Breakthroughs in AI and innovations in applying blockchain for personal data control and monetization enable new ways to make money off of personal information that most people currently give away for free.
Here we highlight three data science and business model innovations, starting with breakthrough ML technology that learns on the fly.
This AI Bloodhound can hunt
There’s an emergent machine learning technology out there that offers a clever new way of finding and classifying unstructured content.
In geek-speak, the technology is a vertical, personalized search engine that doesn’t require expensive knowledge graphs.
In human speak, it’s a context-sensitive, human-in-the-loop search engine that uses search criteria and implicit user feedback to recommend high-quality results.
And in talk to me as you might a young child, or a golden retriever speak, it’s like an AI Bloodhound in that it is instantaneously trained by the “scent” of content to go find, score, and bring back content that has an implicitly similar “scent.”
In user experience speak, it’s like this:
- Start a search session by using examples of what you’re looking for
- Choose results you like to tell your personalized AI to “go find more results like this”
- And alert me when you find me something good (if you’re out and about)
Unlike other ML applications, which need to be trained using high-quality content that can take weeks or months – this AI learns on the fly. Also, unlike other ML models, which are monolithic and have a reputation for being expensive and including things like “AI bias,” with this AI, pristine engines are instanted at each user’s search session level. There isn’t one ML engine – there are as many as you need. And because the ML engines are pristine when instantiated, there is no AI Bias. Instead, it’s the human-in-the-loop that introduces the “bias” when the human gives the personalized ML engine examples of the types of results it should be finding and bringing back.
One of the areas where this AI is being used is hiring and recruiting, within the context of a unique personal data monetization community.
Hey fam, the FAAAM oligarchs won’t be too happy about this
There’s an emergent community platform model in which members are given the ability to provide their personal information, configure its availability to others in the community, and then choose how to monetize it.
Members own all their data and determine which community actors benefit from it (unlike the tech giants who take your data, do who-knows-what with it while making money hand over fist, and then pay you little to nothing for it).
Everything is stored securely thanks to blockchain technology, and only users (not the platform administrators) have the key to access it.
Opportunities are created for users to share their information with companies and other community members interested in purchasing it while also keeping that information safe and secure. AI expedites monetization by optimizing the matching of sellers and buyers.
An AI-powered staffing service that pays candidates to interview
One category of personal information that community members can choose to monetize is their resume. This is done via a Staffing Service “module” within the community and is powered by that AI Bloodhound technology. Both sides of the supply-demand equation experience value:
The user experience (UX) for Staffing Company users or enterprise HR users:
- Create a new job or requisition and configure the usual fields like role name, description, key requirements, and so on.
- Drag and drop examples of resumes that the client or staffing company believes would be great for the role. Think of it as giving the AI resumes the likes of which the recruiter would hope to see from candidates.
- Click the Save button.
That req is now its own AI-powered search session. When it runs a search, its results will include candidates whose resumes were implicitly similar to those used in step #2 above. The AI Bloodhound technology found resumes with the same “scent” as those it was trained on.
As recruiters review the search results, they select those resumes that they feel the AI got right. “These are the types of candidates I was hoping to find!”. With those results selected, they click the Search button again. The AI engine powering that specific requisition becomes instantly re-trained using the resumes that the recruiter just selected (positive model reinforcement). After only a couple of these cycles, the AI search results will reliably show candidates whose resumes were given a high AI score because they were implicitly and contextually similar to what the recruiter explicitly told the engine they were interested in.
It’s important to note that high-quality search results that the recruiters see don’t include candidates’ names or contact information at first. You’ll see why in a moment.
The UX for community members looking for jobs:
- When candidates configure their resume settings, they set the dollar amount they want to be paid to reveal their contact information and take an interview with a recruiter.
- After a candidate’s resume is uploaded or set as Active, it is analyzed and scored by all of the AI engines powering all of the jobs/requisitions created by the Staffing Companies.
- A candidate’s resume will be given high AI scores by those requisitions whose AI engines were trained by example resumes implicitly similar to the candidate’s
- A candidate is notified when there’s a position for which they’re a good fit, and the recruiter has agreed to pay the fee configured in #1.
- The candidate then coordinates with the recruiter to discuss the opportunity.
Winner winner, chicken dinner
The hiring manager wins because the AI found the type of candidate they were looking for. They are happy to pay the candidate’s fee to avoid the time and frustrations involved with a keyword search.
The candidate wins twice: 1) They found a job opportunity that seems to be an excellent fit for them, and 2) They got paid while doing so.
And of course, everybody wins as more people are employed in jobs that are a good fit for them. Better quality of work. Less turnover. This can only help with getting the economy on a path for returning to pre-Covid levels.
Peer-to-peer digital marketplace for personal information: a sharing economy to dwarf all others?
Companies like Uber and Airbnb showed the world the power of “Sharing Economies” and the value that can be unleashed when you take an otherwise idle asset and make it available for utilization.
This data control and monetization community is like a sharing economy, except instead of putting physical assets into play, it puts members’ personal data and intellectual capital into play.
There are many more people on the planet than there are cars, homes and apartments, private rooms, and other properties. Every person has personal information. Therefore everyone stands to benefit.
Except, of course, companies with business models that depend on people giving them their personal information for free.
Co-author:
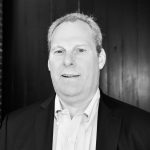
As a serial entrepreneur, Erik has started a number of companies as the main inventor and technologist. Being a veteran of the tech industry, his passion for innovation and belief in the power of creativity has led to the success of several leading startups and high-growth technology companies. As Founder and CEO of ImagineBC, his current mission is to unlock data for more equitable outcomes for users of data. He has kickstarted a new data economy and, in doing so, worked to align data practices with growing consumer expectations.
Prior to launching his latest venture, Erik was at the forefront of the human capital management and technology intersection with his company Benepay Technology. Benepay Technology, the parent company of ImagineBC, remains operational and well-positioned. Coupled with the successful activation of Benepay Technology, Erik also founded and, later, sold PowerPay software for $22 million. Erik is also the president of the Data Union, a global movement aimed at creating a more equitable and ethical data economy.
Erik’s industry accolades include his appointment to the Forbes Tech Council, Rolling Stone’s Culture Council, and the Radical Exchange.