At the last AI Conference, we had a chance to sit down with Roman Shaposhnik and Tanya Dadasheva, the co-founders of Ainekko/AIFoundry, and discuss with them an ambiguous topic of data value for enterprises in the times of AI. One of the key questions we started from was: are most companies running the same frontier AI models, is incorporating their data the only way they have a chance to differentiate? Is data really a moat for enterprises?
Roman recalls: “Back in 2009, when he started in the big data community, everyone talked about how enterprises would transform by leveraging data. At that time, they weren’t even digital enterprises; the digital transformation hadn’t occurred yet. These were mostly analog enterprises, but they were already emphasizing the value of the data they collected—data about their customers, transactions, supply chains, and more. People likened data to oil, something with inherent value that needed to be extracted to realize its true potential.”
However, oil is a commodity. So, if we compare data to oil, it suggests everyone has access to the same data, though in different quantities and easier to harvest for some. This comparison makes data feel like a commodity, available to everyone but processed in different ways.
When data sits in an enterprise data warehouse in its crude form, it’s like an amorphous blob—a commodity that everyone has. However, once you start refining it, that’s when the real value comes in. It’s not just about acquiring data but building a process from extraction to refining all the value through the pipeline.
“Interestingly, this reminds me of something an oil corporation executive once told me” – shares Roman. “That executive described the business not as extracting oil but as reconfiguring carbon molecules. Oil, for them, was merely a source of carbon. They had built supply chains capable of reconfiguring these carbon molecules into products tailored to market demands in different locations—plastics, gasoline, whatever the need was. He envisioned software-defined refineries that could adapt outputs based on real-time market needs. This concept blew my mind, and I think it parallels what we’re seeing in data now—bringing compute to data, refining it to get what you need, where you need it” – was Roman’s insight.
In enterprises, when you start collecting data, you realize it’s fragmented and in many places—sometimes stuck in mainframes or scattered across systems like Salesforce. Even if you manage to collect it, there are so many silos, and we need a fracking-like approach to extract the valuable parts. Just as fracking extracts oil from places previously unreachable, we need methods to get enterprise data that is otherwise locked away.
A lot of enterprise data still resides in mainframes, and getting it out is challenging. Here’s a fun fact: with high probability, if you book a flight today, the backend still hits a mainframe. It’s not just about extracting that data once; you need continuous access to it. Many companies are making a business out of helping enterprises get data out of old systems, and tools like Apache Airflow are helping streamline these processes.
But even if data is no longer stuck in mainframes, it’s still fragmented across systems like cloud SaaS services or data lakes. This means enterprises don’t have all their data in one place, and it’s certainly not as accessible or timely as they need. You might think that starting from scratch would give you an advantage, but even newer systems depend on multiple partners, and those partners control parts of the data you need.
The whole notion of data as a moat turns out to be misleading then. Conceptually, enterprises own their data, but they often lack real access. For instance, an enterprise using Salesforce owns the data, but the actual control and access to that data are limited by Salesforce. The distinction between owning and having data is significant.
“Things get even more complicated when AI starts getting involved” – says Tanya Dadasheva, another co-founder of AInekko and AIFoundry.org. “An enterprise might own data, but it doesn’t necessarily mean a company like Salesforce can use it to train models. There’s also the debate about whether anonymized data can be used for training—legally, it’s a gray area. In general, the more data is anonymized, the less value it holds. At some point, getting explicit permission becomes the only way forward”.
This ownership issue extends beyond enterprises; it also affects end-users. Users often agree to share data, but they may not agree to have it used for training models. There have been cases of reverse-engineering data from models, leading to potential breaches of privacy.
At an early stage of balancing data producers, data consumers, and the entities that refine data, legally and technologically it is extremely complex figuring out how these relationships will work. Europe, for example, has much stricter privacy rules compared to the United States (https://artificialintelligenceact.eu/). In the U.S., the legal system often figures things out on the go, whereas Europe prefers to establish laws in advance.
Tanya addresses data availability here: “This all ties back to the value of data available. The massive language models we’ve built have grown impressive thanks to public and semi-public data. However, much of the newer content is now trapped in “walled gardens” like WeChat, Telegram or Discord, where it’s inaccessible for training – true dark web! This means the models may become outdated, unable to learn from new data or understand new trends.
In the end, we risk creating models that are stuck in the past, with no way to absorb new information or adapt to new conversational styles. They’ll still contain older data, and the newer generation’s behavior and culture won’t be represented. It’ll be like talking to a grandparent—interesting, but definitely from another era.”
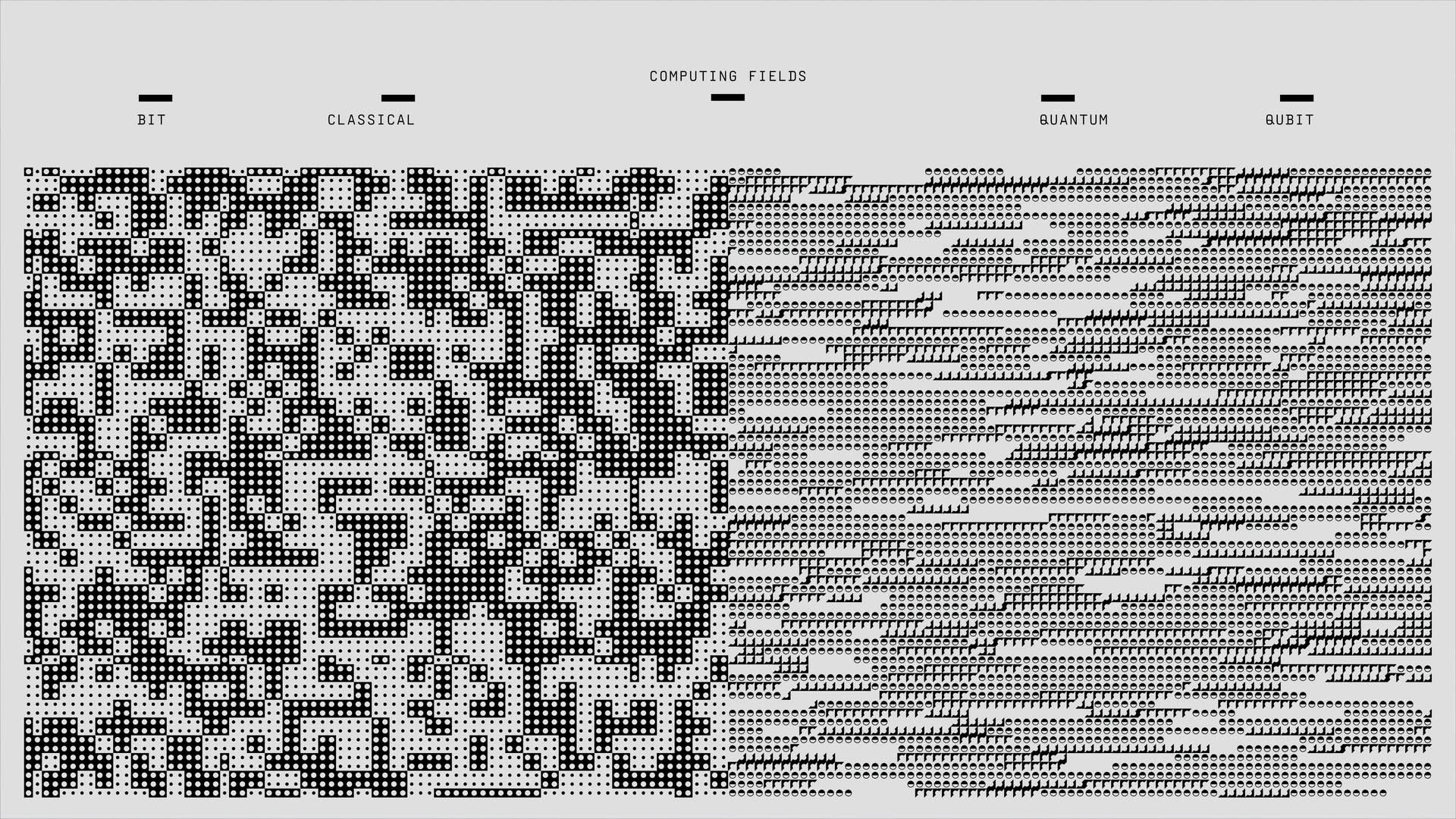
But who are the internal users of the data in an enterprise? Roman recalls the three epochs of data utilization concept within the enterprises: “Obviously, it’s used for many decisions, which is why the whole business intelligence part exists. It all actually started with business intelligence. Corporations had to make predictions and signal to the stock markets what they expect to happen in the next quarter or a few quarters ahead. Many of those decisions have been data-driven for a long time. That’s the first level of data usage—very straightforward and business-oriented.
The second level kicked in with the notion of digitally defined enterprises or digital transformation. Companies realized that the way they interact with their customers is what’s valuable, not necessarily the actual product they’re selling at the moment. The relationship with the customer is the value in and of itself. They wanted that relationship to last as long as possible, sometimes to the extreme of keeping you glued to the screen for as long as possible. It’s about shaping the behavior of the consumer and making them do certain things. That can only be done by analyzing many different things about you—your social and economic status, your gender identity, and other data points that allow them to keep that relationship going for as long as they can.
Now, we come to the third level or third stage of how enterprises can benefit from data products. Everybody is talking about these agentic systems because enterprises now want to be helped not just by the human workforce. Although it sounds futuristic, it’s often as simple as figuring out when a meeting is supposed to happen. We’ve always been in situations where it takes five different emails and three calls to figure out how two people can meet for lunch. It would be much easier if an electronic agent could negotiate all that for us and help with that. That’s a simple example, but enterprises have all sorts of others. Now it’s about externalizing certain sides of the enterprise into these agents. That can only be done if you can train an AI agent on many types of patterns that the enterprise has engaged in the past.”
Getting back to who collects and who owns and, eventually, benefits from data: the first glimpse of that Roman got when working back at Pivotal on a few projects that involved airlines and companies that manufacture engines:
“What I didn’t know at the time is that apparently you don’t actually buy the engine; you lease the engine. That’s the business model. And the companies producing the engines had all this data—all the telemetry they needed to optimize the engine. But then the airline was like, “Wait a minute. That is exactly the same data that we need to optimize the flight routes. And we are the ones collecting that data for you because we actually fly the plane. Your engine stays on the ground until there’s a pilot in the cockpit that actually flies the plane. So who gets to profit from the data? We’re already paying way too much to engine people to maintain those engines. So now you’re telling us that we’ll be giving you the data for free? No, no, no.”
This whole argument is really compelling because that’s exactly what is now repeating itself between OpenAI and all of the big enterprises. Big enterprises think OpenAI is awesome; they can build this chatbot in minutes—this is great. But can they actually send that data to OpenAI that is required for fine-tuning and all these other things? And second of all, suppose those companies even can. Suppose it’s the kind of data that’s fine, but it’s their data – collected by those companies. Surely it’s worth something to OpenAI, so why don’t they drop the bill on the inference side for companies who collected it?
And here the main question of today’s data world kicks in: Is it the same with AI?
In some way, it is, but with important nuances. If we can have a future where the core ‘engine’ of an airplane, the model, gets produced by these bigger companies, and then enterprises leverage their data to fine-tune or augment these models, then there will be a very harmonious coexistence of a really complex thing and a more highly specialized, maybe less complex thing on top of it. If that happens and becomes successful technologically, then it will be a much easier conversation at the economics and policy level of what belongs to whom and how we split the data sets.
As an example, Roman quotes his conversation with an expert who designs cars for a living: “He said that there are basically two types of car designers: one who designs a car for an engine, and the other one who designs a car and then shops for an engine. If you’re producing a car today, it’s much easier to get the engine because the engine is the most complex part of the car. However, it definitely doesn’t define the product. But still, the way that the industry works: it’s much easier to say, well, given some constraints, I’m picking an engine, and then I’m designing a whole lineup of cars around that engine or that engine type at least.”
This drives us to the following concept: we believe that’s what the AI-driven data world will look like. There will be ‘Google’ camp and ‘Meta camp’, and you will pick one of those open models – all of them will be good enough. And then, all of the stuff that you as an enterprise are interested in, is built on top of it in terms of applying your data and your know-how of how to fine-tune them and continuously update those models from different ‘camps’. In case this works out technologically and economically, a brave new world will emerge.