- A CUNY Graduate Center research team has created CODE-AE, an artificial intelligence model that may dramatically improve accuracy and reduce the time and cost of pharmaceutical development.
- The therapeutic effect in a disease model may not necessarily correspond to pharmaceutical efficacy and toxicity in actual patients. This knowledge gap is a major factor in drug development’s high costs and low productivity rates.
- Researchers believe that the technique will significantly accelerate pharmaceutical development and precision medicine.
- CODE-AE beats current techniques in predicting patient-specific pharmaceutical reactions based only on cell-line chemical screenings.
The route from finding a possible therapeutic ingredient to FDA approval of a new medicine can take well over a decade and cost well over a billion dollars. A CUNY Graduate Center research team has developed an artificial intelligence model that might greatly increase the accuracy and lower the time and expense of medication development.
The accuracy of the CODE-AE model is promising
The new model, termed CODE-AE, is described in recent research published in Nature Machine Intelligence and can test novel medicinal molecules to predict efficacy on people reliably. In experiments, it was also able to theoretically discover tailored medications for over 9,000 people that may better treat their ailments. Researchers anticipate that the technology will considerably expedite medication development and precision medicine.
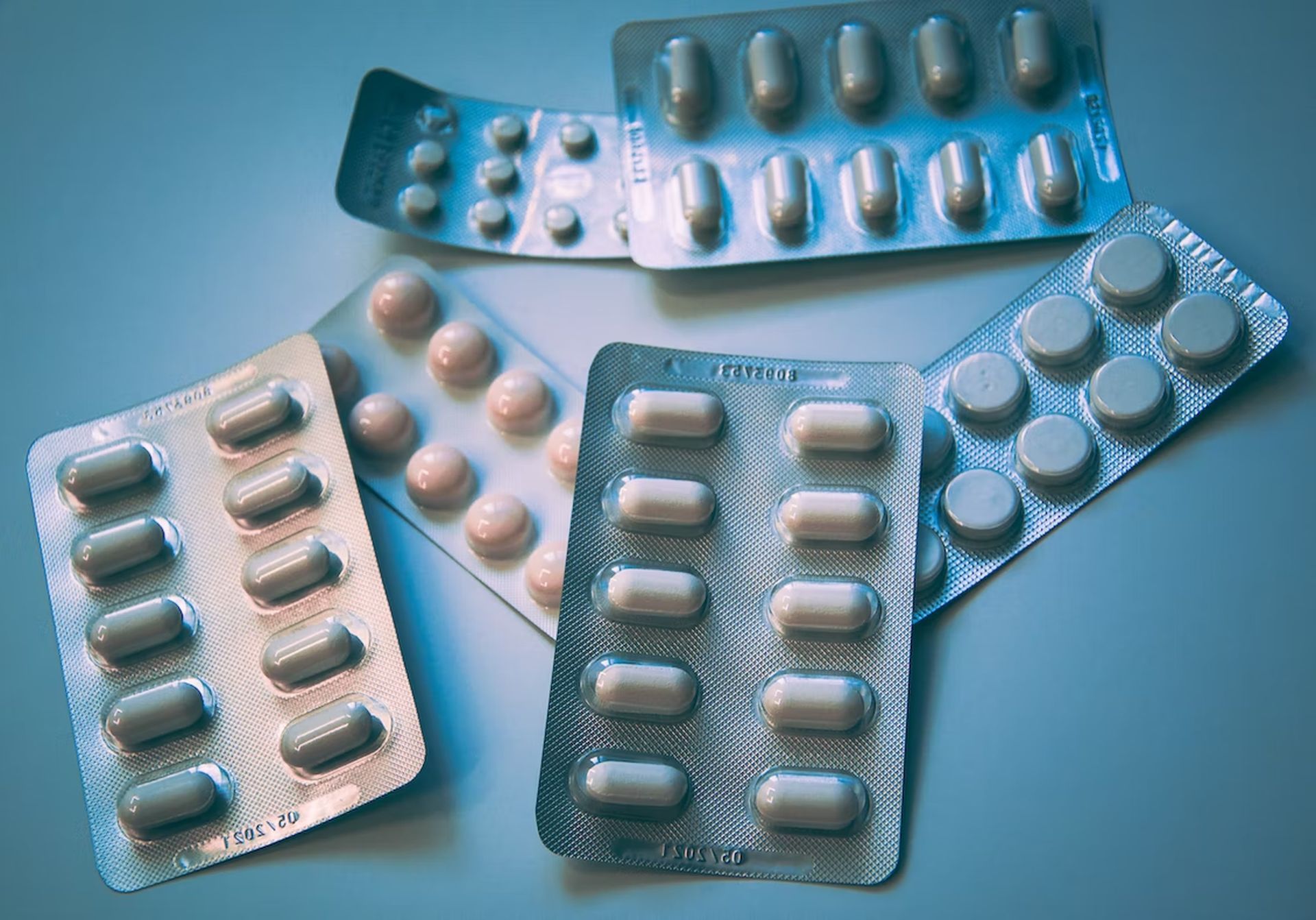
Accurate and robust prediction of patient-specific reactions to a new chemical molecule is crucial for discovering safe and effective therapies and selecting an existing medicine for a specific patient. However, directly studying a drug’s effectiveness in humans is both immoral and impossible.
To assess the therapeutic efficacy of a pharmacological molecule, cell or tissue models are frequently utilized as a proxy of the human body. Unfortunately, the therapeutic impact in a disease model does not always correlate with the medication efficacy and toxicity in actual patients. This information gap is a key contributor to the high costs and low productivity rates of drug development.
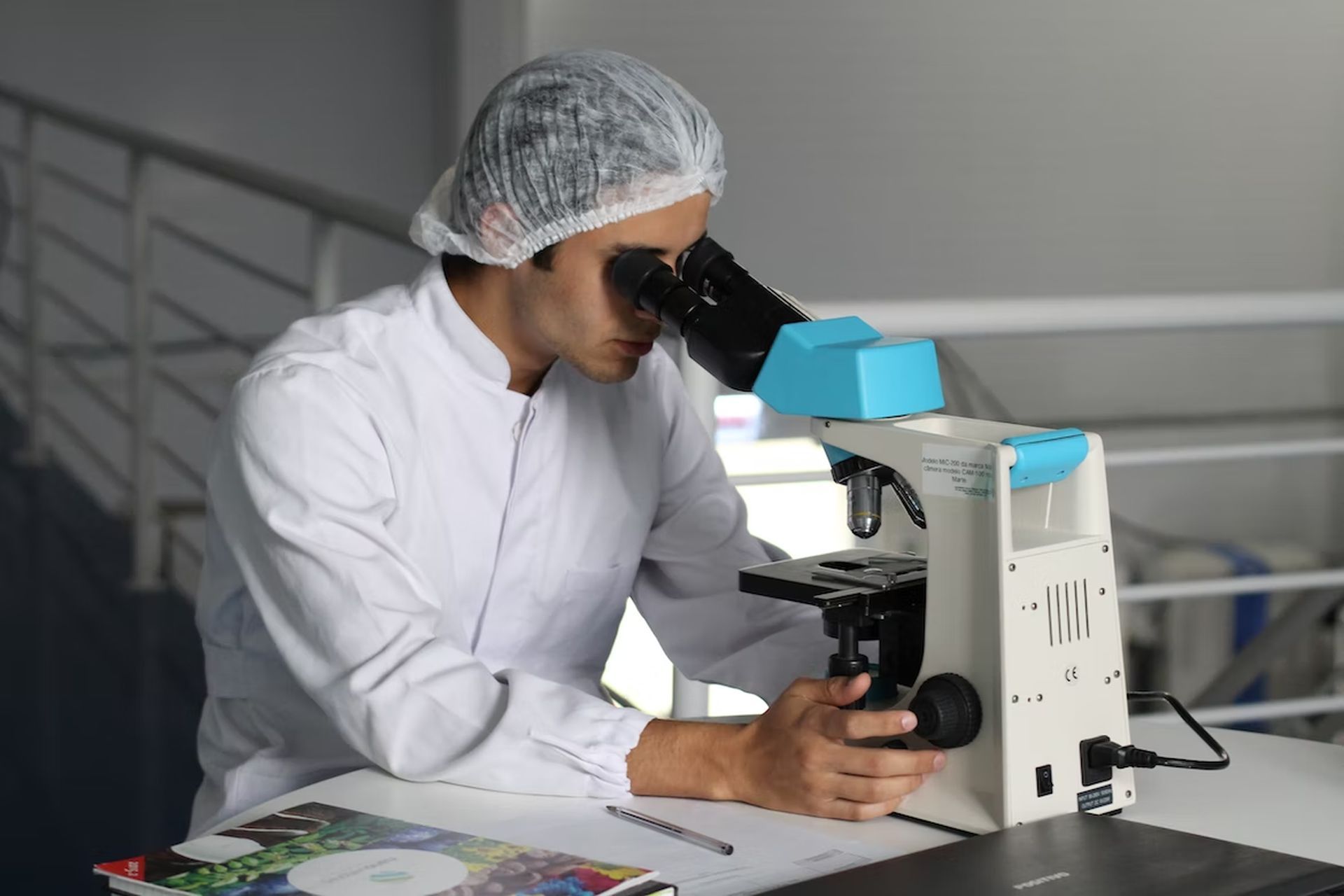
Lei Xie, the paper’s senior author and a professor of computer science, biology, and biochemistry at the CUNY Graduate Center and Hunter College said, “Our new machine learning model can address the translational challenge from disease models to humans. CODE-AE uses biology-inspired design and takes advantage of several recent advances in machine learning. For example, one of its components uses similar techniques in Deepfake image generation.”
According to You Wu, a CUNY Graduate Center Ph.D. student and co-author of the research, the new model can give a solution to the problem of not having enough patient data to train a generic machine learning model. “Although many methods have been developed to utilize cell-line screens for predicting clinical responses, their performances are unreliable due to data incongruity and discrepancies. CODE-AE can extract intrinsic biological signals masked by noise and confounding factors and effectively alleviated the data-discrepancy problem,” Wu added.
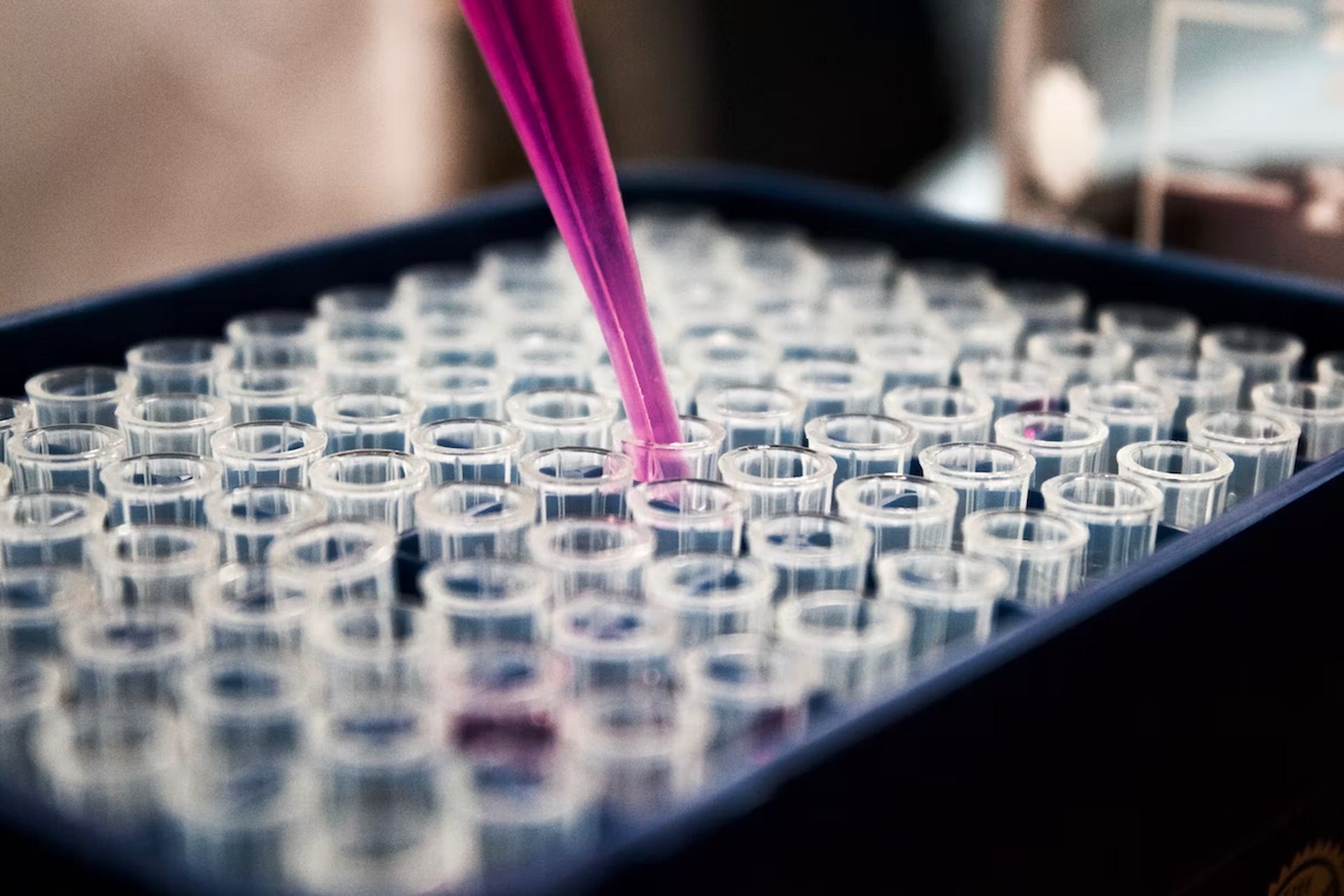
As a consequence, CODE-AE greatly outperforms state-of-the-art approaches in predicting patient-specific medication responses based only on cell-line chemical screens.
New artificial intelligence can diagnose a patient using their speech
Next hurdle that this artificial intelligence has to overcome
The next hurdle for the study team in extending the technology’s utility in drug development is to establish a method for CODE-AE to forecast the effect of a novel drug’s concentration and metabolization in human bodies. The researchers also mentioned that the AI model may be adjusted to anticipate human medication adverse effects correctly.