By now, it’s almost impossible to not have heard the term Big Data- a cursory glance at Google Trends will show how the term has exploded over the past few years, and become unavoidably ubiquitous in public consciousness. But what you may have managed to avoid is gaining a thorough understanding what Big Data actually constitutes.
The first go-to answer is that ‘Big Data’ refers to datasets too large to be processed on a conventional database system. In this way, the term Big Data is nebulous- whilst size is certainly a part of it, scale alone doesn’t tell the whole story of what makes Big Data ‘big’.
When looking for a slightly more comprehensive overview, many defer to Doug Laney’s 3 V’s:
1. Volume
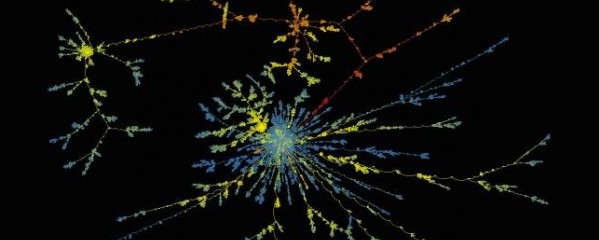
Visualisation of the spread of 250,000 Facebook comments- read more here.
100 terabytes of data are uploaded daily to Facebook; Akamai analyses 75 million events a day to target online ads; Walmart handles 1 million customer transactions every single hour. 90% of all data ever created was generated in the past 2 years.
Scale is certainly a part of what makes Big Data big. The internet-mobile revolution, bringing with it a torrent of social media updates, sensor data from devices and an explosion of e-commerce, means that every industry is swamped with data- which can be incredibly valuable, if you know how to use it.
2. Velocity
In 1999, Wal-Mart’s data warehouse stored 1,000 terabytes (1,000,000 gigabytes) of data. In 2012, it had access to over 2.5 petabytes (2,500,000 gigabytes) of data.
Every minute of every day, we upload 100 hours of video on Youtube, send over 200 million emails and send 300,000 tweets. ‘Velocity’ refers to the increasing speed at which this data is created, and the increasing speed at which the data can be processed, stored and analysed by relational databases. The possibilities of processing data in real-time is an area of particular interest, which allows companies to do things like display personalised ads on the web pages you visit, based on your recent search, viewing and purchase history.
3. Variety
Gone are the days when a company’s data could be neatly slotted into a table and analysed. 90% of data generated is ‘unstructured’, coming in all shapes and forms- from geo-spatial data, to tweets which can be analysed for content and sentiment, to visual data such as photos and videos.
The ‘3 V’s’ certainly give us an insight into the almost unenvisionable scale of data, and the break-neck speeds at which these vast datasets grow and multiply. But only ‘Variety’ really begins to scratch the surface of the depth- and crucially, the challenges- of Big Data. An article from 2013 by Mark van Rijmenam proposes four more V’s, to further understand the incredibly complex nature of Big Data.
4. Variability
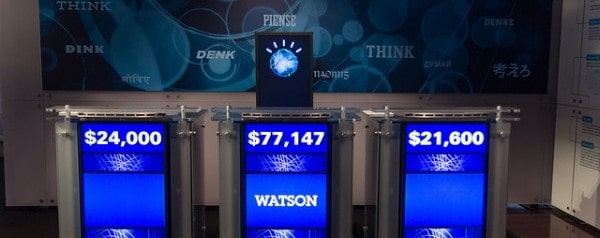
The Watson supercomputer; find out more here.
Say a company was trying to gauge sentiment towards a cafe using these ‘tweets’:
“Delicious muesli from the @imaginarycafe- what a great way to start the day!”
“Greatly disappointed that my local Imaginary Cafe have stopped stocking BLTs.”
“Had to wait in line for 45 minutes at the Imaginary Cafe today. Great, well there’s my lunchbreak gone…”
Evidently, “great” on its own is not a sufficient signifier of positive sentiment. Instead, companies have to develop sophisticated programmes which can ‘understand’ context and decode the precise meaning of words through it. Although challenging, it’s not impossible; Bloomberg, for instance, launched a programme that gauged social media buzz about companies for Wall Street last year.
5. Veracity
Although there’s widespread agreement about the potential value of Big Data, the data is virtually worthless if it’s not accurate. This is particularly true in programmes that involve automated decision-making, or feeding the data into an unsupervised machine learning algorithm. The results of such programmes are only as good as the data they’re working with.
Sean Owen, Senior Director of Data Science at CloudEra, expanded upon this: ‘Let’s say that, in theory, you have customer behaviour data and want to predict purchase intent. In practice what you have are log files in four formats from six systems, some incomplete, with noise and errors. These have to be copied, translated and unified.’ Owens’ US counterpart, Josh Wills, said their job revolves so much around the cleaning up of messy data that he was more a ‘data janitor’ than a data scientist.
What’s crucial to understanding Big Data is the messy, noisy nature of it, and the amount of work that goes in to producing an accurate dataset before analysis can even begin.
6. Visualisation

A visualisation of Divvy bike rides across Chicago; find out more here.
Once it’s been processed, you need a way of presenting the data in a manner that’s readable and accessible- this is where visualisation comes in. Visualisations can contain dozens of variables and parameters- a far cry from the x and y variables of your standard bar chart- and finding a way to present this information that makes the findings clear is one of the challenges of Big Data.
It’s a problem that’s spurned a burgeoning market- new visualisation packages are appearing all of the time, with AT&T announcing their offering, Nanocubes, just this week.
7. Value
The potential value of Big Data is huge. Speaking about new Big Data initiatives in the US healthcare system last year, McKinsey estimated if these initiatives were rolled out system-wide, they “could account for $300 billion to $450 billion in reduced health-care spending, or 12 to 17 percent of the $2.6 trillion baseline in US health-care costs”. However, the cost of poor data is also huge- it’s estimated to cost US businesses $3.1 trillion a year. In essence, data on its own is virtually worthless. The value lies in rigorous analysis of accurate data, and the information and insights this provides.
So what does all of this tell us about the nature of Big Data? Well, it’s massive and rapidly-expanding, but it’s also noisy, messy, constantly-changing, in hundreds of formats and virtually worthless without analysis and visualisation.
In essence, when the media talk about Big Data, they’re not just talking about vast amounts of data that are potential treasure troves of information. They’re also talking about the business of analysing this data- the way we pick the lock to the treasure trove. In the world of Big Data, data and analysis are totally interdependent- one without the other is virtually useless, but the power of them combined is virtually limitless.
(Featured image source: Intel Free Press)
Eileen McNulty-Holmes – Editor
Eileen has five years’ experience in journalism and editing for a range of online publications. She has a degree in English Literature from the University of Exeter, and is particularly interested in big data’s application in humanities. She is a native of Shropshire, United Kingdom.
Email: [email protected]
Thanks for the shout out Eileen. Yes, many have posited other Vs like these over the years, but fail to realize that only the original three (volume, velocity, variety) are dimensions of “bigness”. The others are either attributes of all/any data or are aspirational (e.g. value).
See @SethGrimes excellent piece on this: http://www.informationweek.com/big-data/commentary/big-data-analytics/big-data-avoid-wanna-v-confusion/240159597.
And see my blog on what Batman thinks of additional V’s. http://goo.gl/wH3qG 🙂
BTW…Gartner has a 12Vs model for defining all the key characteristics of information. And we produced a toolkit for measuring over a dozen distinct data quality characteristics.
Cheers,
Doug
–Doug Laney, VP Research, Gartner, @doug_laney
Oops that was my original “3Vs” piece from 2001. Here’s the Batman piece: http://blogs.gartner.com/doug-laney/batman-on-big-data/
Thanks for getting in touch Doug. I absolutely love the Batman piece!
I totally agree that the 3 V’s offer a comprehensive overview of the definitional qualities of Big Data, and a smart way of thinking about Big Data for those familiar with the term. But I think the ‘wanna-Vs’ have value for helping newcomers understand the depth and challenges of Big Data. I agree they’re not absolute definitions, but I do think they offer an insight into the complexity of Big Data for those previously unacquainted with it.
As I said, additional “V”s are qualities of all/any data. They are not specific to the magnitude issues of Big Data, and certainly not definitional. I think they only serve to confuse people about the “bigness” challenges of big data. BTW…Gartner years ago identified a dozen qualities of data in general. We published a toolkit on how to measure each. –Doug Laney, VP Research, Gartner, @doug_laney
Hi Eileen, could you please cite the source for this article: https://datafloq.com/read/3vs-sufficient-describe-big-data/166, which I published on August 7, 2013 and which is also part of my book Think Bigger, which was published on April 1, 2014. Thanks.
Hi Mark, Eileen already credited you properly when the article was first published. If you take a look at point #3, “Variety”, Eileen writes: “An article from 2013 by Mark van Rijmenam proposes four more V’s, to further understand the incredibly complex nature of Big Data”, and links to your article https://datafloq.com/read/3vs-sufficient-describe-big-data/166.
Hi Mark. Eileen credited you properly when she first published the article in May 2014. If you read the article, you’ll see that in point nr. 3, “Variety”, she writes: “An article from 2013 by Mark van Rijmenam proposes four more V’s, to further understand the incredibly complex nature of Big Data.”, linking to your post https://datafloq.com/read/3vs-sufficient-describe-big-data/166
Hi Mark. Eileen credited you properly when she first published the article in May 2014. If you read the article, you’ll see that in point nr. 3, “Variety”, she writes: “An article from 2013 by Mark van Rijmenam proposes four more V’s, to further understand the incredibly complex nature of Big Data.”, linking to your post https://datafloq.com/read/3vs-sufficient-describe-big-data/166
Thanks for pointing that out, apologies, I must have missed that.