Devices were once valued only for their direct function. We dreamed, invented, and benefited. We continued to develop our ideas as time passed. We have pocketed more processing power than early spacecrafts and succeeded in connecting the whole world at this point. Data wells out from this digital world we create, and it grants valuable secrets about the real world when tinkered with the right tools. This is data processing technology history:
Manual data processing
The term “data processing” was first used in the 1950s, although data processing functions have been done manually for millennia. Bookkeeping, for example, entails activities such as recording transactions and generating reports like the balance sheet and cash flow statement. Mechanical or electronic calculators helped to speed up completely manual procedures.
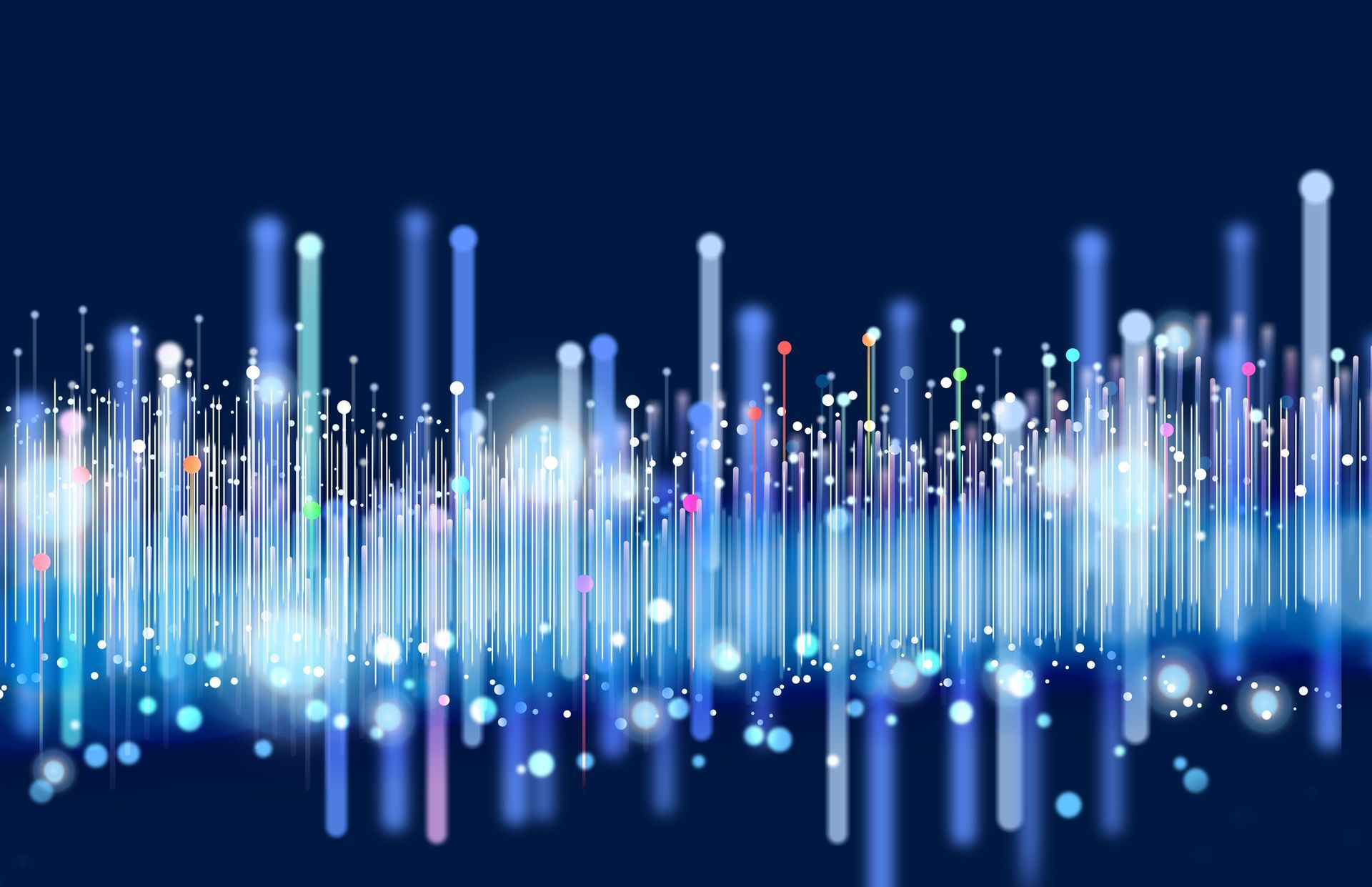
Punch cards
Computers have revolutionized the world of business in many ways, resulting in a clear demand for data processing. During the early days, computer scientists had to create unique programs for data processing on punch cards.
The evolution of programming languages has been defined in terms of the evolution of hardware architecture. The initial ones were assembly language, followed by more purposeful programming languages like Fortran, C, and Java. During the prehistoric big data era, programmers would use these languages to construct purpose-built programs for specific data processing activities.
Nevertheless, the computing platform was restricted to a select few with a programming background, preventing wider adoption by data analysts or the broader business community who wished to process information and make certain decisions.
The development of the database around the 1970s was the next logical step. Traditional relational database systems, such as IBM’s DB2 from 1977 onwards, allowed SQL and expanded data processing across a broader audience.
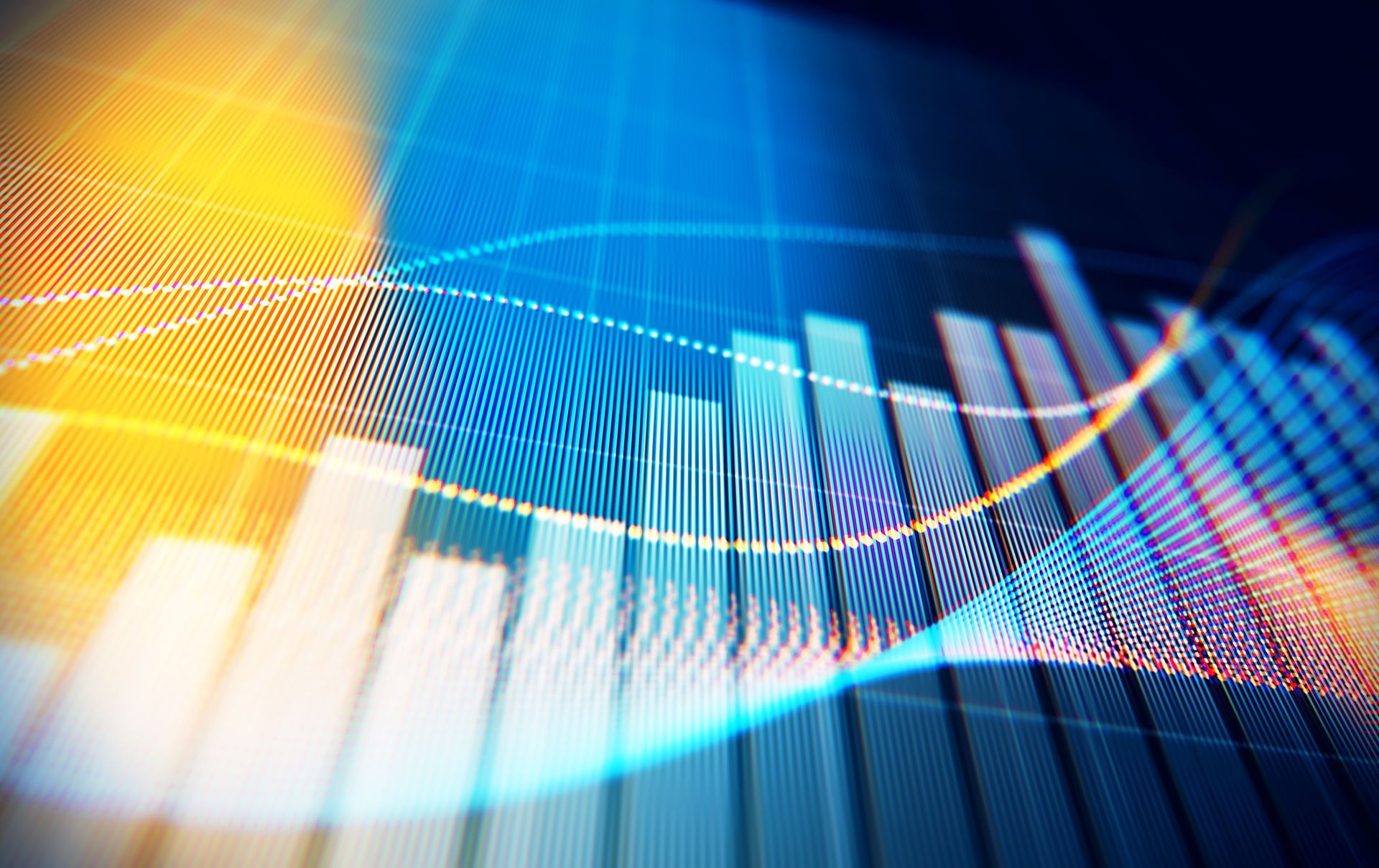
SQL
SQL is a standardized and descriptive query language that reads somewhat like English. As a result, more individuals could access data processing, which meant they didn’t have to hire expensive coders to write custom case-by-case programs and analyze data. SQL also broadened the scope of data processing tools, adding more and different applications relevant to data processing, such as business apps, churn rate analyses, average basket size fluctuations, year-over-year growth rates, and so on.
Big Data
The era of Big Data began with Google’s MapReduce paper, which describes a basic model composed of two primitives: map and reduces. The MapReduce paradigm enabled parallel computations across a large number of different computers. Parallel computations have long been possible via various computers, supercomputers, and MPI systems. However, the advent of MapReduce made it available to a much wider audience.
The Apache Hadoop framework was originally created at Yahoo! and made open source. Hadoop has been embraced by various organizations, and many Big Data firms started out as Hadoop developers. Hadoop introduced a new paradigm for data processing: the ability to save information in a distributed file system or storage (such as HDFS for Hadoop) that may be examined/queried later.
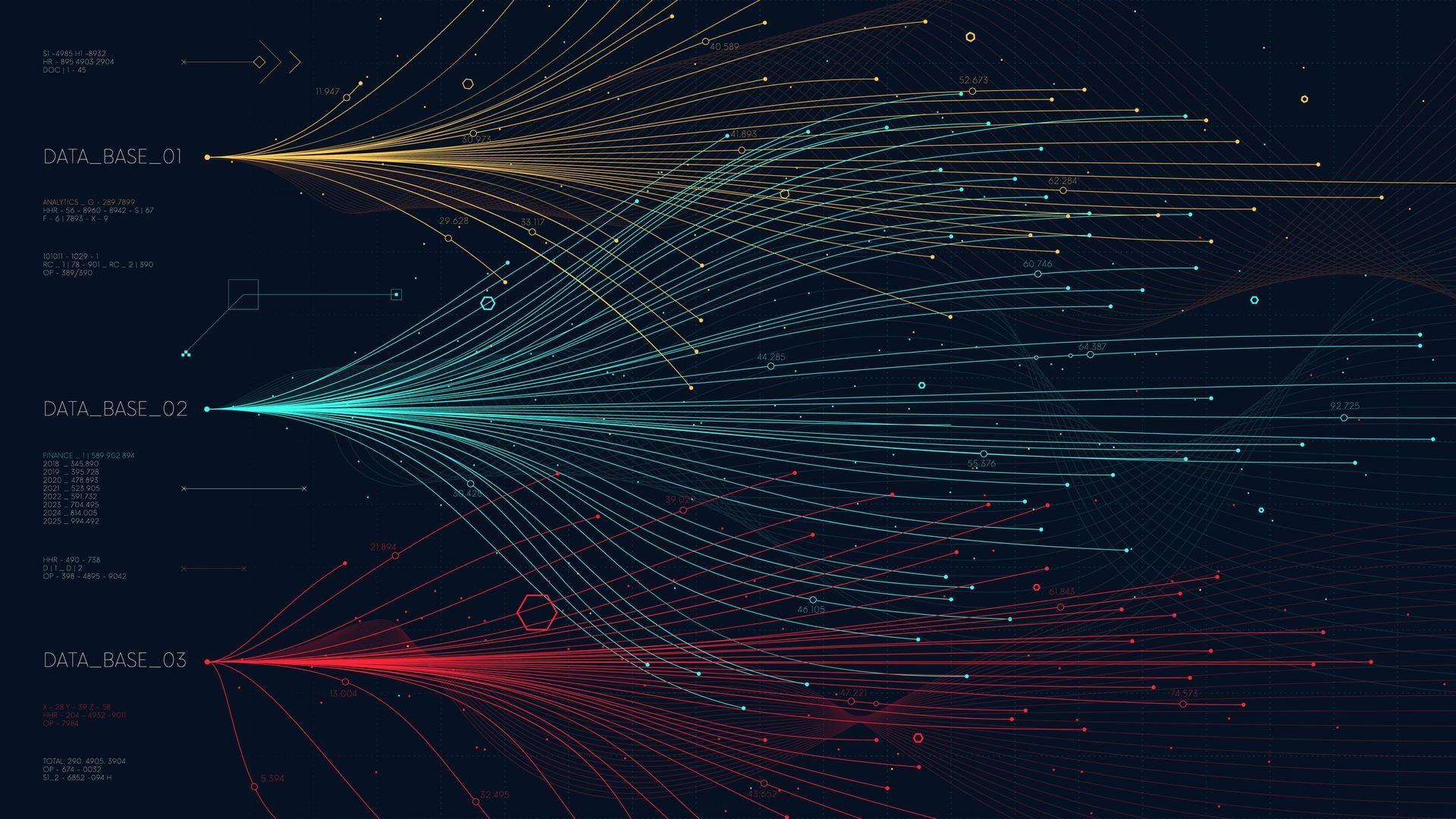
The first step in the Hadoop road was custom programming by a certain “cast” of people who could build programs to execute SQL queries on data stored in a distributed file system, such as Hive or other storage platforms.
The development of Big Data was accelerated with the introduction of Apache Spark. Spark made it possible to parallelize computations and take batch processing to new heights. Batch processing, as previously stated, is the process of putting data into a storage system before executing computations on it. The fundamental notion is that your data is stored somewhere while you carry out computations to obtain insights based on past information regularly (daily, weekly, or hourly). These computations aren’t always active and need a start and end date. Consequently, you must rerun them regularly for current results.
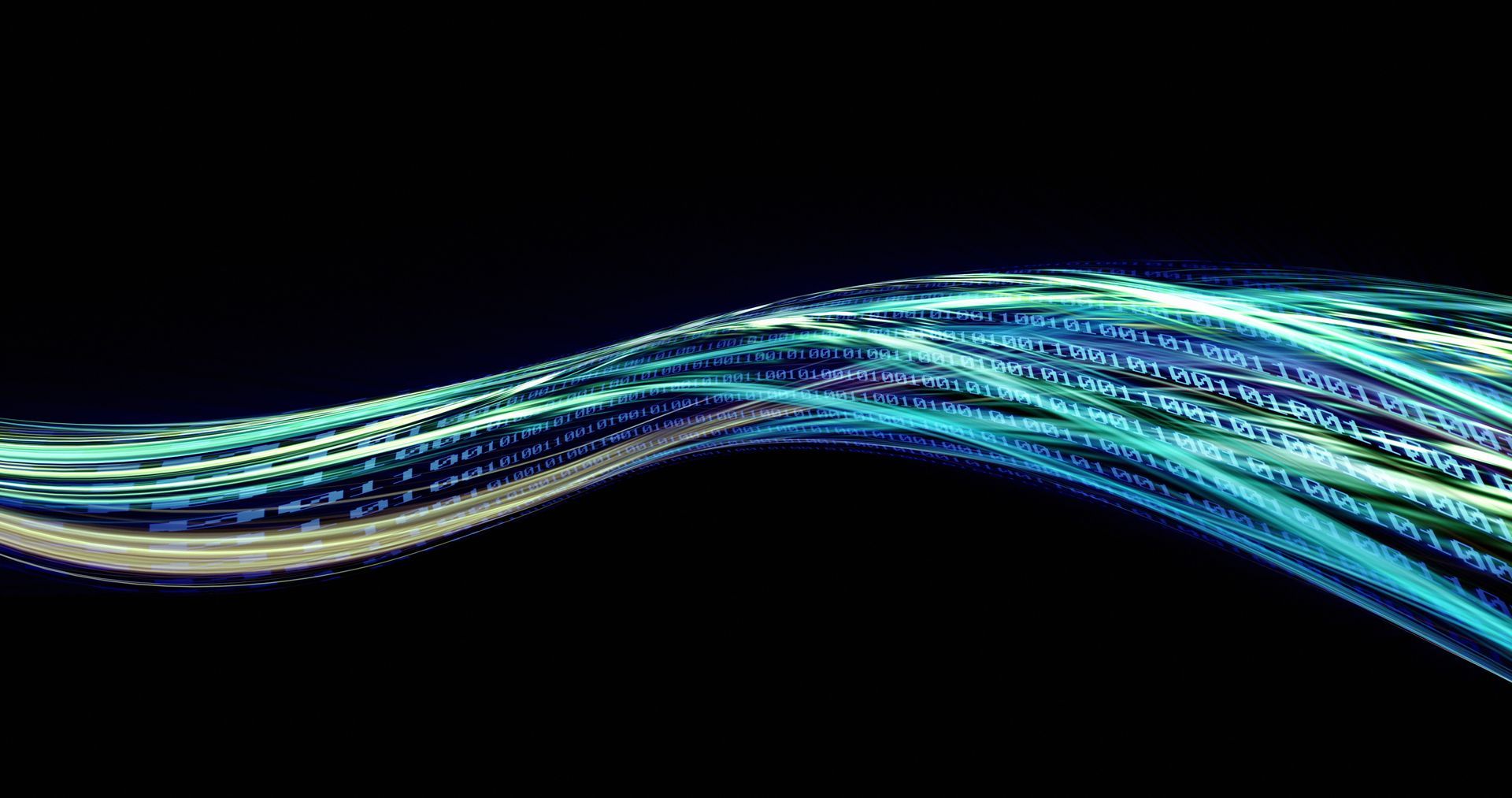
Stream processing
The advent of stream processing was a significant step toward achieving Big Data goals. This technology allowed applications to be developed that could run indefinitely.
The data stream processing revolutionized the data protection industry by shifting from a request-response mentality, where data is kept before fraud case investigation, to one where you ask questions first and then obtain real-time data as it happens.
Stream processing allows you to build a fraud detection system that is operational 24/7. It captures events in real-time and provides insight into when credit card fraud is being committed, preventing it from happening. This is perhaps one of the most important changes in data processing since it allows for real-time insights into what’s going on in the world.
The development of open-source data processing has followed a typical pattern: a new framework is introduced to the market (e.g., a relational database, batch processing, or stream processing) that is initially accessible only to certain people (programmers). The introduction of SQL into the framework makes it more accessible to a larger audience that does not require programming for complex data processing.
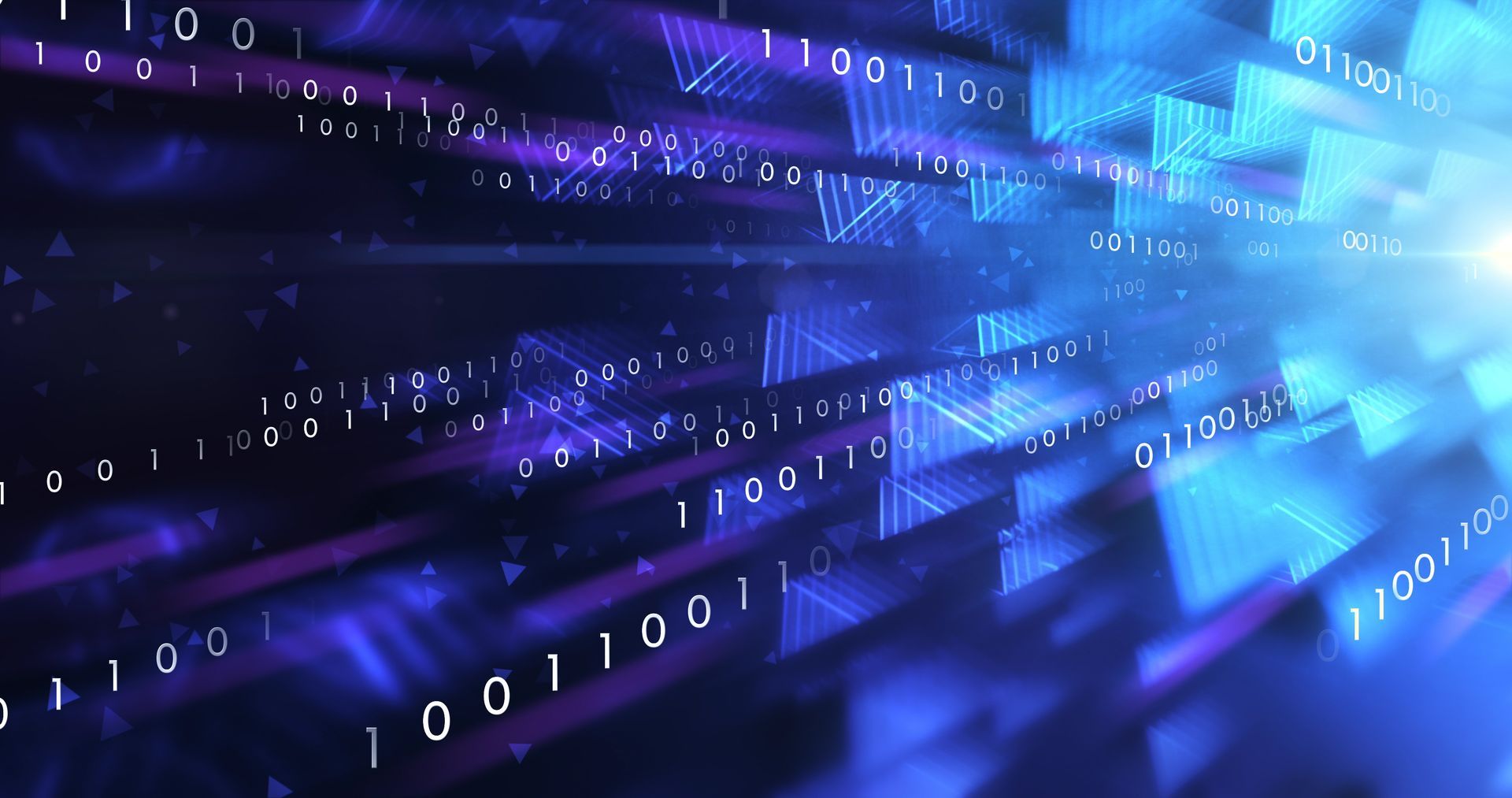
Modern data processing technology history
The term “data processing” is generally used for the first stage, followed by data analysis in the second stage of overall data handling.
The data analysis process is considerably more complicated and technical than it appears. Data analysis employs specialist algorithms and statistical calculations less common in a normal business environment.
SPSS, SAS, or their free counterparts DAP, Gretl, or PSPP are popular for data analysis software suites.