Meet the best free LLMs on the market: LLama-2, GPT-3.5, and PaLM 2. Step into unparalleled linguistic mastery and AI brilliance without spending a dime!
LLama-2, GPT-3.5, and PaLM 2 are the vanguards of natural language processing, offering an extraordinary feat, and they’re free for personal usage! These remarkable language models have broken barriers, not just in their capabilities but also in accessibility. As we delve into the arena of free LLMs (language learning models), prepare to witness the astonishing power of these cutting-edge giants, revolutionizing communication and innovation without costing a cent.
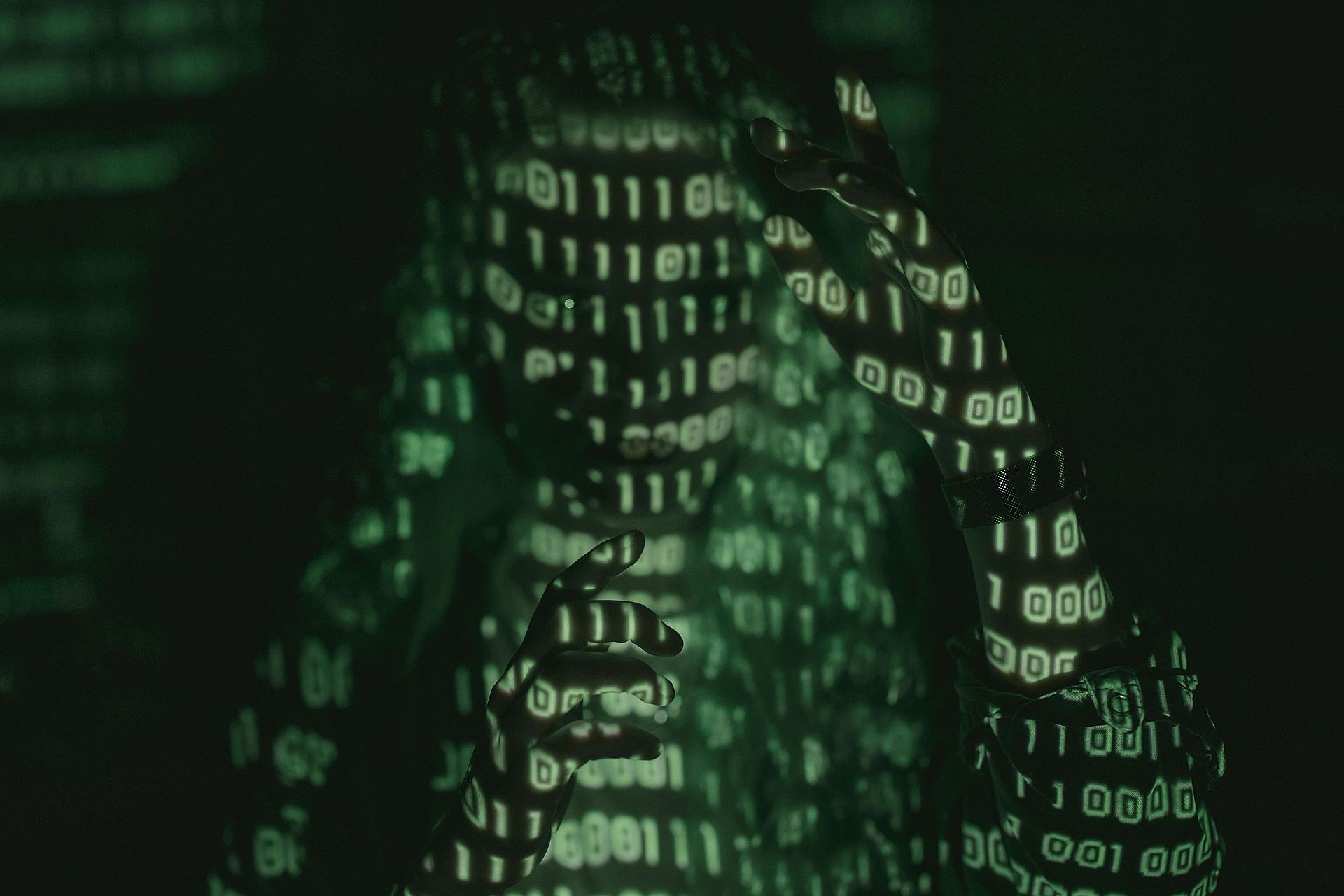
Best free LLMs: LLama-2 vs GPT-3.5 vs PaLM 2
The landscape of large language models (LLMs) is dominated by the triumvirate of Meta’s LLama-2, OpenAI’s GPT-3.5, and Google’s PaLM-2, each wielding its own distinct capabilities and intricacies. So, which one is better? Let’s dig deeper and find out!
Parameter Size: The tug of scale
The bedrock of an LLM’s capabilities often lies in its parameter size, dictating the model’s depth and complexity.
LLama-2 (70 billion parameters)
- Advantages: Efficient and cost-effective, excelling in tasks prioritizing affordability and swiftness. Its modest parameter size maintains a balance between capability and accessibility.
- Limitations: Smaller parameter size may limit its depth of understanding, impacting performance in tasks requiring intricate language comprehension.
GPT-3.5 (175 billion parameters)
- Advantages: Strikes a balance between scale and capability. Its larger parameter size enables nuanced language understanding across various tasks without compromising efficiency or accessibility significantly.
- Limitations: Falls short in scale compared to PaLM-2, potentially limiting performance in tasks demanding extensive information processing.
PaLM-2 (540 billion parameters)
- Advantages: Boasts unparalleled complexity, excelling in understanding intricate language nuances. Ideal for tasks requiring comprehensive language processing.
- Limitations: Demands substantial resources and investment, limiting practicality for resource-constrained applications.
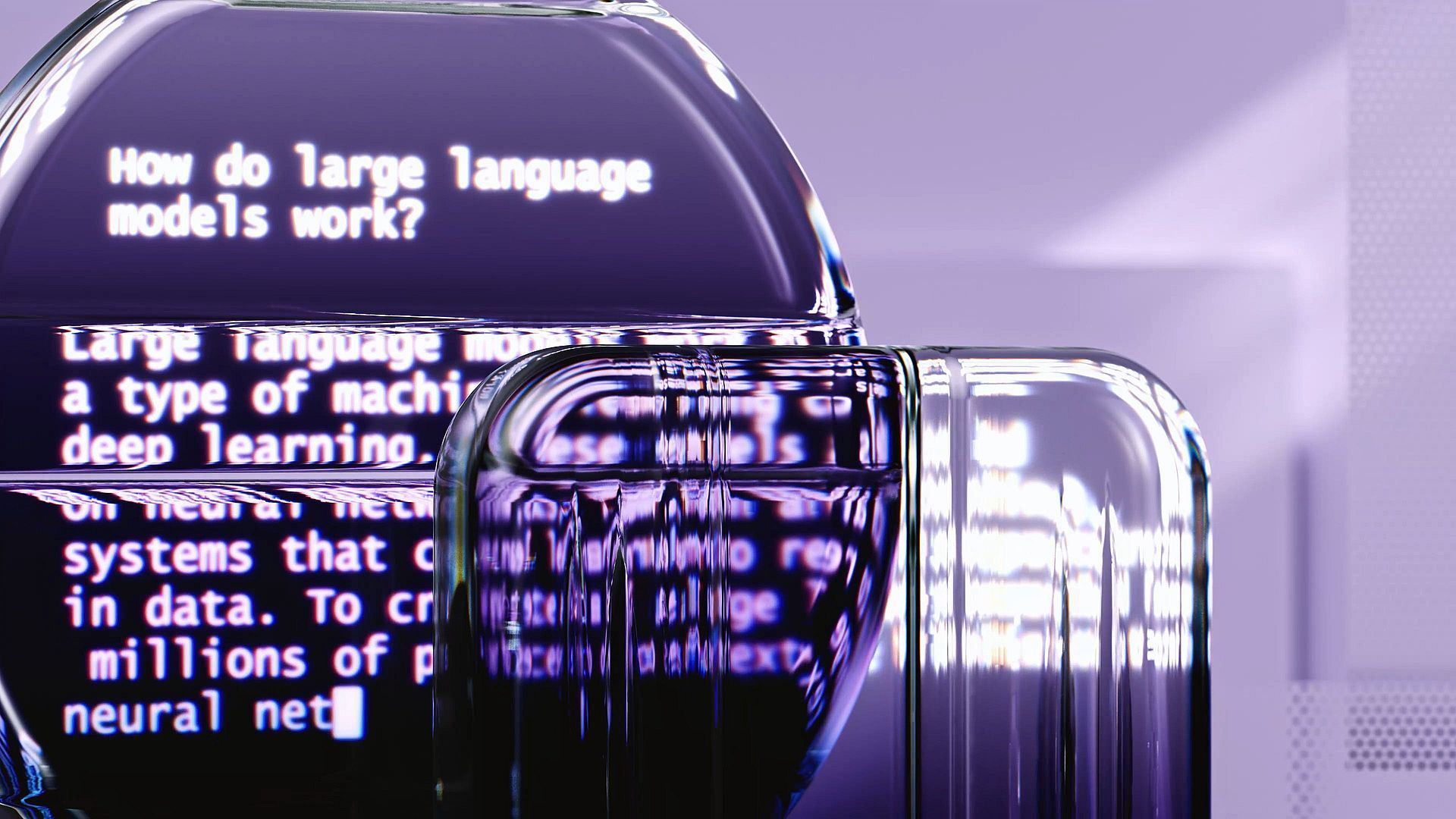
Verdict
Choosing the “better” model hinges on task-specific needs. LLama-2 prioritizes efficiency and affordability, GPT-3.5 balances capability and accessibility, while PaLM-2 excels in intricate language comprehension but demands substantial resources. However, PaLM-2, with its 540 billion parameters, won this round.
Accuracy
When it comes to accuracy among LLama-2, GPT-3.5, and PaLM-2, each model showcases a distinct level of precision, influenced by their parameter size, training data, and architectural intricacies.
- LLama-2: Despite its smaller parameter size of 70 billion, LLama-2 demonstrates high accuracy. It’s capable of generating precise and grammatically correct text, showcasing reliability in content creation and language processing tasks.
- GPT-3.5: OpenAI’s GPT-3.5, with a larger parameter size of 175 billion, maintains a similar level of high accuracy. Its robust architecture allows for contextually relevant and grammatically sound text generation, ensuring reliability across various applications.
- PaLM-2: Google’s PaLM-2, standing at a monumental 540 billion parameters, boasts very high accuracy. Leveraging its extensive parameter size, PaLM-2 dives deeper into language nuances, showcasing an unparalleled understanding and precision in text generation tasks.
Verdict
The scale of parameters isn’t the sole determinant of an LLM’s performance. Based on our personal usage, GPT-3.5 emerged victorious in this round.
Efficiency
Efficiency in Large Language Models (LLMs) is a crucial metric, encompassing both speed and computational resource utilization
- LLama-2: Leads in efficiency with swift performance and optimized resource usage due to its tailored 70 billion parameters, making it a proficient and cost-effective choice.
- GPT-3.5: Moderately efficient, balancing capability and resource utilization with 175 billion parameters. It offers commendable performance across tasks but lags slightly behind LLama-2 in speed and resource efficiency.
- PaLM-2: Sacrifices efficiency for capability with its extensive 540 billion parameters, demanding significantly higher computational resources. Its slower processing speed limits its efficiency compared to LLama-2 and GPT-3.5.
Verdict
LLama-2 emerges as the efficiency champion, offering a balanced blend of speed and resource optimization. GPT-3.5 follows with moderate efficiency, while PaLM-2’s resource-intensive operations hinder its efficiency in practical applications.
Sum up
For affordability and general tasks, the LLama-2, with its efficient size and commendable accuracy, stands out. It strikes a balance between capability and accessibility, making it a prudent choice for basic to moderately complex tasks without demanding excessive resources.
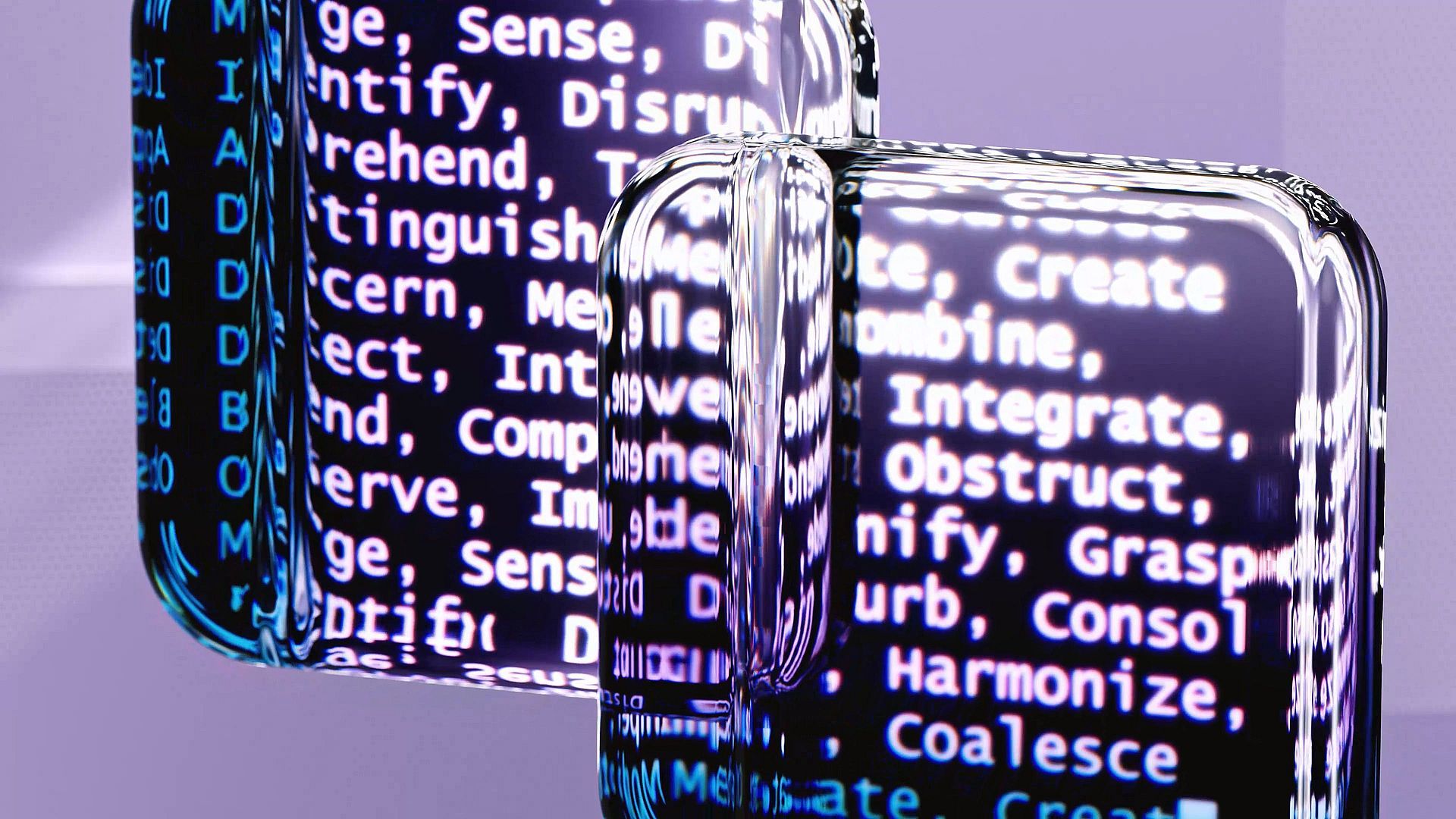
When comprehensive understanding matters, PaLM-2 shines with its expansive parameter size, enabling an unparalleled depth of language comprehension. It excels in tasks that demand intricate language nuances but might be overkill for resource-constrained applications.
Overall performance: GPT-3.5 emerges as a versatile choice. While it may not boast the largest parameter size like PaLM-2, it strikes a commendable balance between capability, accessibility, and efficiency, making it a strong all-rounder for various tasks.
In essence, the best choice among LLama-2, GPT-3.5, and PaLM 2 boils down to the specific requirements of the task at hand. There’s no universal “best” model; instead, each offers a tailored solution catering to different needs, from affordability to comprehensive language processing.
Featured image credit: ThisIsEngineering/Pexels