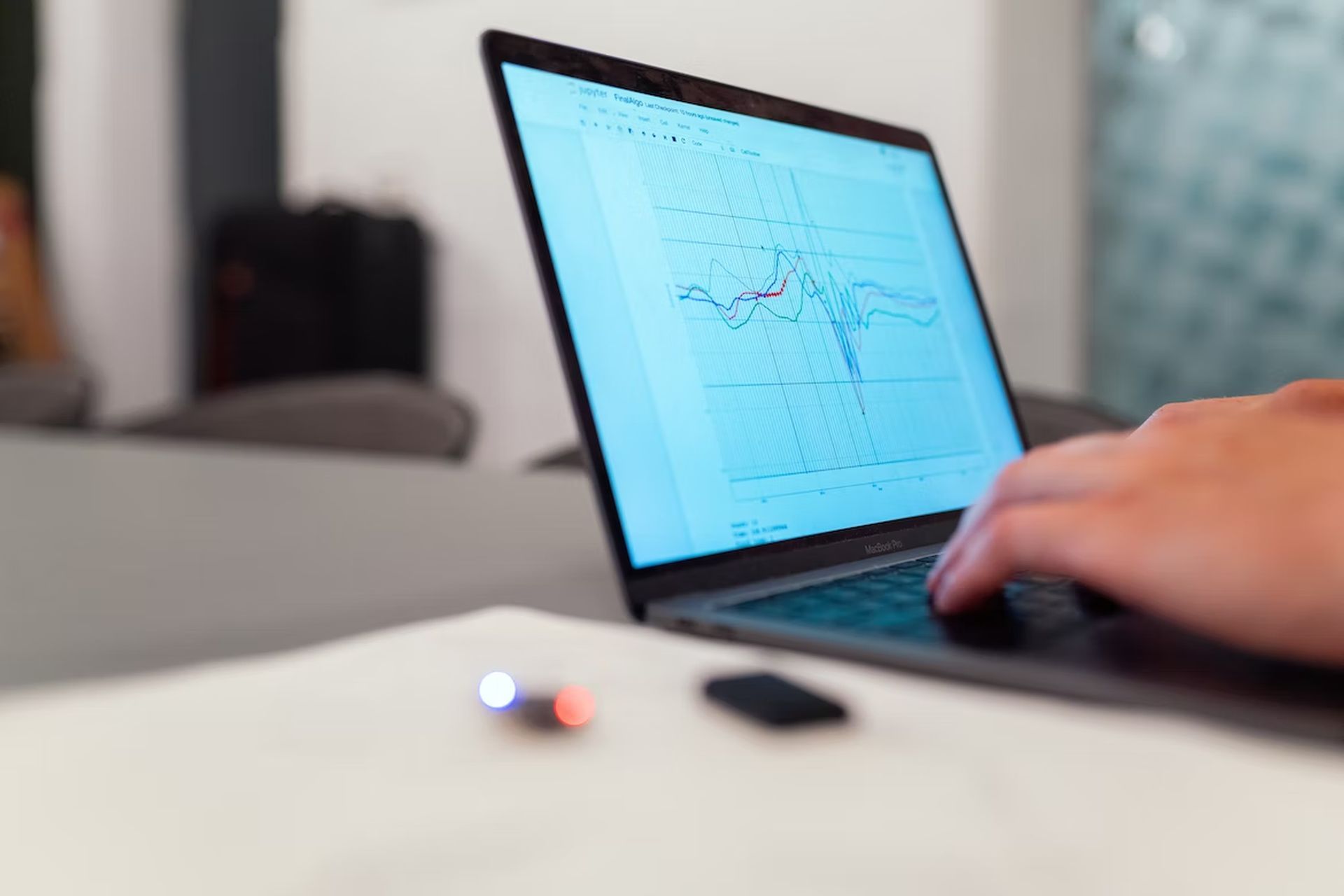
Although artificial intelligence is gaining popularity, this does not mean that it will completely dominate the data analytics industry. Instead, all the tasks in the systems are performed by data science and artificial intelligence using far more virtuosic and sophisticated methods. Data analytics is used to create conclusions utilizing logical reasoning and expository thinking, while artificial intelligence is used to interpret stored data.
Data analytics are ideal for controlling artificial intelligence, which results in the longer continuation of the business. Machines will always need a command to function properly in all areas. But only a qualified data analyst with the necessary certificates can operate this equipment and carry out essential tasks for the company.
Who earns more, data scientist or artificial intelligence expert?
A data scientist can expect to make about $116,654 a year on average, according to Indeed. Big data is powerful, and the companies paying these hefty salaries are keen to use it to improve business decisions. Even entry-level pay is beginning to look appealing in this expanding sector. Entry-level data scientists can make up to $93,167 per year, while seasoned data scientists can make up to $142,131 annually.
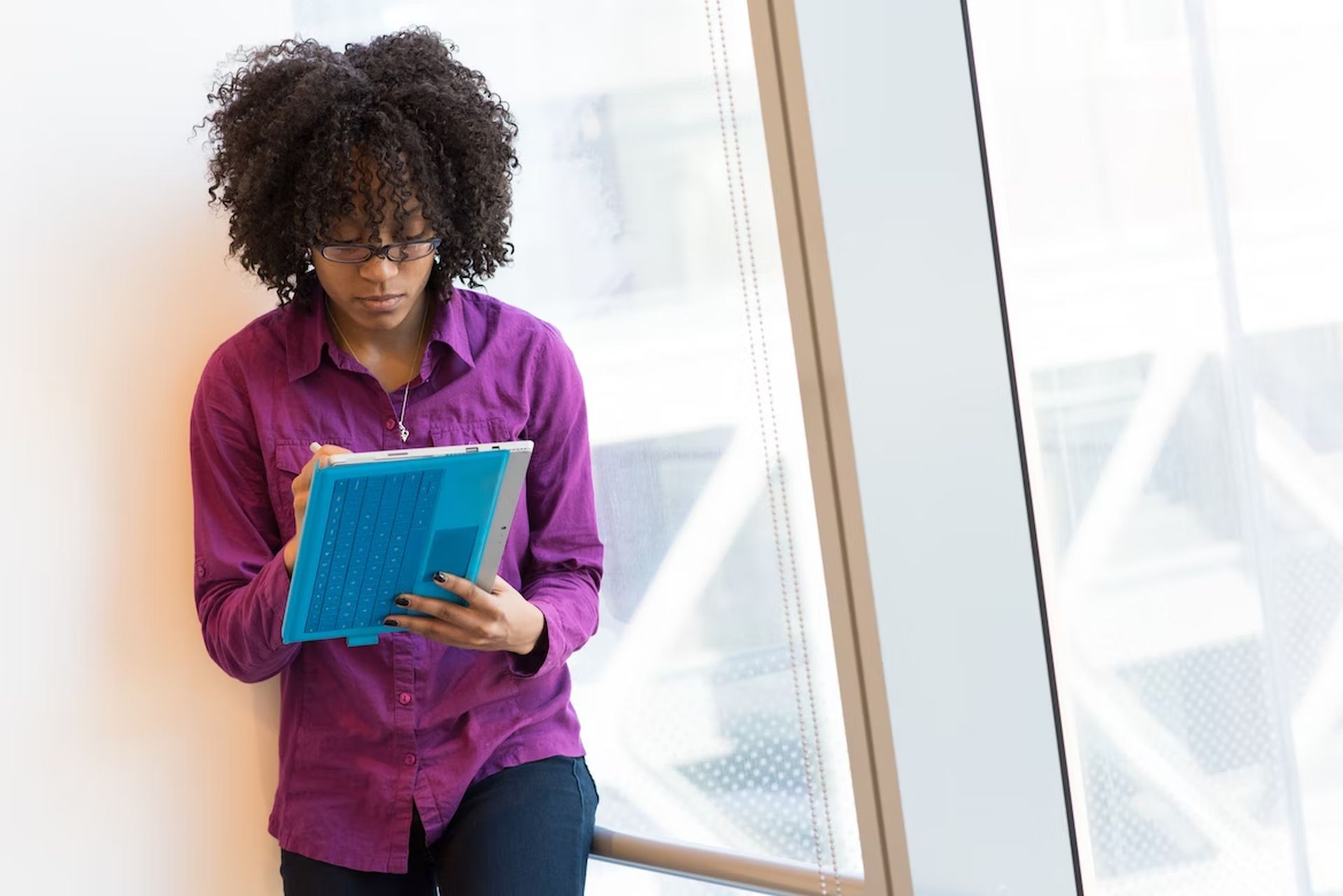
Similar to that, an artificial intelligence engineer makes well over $100,000 per year on average. With an average low of $90,000 and a high of $304,500, the average national wage in the United States is $164,769 each year. The salary of AI engineers will rise as the number of job options for them dramatically increases.
What is an AI analyst?
An AI data analyst is a computer expert tasked with gathering, processing, and locating statistical data from the datasets that already exist. Due to its promising future, the career path of an AI data analyst is in high demand. You can investigate careers in AI & ML, data science, and related areas after you are on the learning path for AI data analysts.
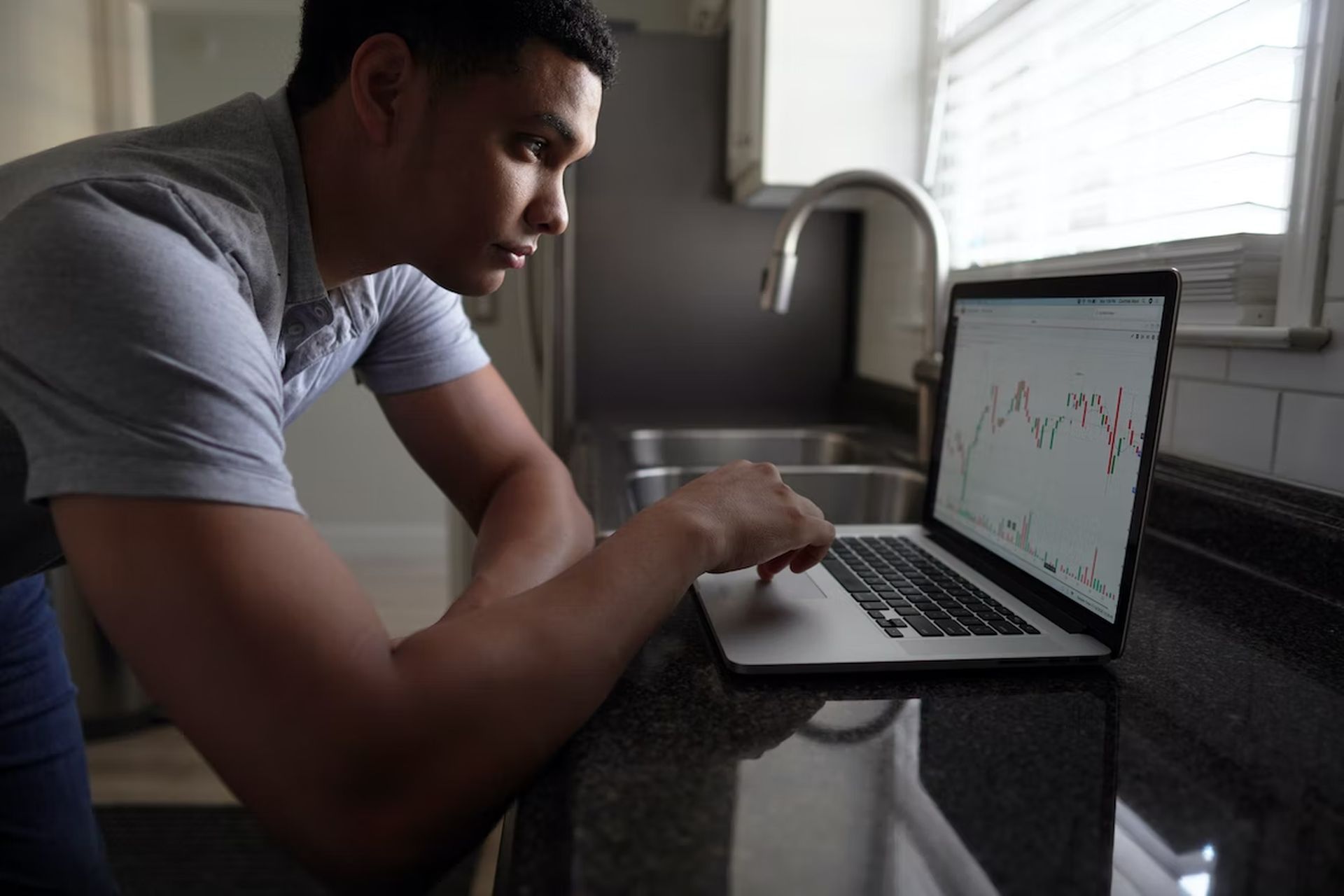
AI data analyst salary
On average, an artificial intelligence analyst makes $105,456 a year in the US\ according to ZipRecruiter.
Suppose you need a quick pay estimator. That comes out to be about $50.70 per hour. This is the same as $2,028 every week or $8,788 per month.
Data analytics and artificial intelligence jobs
If these data-driven fields of interest intrigue you, you may be thinking about a job in a related field. But what jobs are available in the various fields? We’ve only chosen a handful of instances of each.
Data analytics jobs
- Data analyst: The primary responsibility of a data analyst is to transform raw data into insightful knowledge. They strive to recognize trends and convey them in a useful and clear manner.
- BI analyst: Business intelligence analysts strive to offer data insights that can help with decision-making in the workplace. They employ a range of methodologies and tools to empower organizations to make data-driven decisions.
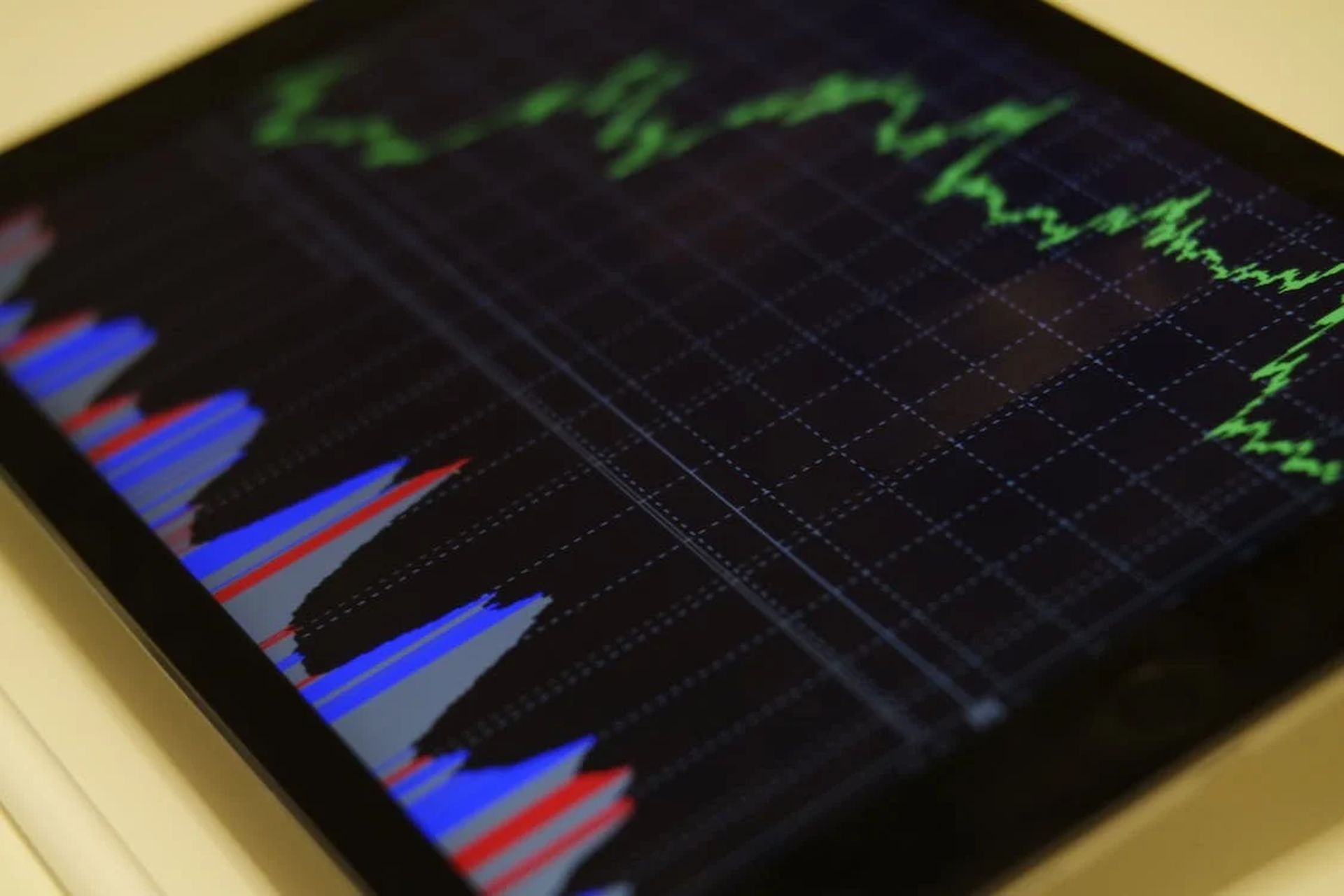
Artificial intelligence jobs
- Robotics engineer: This position focuses on creating and designing machines that can perform work for us. AI is necessary for robotics when building robots that can carry out difficult jobs. It is a very important job for data analytics and artificial intelligence.
- AI programmer: An artificial intelligence programmer works to develop software that’s used for AI applications. It’s a role very much focused on the software development perspective.
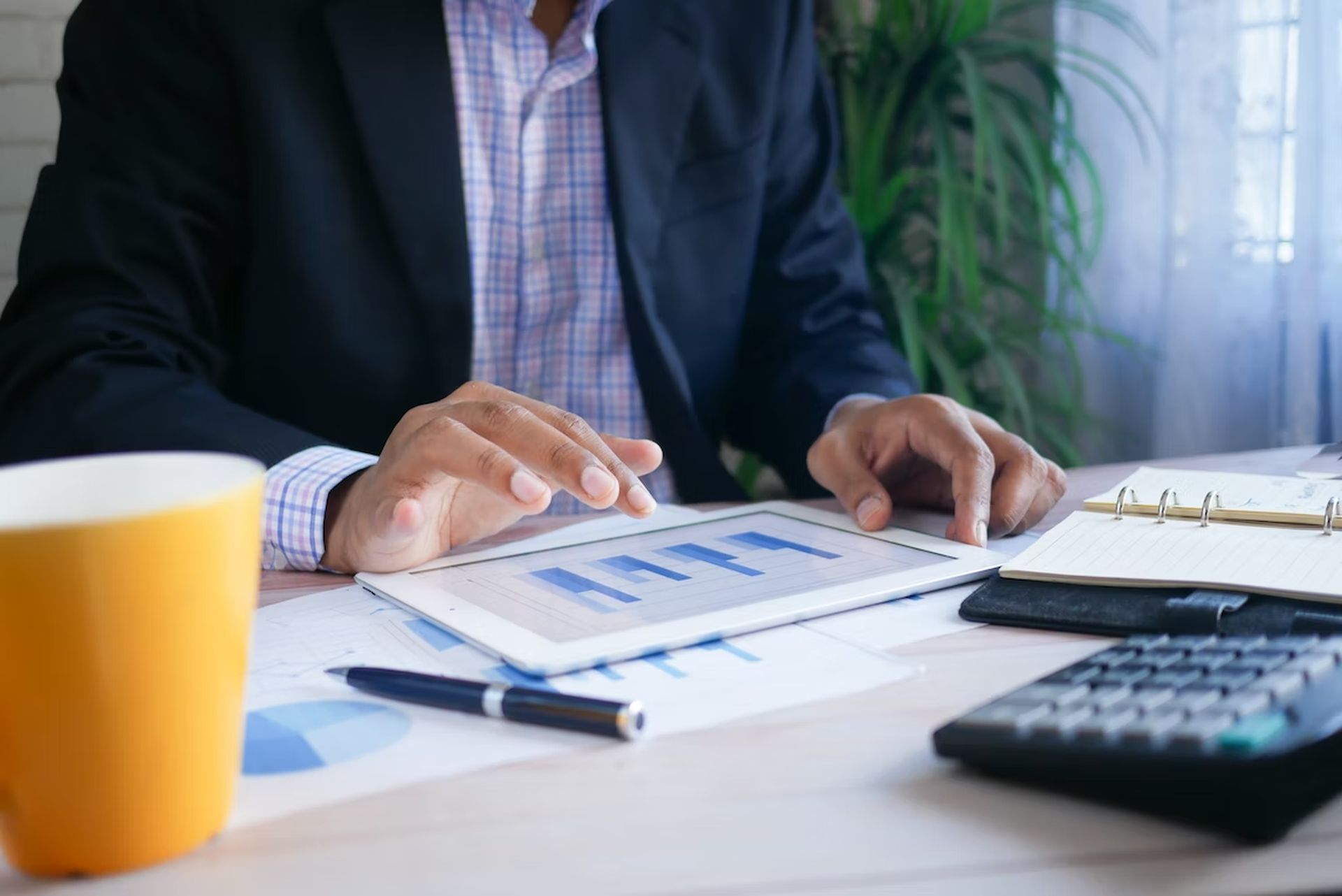
Machine learning jobs
- ML engineer: In this position, there are overlaps between software engineering and data science. Computer programs and algorithms are developed by machine learning engineers to aid in the autonomous learning of computers.
- NLP scientist: The technology known as natural language processing (NLP) enables computers to comprehend ordinary spoken language. In order to aid in the process of comprehending human language, NLP researchers develop algorithms. It is a vital profession for data analytics and artificial intelligence.
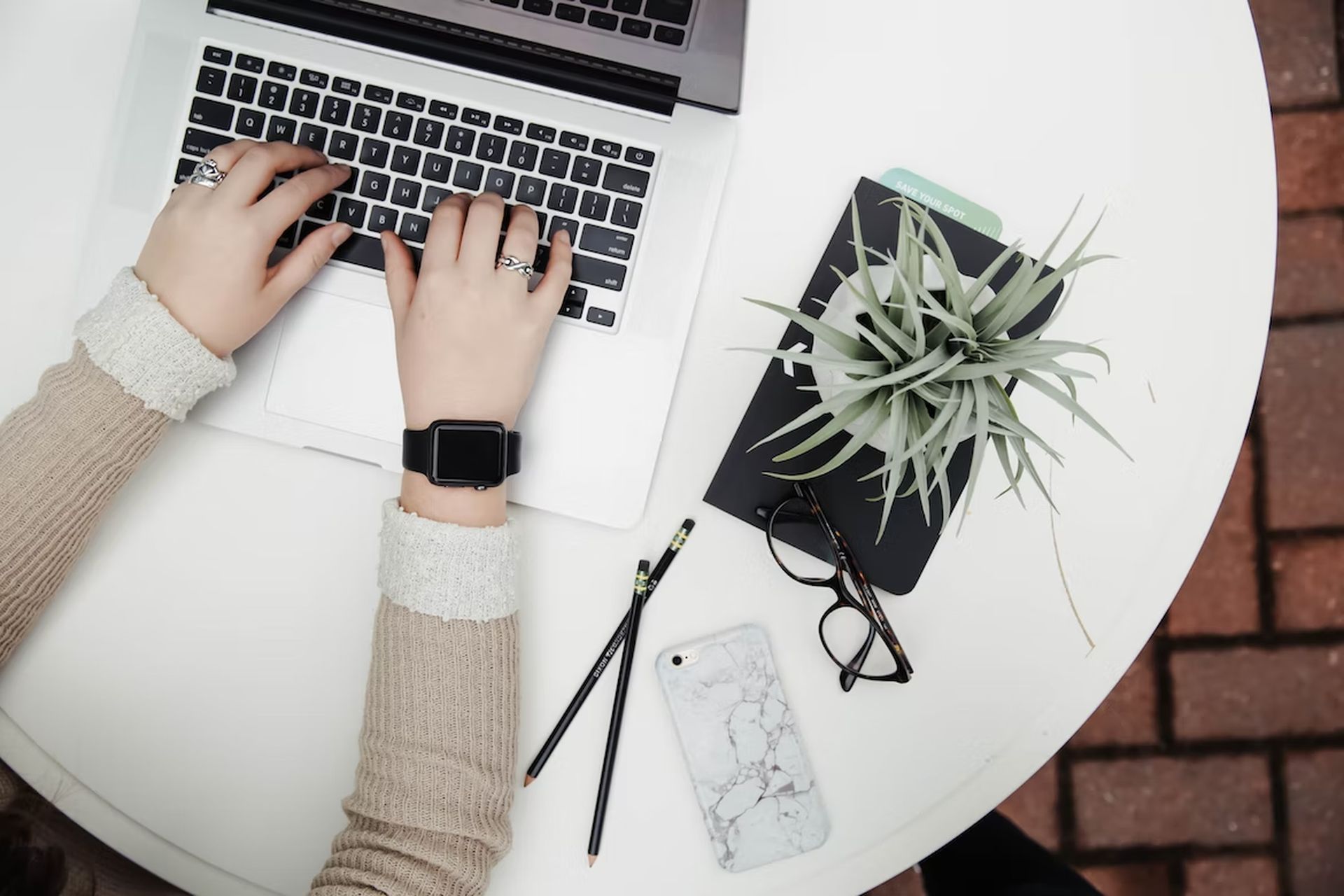
Conclusion
Data analytics and artificial intelligence have applications in all sectors of the economy and in all types of services, and they will continue to develop new uses in business, government, and academia. Healthcare, banking, education, media, and customer service are among the sectors likely to use AI in increasingly potent and widespread ways.
Build a wall around your sensitive data with advanced threat protection
Leveraging data to innovate is a cornerstone of the financial services industry and includes anything from financial modeling to risk and fraud detection to performing customer and credit analytics. Many businesses are using data science and machine learning to keep up with industry standards and rivals. Data science assists businesses in deriving knowledge from their data so they may make fact-based business choices while safeguarding private consumer data. Data analytics and artificial intelligence will change how we do business.