The emergence of the Internet of Things (IoT) has led to the proliferation of connected devices and sensors that generate vast amounts of data. This data is a goldmine of insights that can be harnessed to optimize various systems and processes. However, to unlock the full potential of IoT data, organizations need to leverage the power of data science. Data science can help organizations derive valuable insights from IoT data and make data-driven decisions to optimize their operations.
Coherence between IoT and data science is critical to ensure that organizations can maximize the value of their IoT ecosystems. It requires a deep understanding of the interplay between IoT devices, sensors, networks, and data science tools and techniques. Organizations that can effectively integrate IoT and data science can derive significant benefits, such as improved efficiency, reduced costs, and enhanced customer experiences.
What is an IoT ecosystem?
An IoT (Internet of Things) ecosystem refers to a network of interconnected devices, sensors, and software applications that work together to collect, analyze, and share data. The ecosystem consists of various components, including devices, communication networks, data storage, and analytics tools, that work together to create an intelligent system that enables automation, monitoring, and control of various processes.
IoT protocols 101: The essential guide to choosing the right option
Some key characteristics of an IoT ecosystem include the following:
- Interconnectivity: IoT devices and applications are connected and communicate with each other to share data and enable coordinated actions.
- Data-driven: The ecosystem is built around data, and devices generate and share data that is used to enable automation, predictive maintenance, and other applications.
- Scalable: IoT ecosystems can be scaled up or down depending on the number of devices and the amount of data being generated.
- Intelligent: The ecosystem uses AI and machine learning algorithms to analyze data and derive insights that can be used to optimize processes and drive efficiencies.
What is an IoT ecosystem diagram?
An IoT ecosystem diagram is a visual representation of the components and relationships that make up an IoT ecosystem. It typically includes devices, communication networks, data storage, and analytics tools that work together to create an intelligent system.
The diagram provides a high-level overview of the ecosystem and helps to visualize the various components and how they are interconnected. It can also be used to identify potential areas for improvement and optimization within the system.
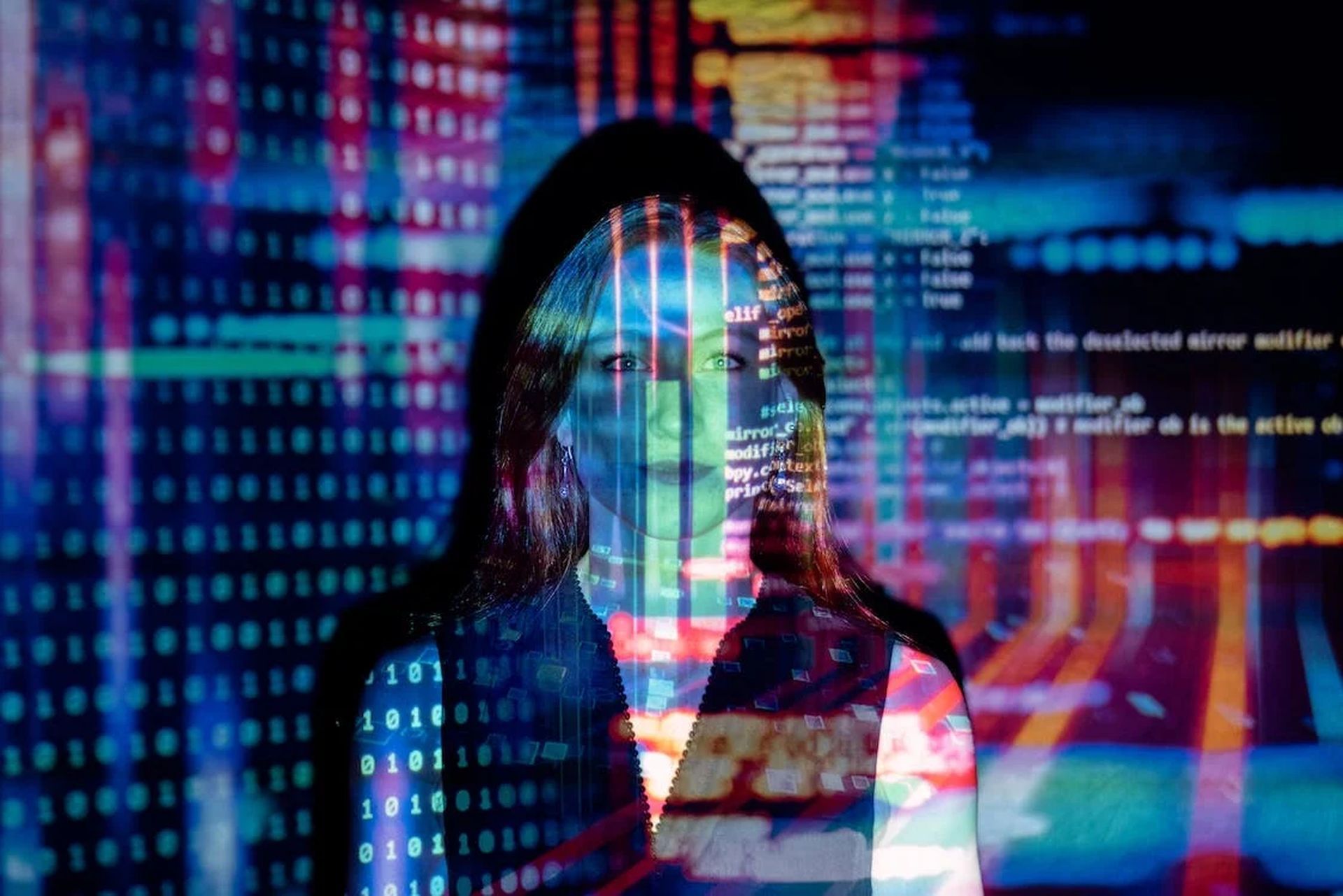
Understanding IoT ecosystem architecture
IoT ecosystem architecture refers to the design and structure of an IoT system, including the various components and how they are connected.
There are several layers to an IoT ecosystem architecture, including:
- Device layer: This layer includes the sensors and other devices that collect data and interact with the physical environment.
- Communication layer: This layer includes the communication networks that enable data to be transmitted between devices and other components.
- Data layer: This layer includes the data storage and management systems that store and process the data generated by the IoT system.
- Application layer: This layer includes software applications and tools that enable users to interact with and make sense of the data generated by the system.
Defining IoT ecosystems and their role in data science
IoT ecosystems play an important role in data science, as they generate vast amounts of data that can be used to drive insights and optimize processes.
Some ways that IoT ecosystems contribute to data science include:
- Enabling data collection: IoT devices generate large amounts of data that can be used to train machine learning algorithms and drive predictive models.
- Providing real-time data: IoT ecosystems can provide real-time data that can be used to identify trends and patterns and drive immediate action.
- Facilitating automation: IoT ecosystems can be used to automate various processes, reducing the need for manual intervention and enabling greater efficiency.
IoT ecosystems provide a rich source of data that can be used to drive insights and optimize processes, making them a valuable tool in the data science toolkit.
Components of IoT ecosystems
IoT ecosystems are composed of various components that work together to collect, process, and transmit data.
Component | Description |
Sensors | IoT sensors collect data from the physical environment. |
Connectivity | IoT connectivity enables the transfer of data between devices and networks. |
Cloud Platform | IoT cloud platforms enable data storage, processing, and analysis in the cloud. |
Edge Computing | IoT edge computing involves processing data closer to the source, reducing latency and improving performance. |
Applications | IoT applications provide users with a way to interact with IoT data and devices. |
Analytics | IoT analytics involves using data science techniques to derive insights from IoT data. |
Hardware and software components of IoT ecosystems
IoT ecosystems consist of both hardware and software components that work together to enable automation, monitoring, and control of various processes. Some of the key hardware and software components of IoT ecosystems include:
- Hardware components: IoT hardware components include devices and sensors, communication networks, and data storage systems. These components are responsible for collecting, transmitting, and processing data.
- Software components: IoT software components include applications, operating systems, and analytics tools. These components are responsible for processing and analyzing the data generated by IoT devices and sensors.
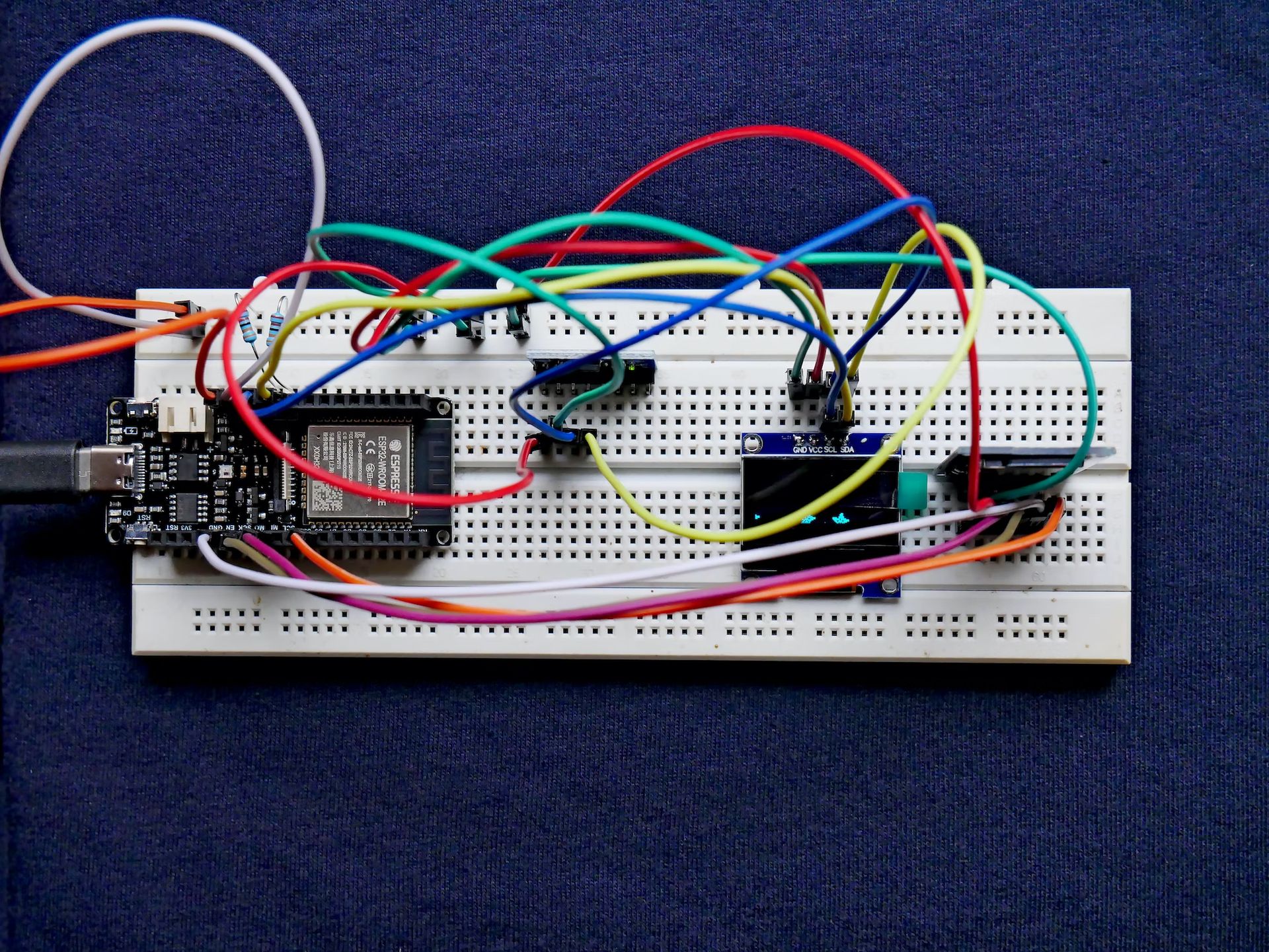
Understanding the role of each component in IoT ecosystems
Each component in an IoT ecosystem plays a critical role in enabling the system to function effectively. Understanding the role of each component is essential in designing and optimizing IoT ecosystems. Some of the key roles of each component in IoT ecosystems include:
- Sensors and devices: IoT sensors and devices are responsible for collecting data from the physical environment. They play a critical role in enabling automation, monitoring, and control of various processes.
- Communication networks: Communication networks enable the transmission of data between IoT devices and other components in the ecosystem. They are responsible for ensuring that data is transmitted securely and reliably.
- Data storage: Data storage is essential in IoT ecosystems, as it is responsible for storing and managing the vast amounts of data generated by IoT devices and sensors. Data storage solutions need to be scalable, secure, and cost-effective.
- Analytics tools: Analytics tools are used to process and analyze the data generated by IoT devices and sensors. They play a critical role in enabling data-driven decision-making and identifying trends and patterns.
Importance of choosing the right components for IoT ecosystems
Choosing the right components for IoT ecosystems is essential in ensuring that the system functions effectively and efficiently. Some of the key reasons why choosing the right components is important to include:
- Scalability: IoT ecosystems need to be scalable, and choosing the right components can ensure that the system can be scaled up or down as needed.
- Reliability: IoT ecosystems need to be reliable, and choosing the right components can ensure that the system is resilient and can operate under various conditions.
- Security: IoT ecosystems need to be secure, and choosing the right components can ensure that data is transmitted and stored securely.
Challenges in designing IoT ecosystems
Designing and implementing IoT ecosystems can be challenging due to various factors, such as the complexity of the system, the diversity of devices, and the need for interoperability. Some of the common challenges in designing and implementing IoT ecosystems include the following:
- Data management: The vast amount of data generated by IoT devices can be overwhelming, making it challenging to store, process, and analyze the data effectively.
- Interoperability: IoT devices and sensors may come from different manufacturers, making it challenging to ensure that they are compatible and can communicate with each other.
- Security: IoT ecosystems are vulnerable to security threats, such as data breaches, hacking, and cyber attacks, making it essential to implement robust security measures.
- Scalability: As the number of devices in an IoT ecosystem increases, the system needs to be scalable and able to handle the increasing volume of data and traffic.
- Lack of standards: The lack of industry-wide standards makes it challenging to ensure that IoT devices and sensors are interoperable and can communicate with each other.
- Data security: IoT ecosystems are vulnerable to security threats, and organizations need to implement robust security measures to protect sensitive data.
- Data management: The vast amount of data generated by IoT devices can be challenging to store, process, and analyze effectively, making it essential to implement effective data management strategies.
- Integration with legacy systems: Integrating IoT ecosystems with legacy systems can be challenging, and organizations need to ensure that the systems are compatible and can work together seamlessly.
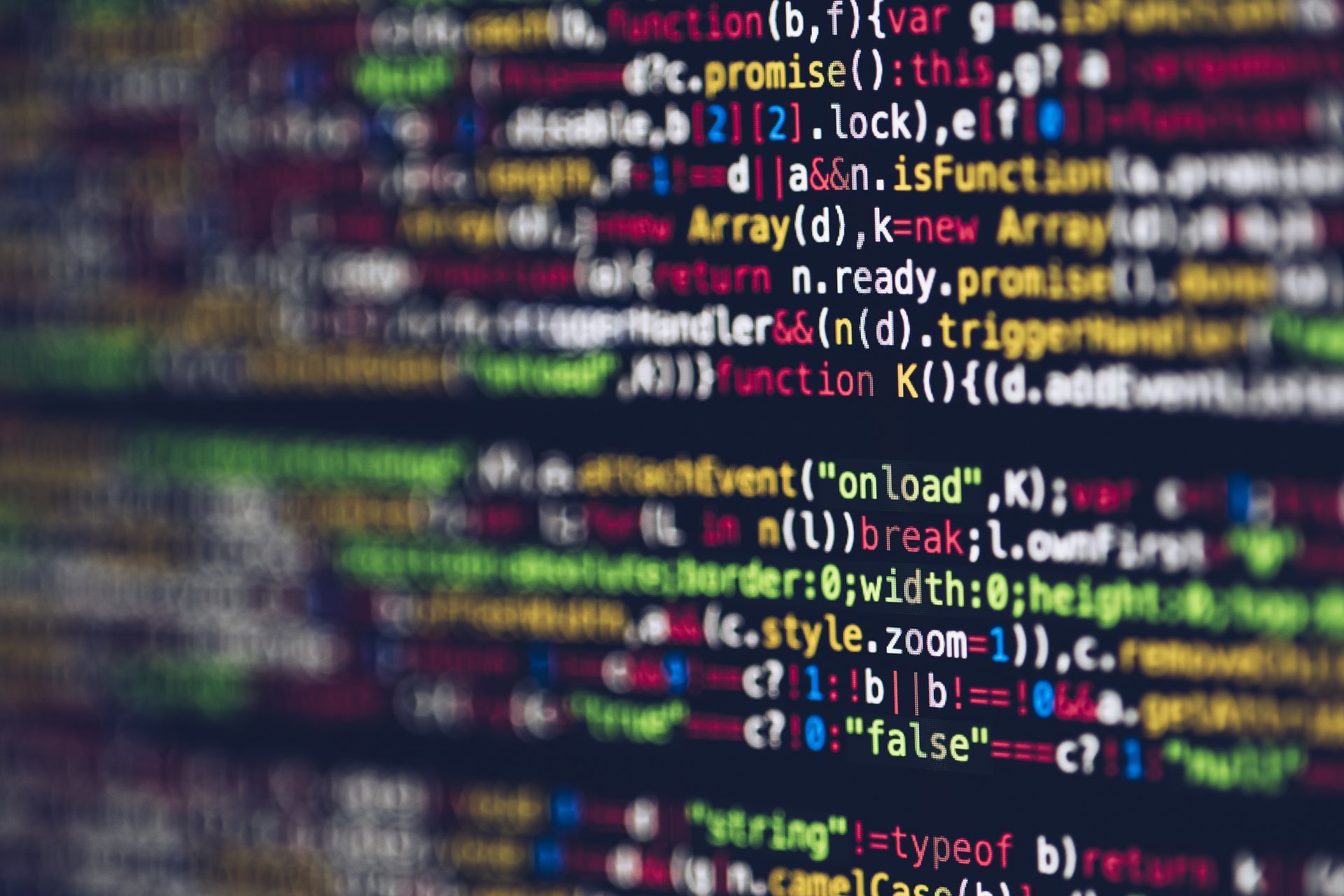
Solutions for overcoming IoT ecosystem design and implementation challenges
Overcoming the challenges of designing and implementing IoT ecosystems requires a combination of technical expertise, strategic planning, and effective execution. Some of the solutions for overcoming IoT ecosystem design and implementation challenges include:
- Adopting standards: Adhering to industry-wide standards can help ensure that IoT devices and sensors are interoperable and can communicate with each other.
- Implementing robust security measures: Implementing robust security measures, such as encryption, firewalls, and intrusion detection systems, can help protect sensitive data.
- Leveraging cloud computing: Cloud computing can provide scalable and cost-effective data storage and processing solutions for IoT ecosystems.
- Implementing effective data management strategies: Implementing effective data management strategies, such as data analytics and visualization tools, can help organizations derive insights from the vast amounts of data generated by IoT devices.
Best practices for designing IoT ecosystems for data science
Designing IoT ecosystems for data science requires careful planning and execution. Some of the best practices for designing IoT ecosystems for data science include:
- Identifying use cases: Identifying use cases and defining clear objectives can help organizations design IoT ecosystems that meet specific business needs.
- Choosing the right components: Choosing the right components, such as sensors, communication networks, data storage, and analytics tools, is critical in ensuring that the system is effective and efficient.
- Ensuring interoperability: Ensuring that IoT devices and sensors are interoperable and can communicate with each other is essential in enabling data-driven decision-making.
- Implementing effective data management strategies: Implementing effective data management strategies, such as data analytics and visualization tools, can help organizations derive insights from the vast amounts of data generated by IoT devices.
Designing IoT ecosystems for data science requires a combination of technical expertise, strategic planning, and effective execution, and organizations need to adopt best practices to ensure success.
IoT and machine learning: Walking hand in hand towards smarter future
The role of data science in optimizing IoT ecosystems
Data science plays a critical role in optimizing IoT ecosystems by enabling organizations to derive insights from the vast amounts of data generated by IoT devices and sensors. Data science can help organizations identify trends and patterns, predict future events, and optimize processes.
Some of the key ways that data science can be used to optimize IoT ecosystems include:
- Predictive maintenance: Data science can be used to predict when equipment is likely to fail, enabling organizations to schedule maintenance proactively and avoid costly downtime.
- Optimization: Data science can be used to optimize processes, such as supply chain management, inventory management, and production scheduling, enabling organizations to operate more efficiently.
- Personalization: Data science can be used to personalize products and services, enabling organizations to deliver better customer experiences.
Leveraging data science to optimize IoT ecosystem performance
Leveraging data science to optimize IoT ecosystem performance requires a combination of technical expertise, strategic planning, and effective execution. Some of the key steps involved in leveraging data science to optimize IoT ecosystem performance include:
- Data collection: Collecting data from IoT devices and sensors is the first step in leveraging data science to optimize IoT ecosystem performance.
- Data management: Managing the vast amounts of data generated by IoT devices and sensors requires effective data management strategies, such as data cleansing, data normalization, and data modeling.
- Data analysis: Analyzing the data generated by IoT devices and sensors requires advanced analytics tools, such as machine learning algorithms and artificial intelligence.
- Insights and action: Deriving insights from the data generated by IoT devices and sensors is only useful if organizations can take action based on those insights. This requires effective communication, collaboration, and execution.
IoT ecosystem examples
There are several examples of data science applications in IoT ecosystems. Some of the key examples include:
- Predictive maintenance: Data science can be used to predict when equipment is likely to fail, enabling organizations to schedule maintenance proactively and avoid costly downtime. For example, General Electric uses data science to predict when its engines are likely to fail and schedule maintenance accordingly.
- Optimization: Data science can be used to optimize processes, such as supply chain management, inventory management, and production scheduling, enabling organizations to operate more efficiently. For example, Walmart uses data science to optimize its supply chain and reduce costs.
- Personalization: Data science can be used to personalize products and services, enabling organizations to deliver better customer experiences. For example, Amazon uses data science to personalize its recommendations for customers based on their browsing and purchase history.
Security and privacy concerns in IoT ecosystems
IoT ecosystems pose significant security and privacy challenges due to the sheer volume of data generated by numerous devices and sensors. The data can include highly sensitive information, such as biometric data, personal information, and financial details, making it critical to ensure that it is secured and protected.
One of the significant concerns is device security, where the devices are vulnerable to hacking, compromising their integrity and privacy. Network security is also a concern, where the data transmitted over the networks may be intercepted and compromised. Data privacy is another critical concern where there is a risk of unauthorized access to the vast amounts of sensitive data generated by IoT devices.
Devices and sensors are vulnerable to various types of attacks, including malware, distributed denial-of-service (DDoS) attacks, and phishing scams. These attacks can compromise the security of the devices and data generated, leading to devastating consequences.
Data breaches are another concern where the vast amounts of data generated by IoT devices need to be stored and transmitted securely. Any breach of the data can expose sensitive information, leading to privacy violations, identity theft, and other serious consequences.
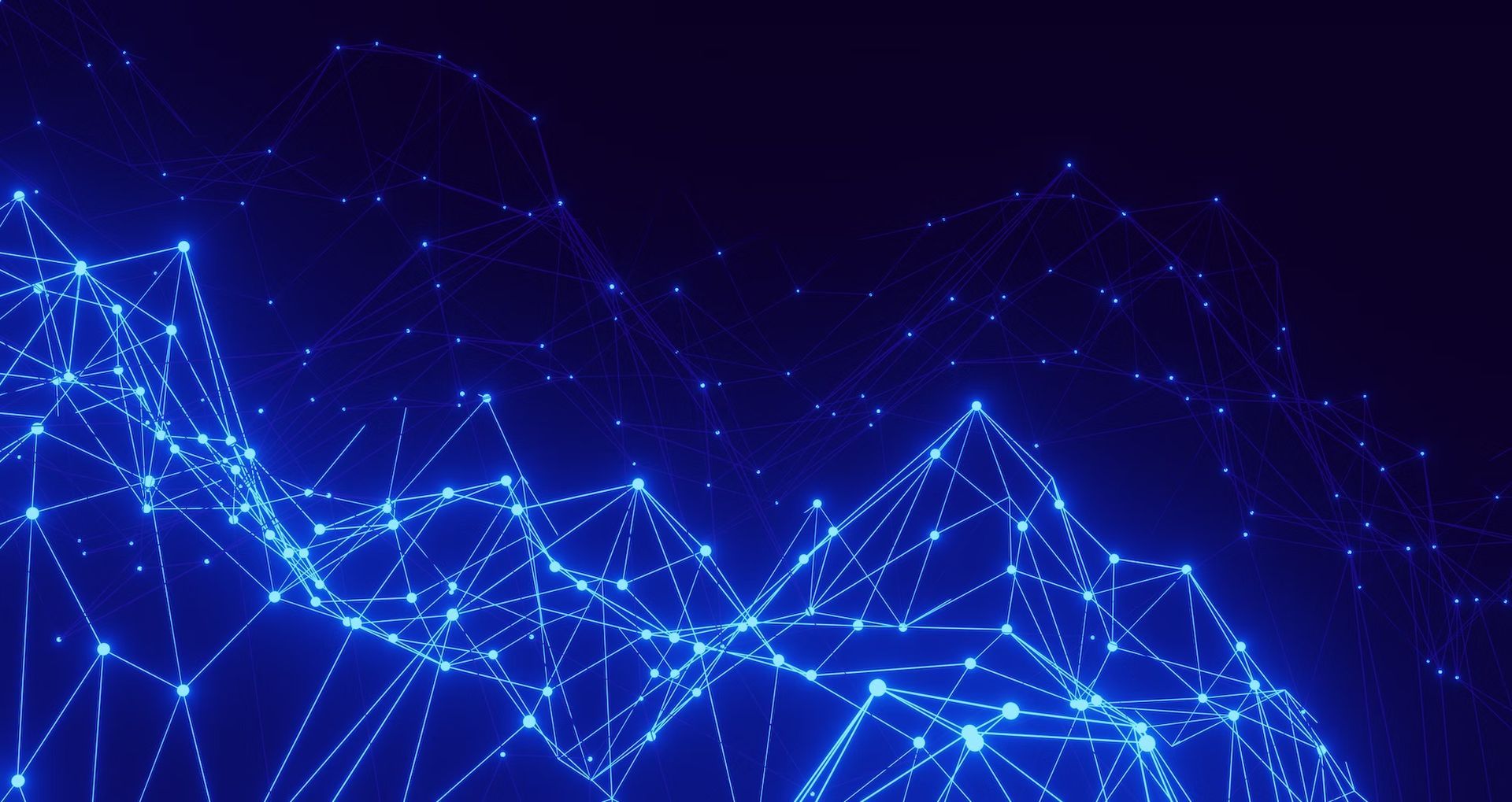
Impact of security and privacy concerns on data science in IoT ecosystems
Security and privacy concerns can have a significant impact on data science in IoT ecosystems. Data quality can be compromised due to security and privacy concerns, leading to incomplete or inaccurate data that can affect the effectiveness of data science. The volume of data that is available for analysis may also be limited due to security and privacy concerns. Furthermore, security and privacy concerns can make it challenging to store and transmit data securely, increasing the risk of unauthorized access and misuse.
Building trust in IoT ecosystems: A privacy-enhancing approach to cybersecurity
Best practices for ensuring security and privacy in IoT ecosystems
Ensuring security and privacy in IoT ecosystems requires a combination of technical expertise, strategic planning, and effective execution. Some of the best practices for ensuring security and privacy in IoT ecosystems include:
- Adopting security standards: Adhering to industry-wide security standards can help ensure that IoT devices and sensors are secure and can protect sensitive data.
- Implementing robust encryption: Implementing robust encryption, such as SSL/TLS, can help protect data transmitted between IoT devices and other components in the ecosystem.
- Implementing access controls: Implementing access controls, such as multi-factor authentication and role-based access control, can help ensure that only authorized users can access sensitive data.
- Conducting regular security audits: Conducting regular security audits can help organizations identify vulnerabilities and address security and privacy concerns proactively.
Ensuring security and privacy in IoT ecosystems are essential in enabling organizations to leverage data science to optimize their systems. Implementing best practices can help organizations minimize security and privacy risks and derive maximum value from their IoT ecosystems.
Final words
In closing, the combination of IoT and data science offers a world of endless possibilities for organizations looking to optimize their systems and processes. However, it also presents significant challenges, particularly around security and privacy.
To ensure the coherence of IoT and data science, organizations must take a comprehensive approach to data management and security, adopting best practices and adhering to industry standards. By doing so, they can unlock the full potential of their IoT ecosystems, derive valuable insights from their data, and make data-driven decisions that drive growth and success.
As IoT continues to evolve and expand, organizations that can effectively leverage data science to analyze IoT data will be well-positioned to thrive in the digital age.