During its GTC conference, NVIDIA unveiled NVIDIA NIM, a software platform engineered to simplify the integration of both custom and pre-trained AI models into operational settings. NIM leverages NVIDIA’s expertise in model inferencing and optimization, offering an accessible approach by merging any selected model with a finely-tuned inferencing engine and encapsulating this combination within a container, subsequently providing it as a microservice.
NVIDIA contends that what would ordinarily require developers several weeks to months to accomplish in terms of container deployment can be expedited through NIM, especially in scenarios where a company may lack in-house AI expertise. NVIDIA’s strategic goal with NIM is to foster a network of AI-prepared containers that are built upon its hardware infrastructure, with these specialized microservices acting as the principal software component for organizations eager to accelerate their AI initiatives.
Currently, NIM extends support to models originating from NVIDIA, A121, Adept, Cohere, Getty Images, and Shutterstock, in addition to open-source models by Google, Hugging Face, Meta, Microsoft, Mistral AI, and Stability AI. NVIDIA is actively collaborating with Amazon, Google, and Microsoft to make NIM microservices accessible through SageMaker, Kubernetes Engine, and Azure AI, correspondingly. These services are poised to be incorporated into platforms like Deepset, LangChain, and LlamaIndex.
“We believe that the NVIDIA GPU is the best place to run inference of these models on […], and we believe that NVIDIA NIM is the best software package, the best runtime, for developers to build on top of so that they can focus on the enterprise applications” Manuvir Das, NVIDIA’s head of enterprise computing, expressed during a press briefing prior to today’s announcements.
Regarding the inferencing engine, NVIDIA plans to implement the Triton Inference Server, alongside TensorRT and TensorRT-LLM for its operations. Among the offerings NVIDIA provides via NIM are Riva, designed for tailoring speech and translation models, cuOpt for enhancing routing processes, and the Earth-2 model, developed for advanced weather and climate forecasting simulations.
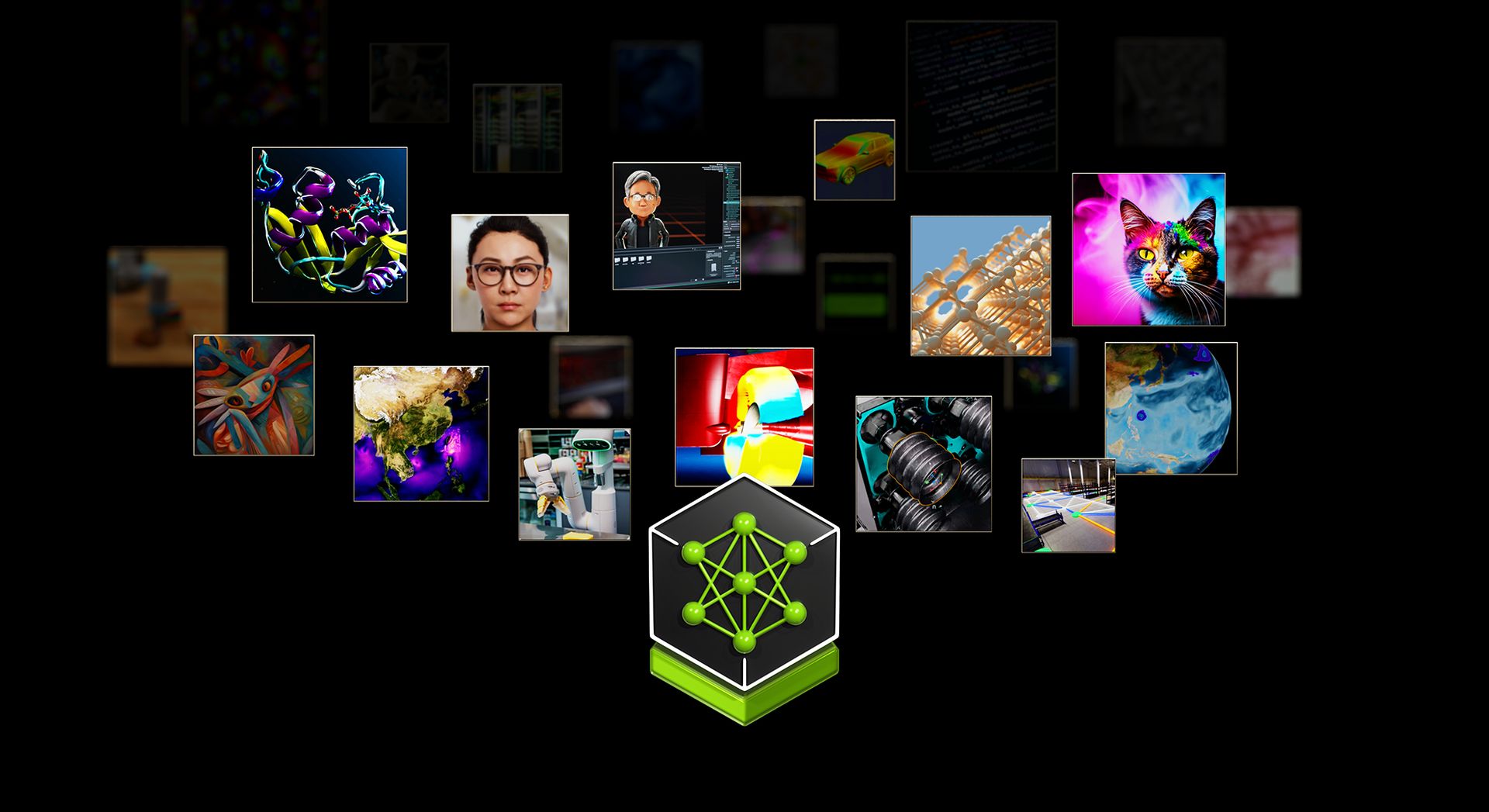
NVIDIA is committed to broadening its suite of services, introducing new features progressively. An upcoming addition is the NVIDIA RAG LLM operator as a NIM service, aimed at simplifying the creation of generative AI chatbots capable of incorporating customized data, significantly easing the development process.
Highlighting the importance of community and partnerships, the conference also spotlighted engagements with leading companies such as Box, Cloudera, Cohesity, Datastax, Dropbox, and NetApp currently utilizing NIM services.
“Established enterprise platforms are sitting on a goldmine of data that can be transformed into generative AI copilots. Created with our partner ecosystem, these containerized AI microservices are the building blocks for enterprises in every industry to become AI companies,” stated Jensen Huang, the CEO of NVIDIA.
What does NVDIA NIM really do?
Fundamentally, an NIM constitutes a container filled with microservices. This container is capable of incorporating any model type, from open-source to proprietary, provided it operates on an NVIDIA GPU—whether that’s hosted in the cloud or simply within a laptop. Consequently, the container can be deployed across any environment supportive of containers, including Kubernetes setups in the cloud, Linux servers, or even within serverless Function-as-a-Service frameworks. NVIDIA is set to introduce a serverless function feature on its forthcoming ai.nvidia.com portal, offering developers an avenue to engage with NIM before its deployment.
It’s important to note, NIM doesn’t aim to supplant any of NVIDIA’s previous model delivery methodologies. Instead, it’s a specialized container that bundles a highly refined model tailored for NVIDIA GPUs, along with the essential technologies to enhance inference performance.
The pressing question concerns the transition to production. How can the initial prototypes, developed with our assistance, be advanced to deliver tangible business outcomes through production deployment using these models? NVIDIA, along with a consortium of leading data providers, views NIM as a solution to this dilemma. Vector database functionality is pivotal for activating RAG, supported by various vector database providers, including Apache Lucene, Datastax, Faiss, Kinetica, Milvus, Redis, and Weaviate.
Featured image credit: Kerem Gülen/DALL-E 3