- AI-powered drug development can shorten the time it takes to acquire and access information, cutting pharmaceutical development time in half and keeping the cost of new medications under control.
- This is becoming increasingly crucial as the cost of discovering and developing new drugs rises.
- Despite several accomplishments, certain challenges must be overcome before pharmaceutical (pharma) firms may reap the benefits of AI.
- We’ve compiled a list of AI’s most prevalent issues for drug development.
AI can speed up the procedures of gathering and accessing information, cutting medication development time in half and keeping the cost of new medicines in check. This is becoming increasingly important as the cost of identifying and developing medications rises.
Importance of AI-powered development
How much does it cost to produce a new drug? According to a 2020 study, the typical research and development expenditure for a new therapy was $985 million. The high trial failure rate contributed significantly to this expense. Approximately seven out of every eight compounds that reach the clinical testing process never make it to the market.
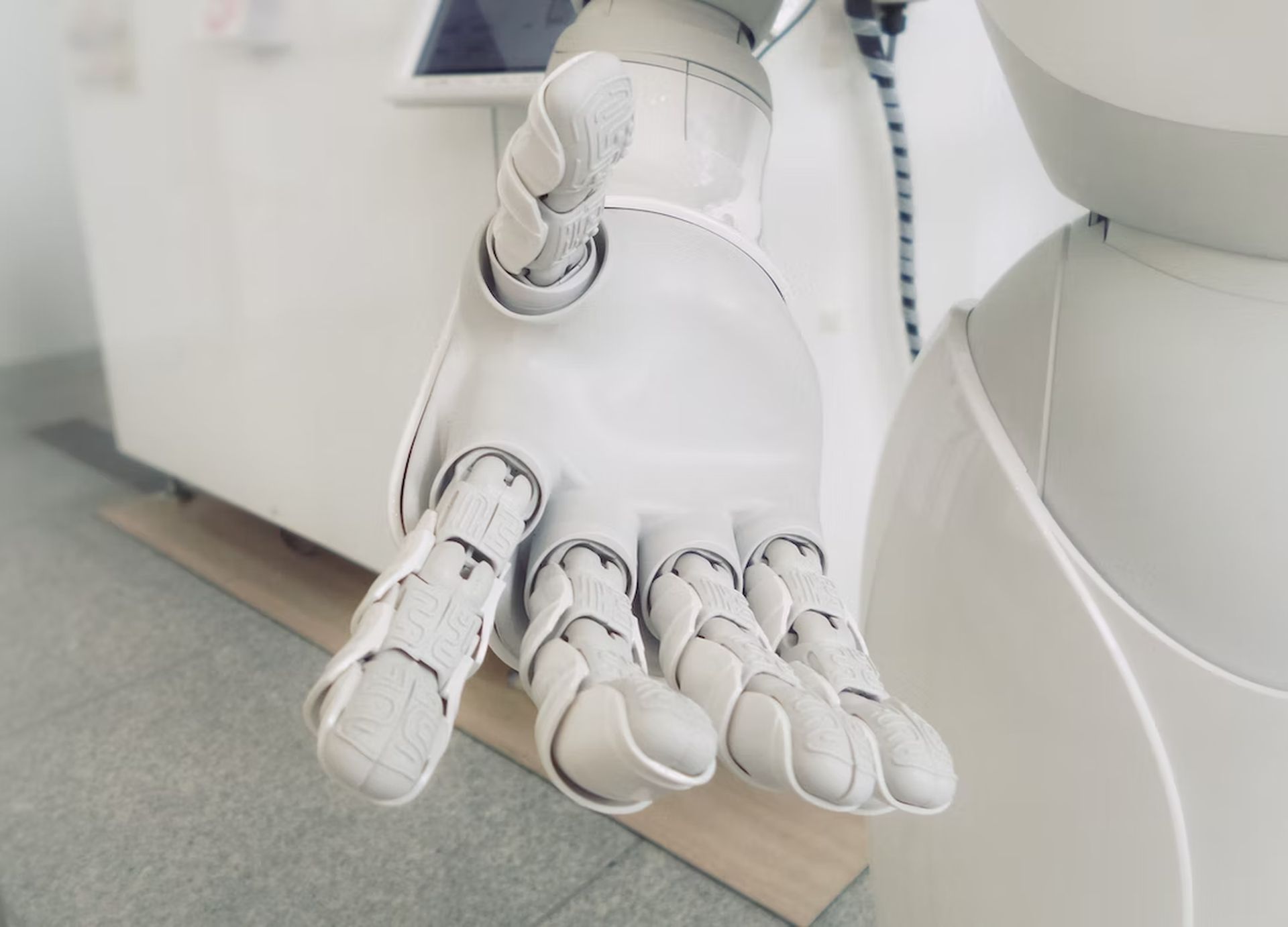
This is where artificial intelligence comes in. AI can discover previously unknown patterns, resulting in new knowledge of illnesses and the treatments developed to treat them. Astra Zeneca, for example, employs machine learning models to find which genes might produce resistance to cancer therapies more rapidly, while Samsung has developed an app to detect early Covid-19 infection.
Challenges facing AI-powered drug development in the future
Despite the numerous achievements, particular hurdles must be solved for pharmaceutical (pharma) enterprises to gain the benefits of AI. Here, we gathered the most common problems faced by AI-powered drug development.
Smaller datasets available
To learn, most AI systems require large datasets. Creating huge data sets for each type of medical condition is difficult due to the enormous range of diseases and illnesses and the relatively small number of incidents.
On average, machine learning models in the pharmaceutical sector require 2 to 3 years of historical data to function well. Because of the huge number of mergers and acquisitions, this aim might be difficult to achieve, especially when the original source of the data is no longer available. Because pharma data sets are often smaller, with fewer patients and fewer observations per patient, gaining useful insights is more difficult.
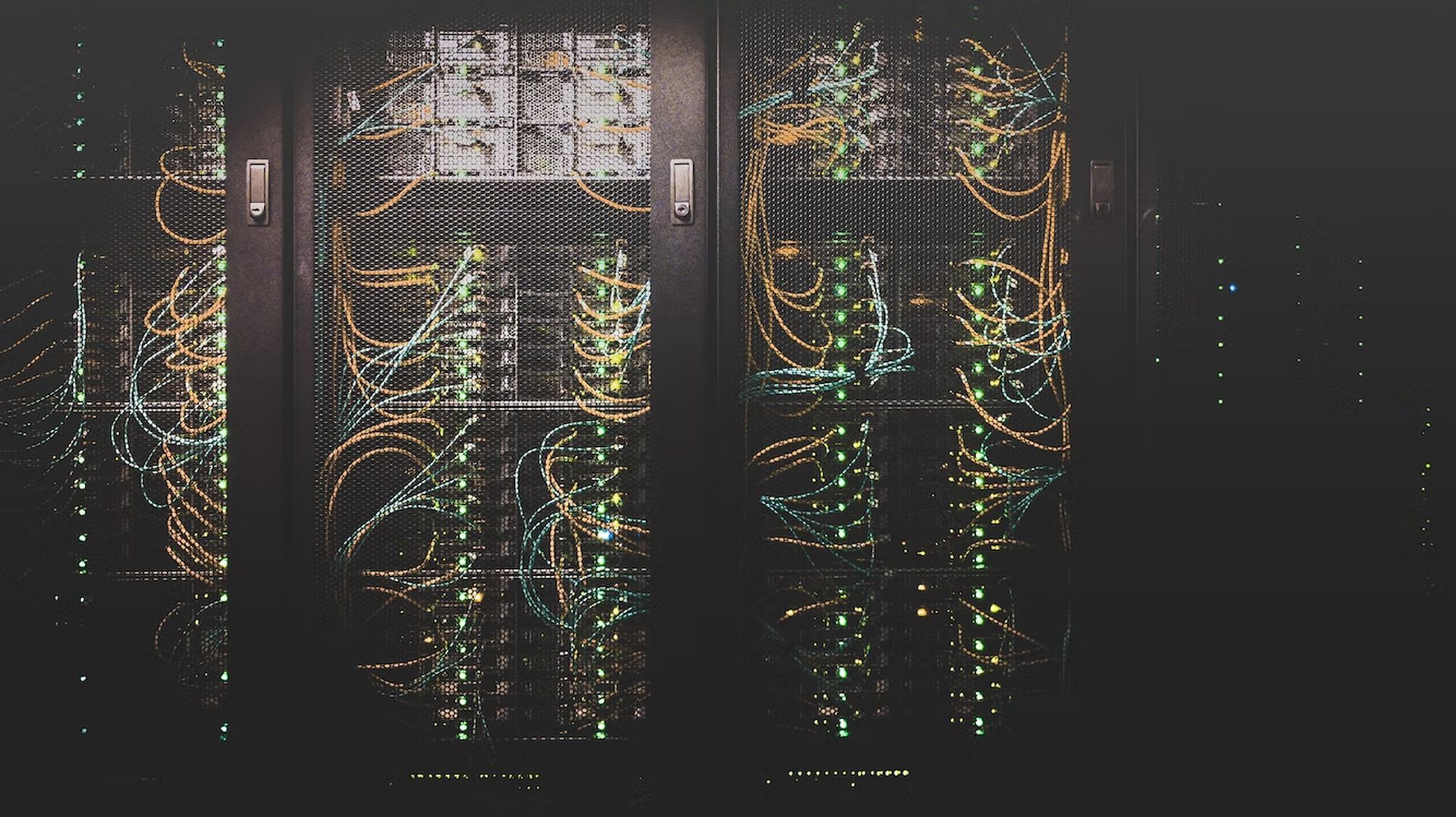
The complexity of the data
At the same time, while there may be fewer data sets, each dataset may have significantly more features. Patient data may comprise historical and present health or sickness information, treatment history, lifestyle decisions, and genetic data.
Biometric data, any quantifiable physical feature measured by a sensor or wearable device, can also be included. Consequently, patient data may comprise alphanumeric information, x-rays, pathology reports, and clinical test results in various formats such as JPEG/JPG and digital imaging and communication for medicine (DICOM). Any AI-powered drug development must be able to handle a wide range of complicated data.
Complicated data labeling
Data labeling is more difficult and needs highly skilled expertise. Consider the many forms of knowledge necessary to recognize the skeletal, internal-organ, nervous system, and vascular abnormalities in X-Ray pictures. Finding the appropriate knowledge is difficult, but labeling may be tiresome and time-consuming. Each brain scan used for cancer screening must be evaluated by doctors (typically three or more), with each inspection taking 5 to 15 minutes.
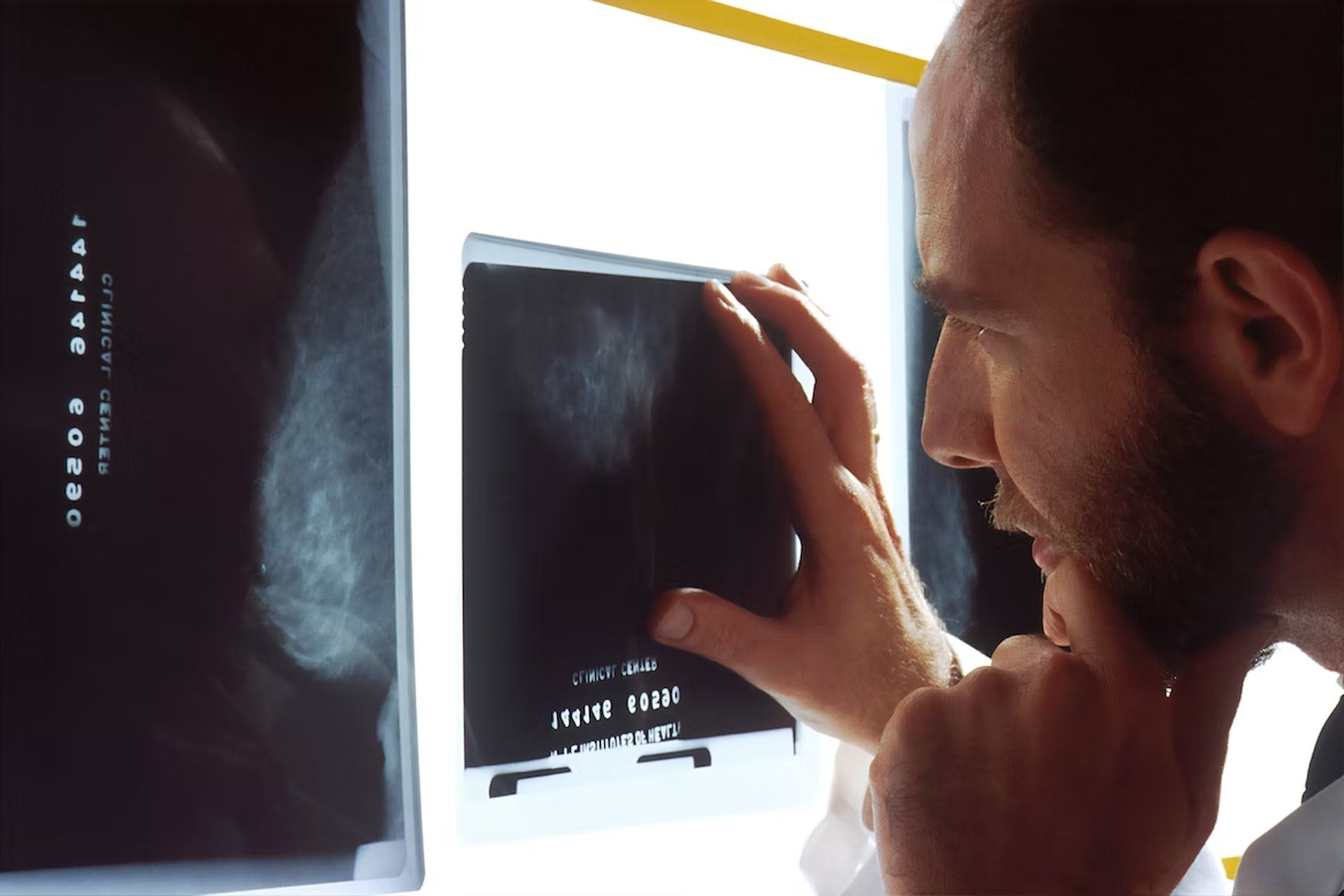
Data bias
Several groups have a lengthy history of missing or misrepresented in medical databases. Misdiagnoses and tragic results might occur if the training data is not representative of the total population. MLOps protocols and Machine learning (ML) model scoring must be built to monitor and detect drift with a continuous feedback loop for AI ethics and transparency. A diverse ML team should regularly test the models to promote transparency and remove data bias from machine learning models.
Absence of data standards
The industry must establish its own definition of what constitutes a good data set. Organizations may collect and use multiple data types, use different coding methods in their systems, use null or dummy data when needed information is unavailable, and document demographic data inconsistently. Companies are still unsure how to replicate study results without well-defined criteria. Once it is obvious how to construct a legitimate data collection, it may be more easily used by other organizations to study further.
The Medical Field is Changing Because of Artificial Intelligence
Shortage of available biologic data
Biological databases are essential in bioinformatics. They provide scientists with access to a wide range of biologically relevant data, including genomic sequences from an expanding number of organisms.
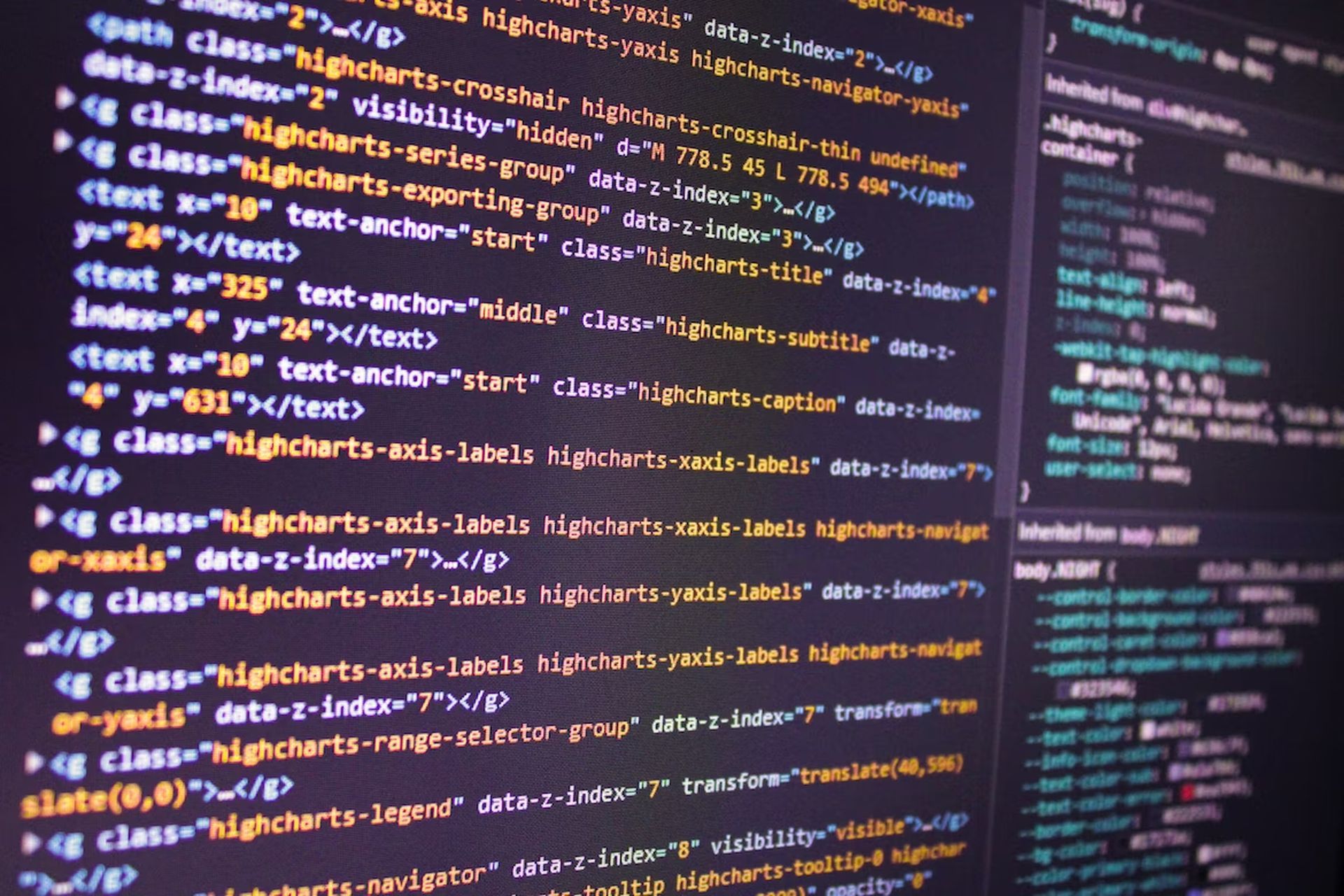
There are numerous ways to use biological data, such as comparing sequences to develop a theory about the function of a newly discovered gene, examining known 3D protein structures to discover patterns that can help predict how the protein folds, or investigating how proteins and metabolites in a cell collaborate to make the cell function. AI-powered drug development relies on this data to understand how patients respond to medicinal treatments.
Regulations in the sector
The pharmaceutical sector is heavily regulated, demanding full disclosure and transparency at every stage of the medication development process. This necessity frequently lengthens and increases the cost of AI-powered drug development. Pharma firms should work with authorities to simplify this process for the benefit of everyone. Both regulators and corporations may use AI and other digital transformation projects to increase the value effectiveness of regulatory activities.
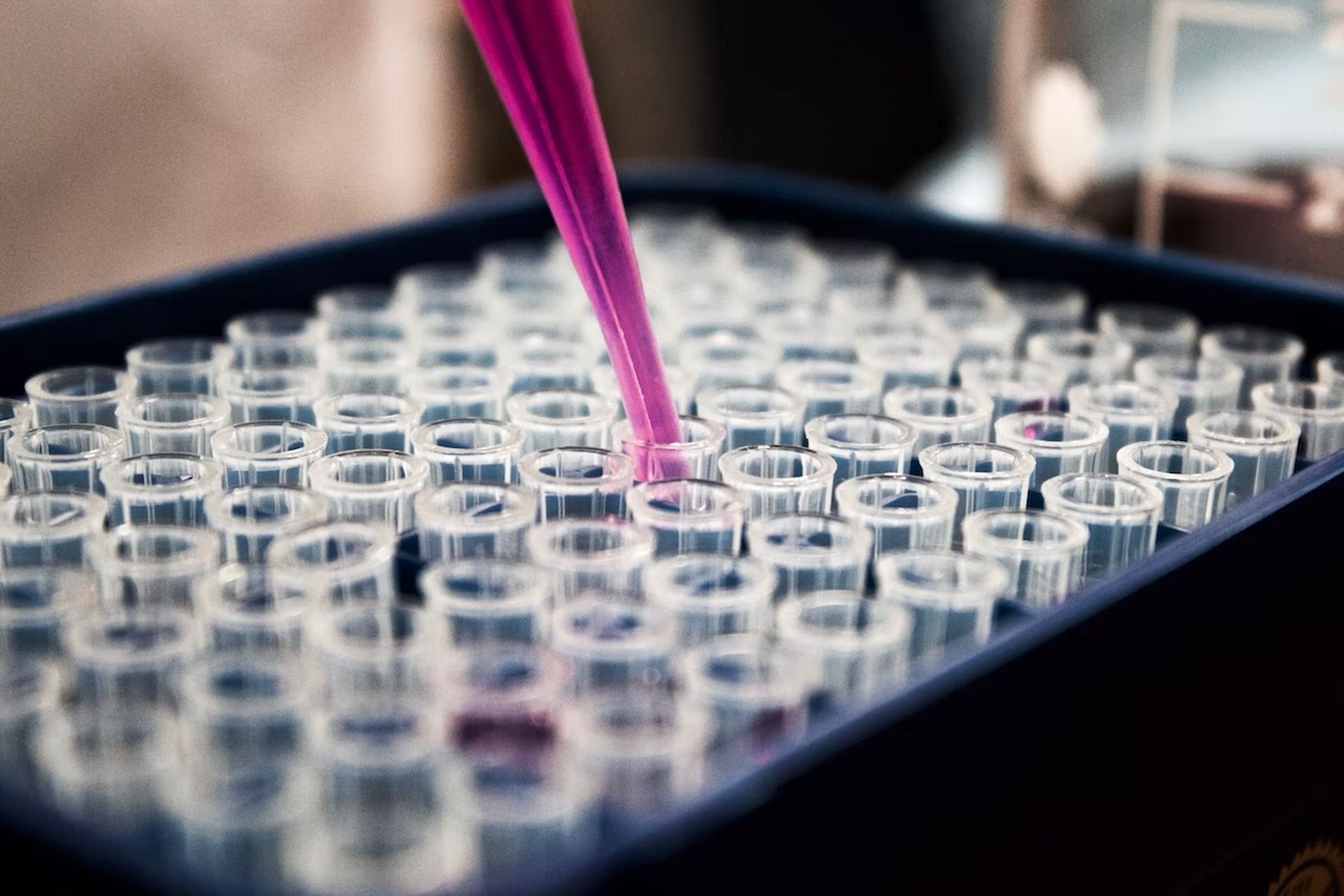
Other hurdles that AI-powered drug development face
In addition to these issues, pharma businesses face standard implementation roadblocks. For example, a flexible infrastructure is required to gather, validate, operate applications, perform data governance, and grow. New technologies, such as greater transfer learning and MLOps platforms, can assist train models and streamline the process of transferring machine learning models into production.
New artificial intelligence can diagnose a patient using their speech
The increased competition to identify therapies faster will enhance the need for AI-powered drug development. The problems of evaluating medical data are growing more complicated. Still, the rate of innovation in developing AI tools and technology that will allow pharma firms to unearth smarter insights faster is also increasing.