Matt Hall has a PhD in sedimentology from the University of Manchester, UK, and 15 years’ experience in the hydrocarbon industry. He has worked for Landmark as a volume interpretation specialist, Statoil as an explorationist, and ConocoPhillips as a geophysical advisor. Matt has a broad range of interests, from signal processing to facies analysis, and from uncertainty modelling to knowledge sharing. Personally as someone with a Physics degree, I think it is really important we in the data science community learn a lot from the scientific modelling community. There is a lot of shared knowledge out there and one reason I am attending EuroSciPy in Cambridge this year.
Follow Peadar’s series of interviews with data scientists here.
Now it is time for another interview with a Data Scientist. Today I decided to be a bit different and I interviewed a professional geophysicist, who runs a consultancy in Nova Scotia, Canada.
1. What project have you worked on do you wish you could go back to, and do better?
If it wasn’t for the fact that revisiting old projects would mean missing out on future ones, I would say ‘All of them’. I learn something new on almost everything I work on, and on the downtime in between. I occasionally do go back and re-do things with new tools or insights, and often projects come back around naturally, in one form or another, but I leave a lot untouched. I can’t even imagine, for example, what I could do with almost every aspect of my postgraduate research with what I have today.
Oddly, and worryingly, the reverse is true too. Sometimes I wish I had the same facility with mathematics now as I did at the age of 20. There’s always stuff you forget — perhaps learned but did not actually need at the time, so you never applied it. I guess this means that learning is an x-steps-forward-y-steps-back situation. I just hope x > y.
2. What advice do you have to younger analytics professionals and in particular PhD students in the Sciences?
At the risk of giving a rather tired answer, the number one things for anyone in science is: learn to program. While you’re doing that, learn a bit about the Linux shell (yes, this means dumping Windows as soon as possible) and version control. These are fundamental skills and it could not be easier to get started today. As for the language, pick Python or R, or maybe even JavaScript, or actually just anything.
Here’s something I wrote for geoscientists about learning to program: http://www.agilegeoscience.com/blog/2011/9/28/learn-to-program.html
3. What do you wish you knew earlier about being a data scientist?
I’m not a data scientist as such, but a scientist. Data science seems like a fun place to play with code and data, and I wish it had been a thing when I was finishing my education. I must say though, possibly horribly tangentially, that I sense the field of ‘data science’ seems in danger of moving so quickly that it trips over itself. ‘Data’ has to include an awareness of provenance, bias, and the statistics of sampling. ‘Science’ has to include rigorous testing, peer review, and reproducibility. And the analysis of data should build on the history of statistics, especially with respect to the handling of uncertainty. This isn’t to say ‘slow down’ or ‘respect your elders’, it’s more about not repeating the mistakes of the past with the scale and speed of today. I highly recommend joining the Royal Statistical Society, and reading every issue of Significance.
4. How do you respond when you hear the phrase ‘big data’?
I know some people freak out when they hear those words, but I don’t mind ‘big data’. It seems to communicate in shorthand something we all understand. Like any jargon, different people often mean different things, so one has to clarify. But it’s clearly taken off so it probably doesn’t matter what we think about it at this point.
I work in applied geophysics. Lots of people in the field roll their eyes at ‘big data’, grumbling that “we’ve been doing that for years”. But they have missed the point entirely. Big data isn’t just lots of data, it implies something about all the other components of the analytics cycles too — storage, retrieval, and analysis.
What is the most exciting thing about your field?
Geophysics is a fantastically hard big data problem. All interesting problems are inverse problems, and learning the geological history of the earth from some acoustic or electromagnetic data recorded at the surface is a very hard inverse problem. The earth is about as complex a system as you can get, so its equation (so to speak) has infinite variables, so any question you can ask is woefully underdetermined. As a bonus, geological hypotheses are incredibly hard to test on human timescales. And we never get to find out the actual answer by, say, visiting the Cretaceous.
Here’s a vertical and a roughly horizontal slice through a dataset offshore Nova Scotia, where I live…
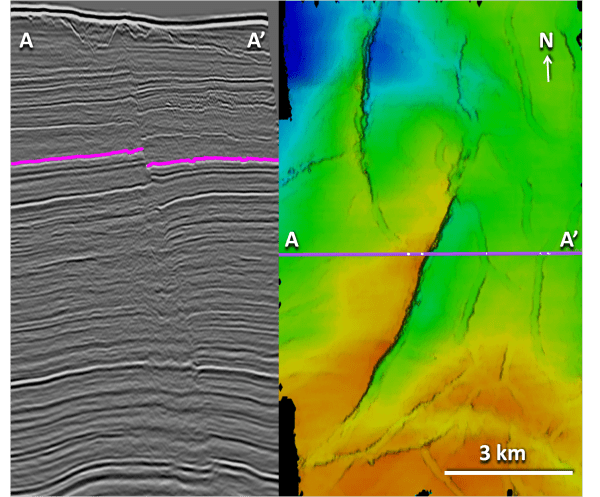
In an attempt to get reasonable answers, we collect huge amounts of data using ships and drills and dynamite and other exciting things. All the data came from the same earth, but of course every channel has its own response, its own noise, and its own bias. Reducing 200 billion data samples to an image of the earth is a hard optimization problem, but it 60% of the time, it works every time.
What gets me excited every day is that we’ve only scratched the surface of what we can learn about the earth from seismic data.
6. How do you go about framing a data problem – in particular, how do you avoid spending too long, how do you manage expectations etc. How do you know what is good enough?
I usually have a specific purpose to work towards, and often a time constraint as well. So you do what you can to deliver the required result (for me, this might be a set of maps, or a ranked list of locations) in the time available.
Scoping projects is hard. The concept of a ‘project’ is somewhat at odds with how scientists work — with a lot of unknowns. It’s better to be more agile — do something easy quickly, then review and iterate. This isn’t always compatible with working to time and resource constraints, especially if you’re working with people with a strong ‘waterfall’ project mindset.
You can’t beat meeting the client (there’s always a client — someone who needs your work) face to face. The more you can iterate on the plan, the better. And the more space you can leave for the stuff you don’t know yet, the better. There’s no point committing to something, then finding out that the available data. Have contingencies for everything. Report back continuously, not after a problem has started eating things for breakfast. Be open and transparent about everything. All common sense, but not easy to stick to once you get stuck in.
Peadar Coyle is a Data Analytics Professional based in Luxembourg. He has helped companies solve problems using data relating to Business Process Optimization, Supply Chain Management, Air Traffic Data Analysis, Data Product Architecture and in Commercial Sales teams. He is always excited to evangelize about ‘Big Data’ and the ‘Data Mentality’, which comes from his experience as a Mathematics teacher and his Masters studies in Mathematics and Statistics. His recent speaking engagements include PyCon Sei in Florence and he will soon be speaking at PyData in Berlin and London. His expertise includes Bayesian Statistics, Optimization, Statistical Modelling and Data Products