Artificial microswimmers that move similarly to naturally occurring swimming microorganisms have recently been the focus of some researchers. Microorganisms are all around us and are closely connected to how people live their daily lives. Microorganisms have piqued the interest of scientists ever since their discovery in the 19th century. They were cultivated for research purposes, but this process is costly and time-consuming.
However, high-throughput sequencing technology cannot be developed at the same rate as the culture approach. Machine learning (ML) and artificial intelligence (AI) techniques have been extensively used in microbiology to address this issue. For instance, last month, researchers from the University of Illinois at Urbana-Champaign showed how AI-based MARL method could improve cooperation between teams of robots.
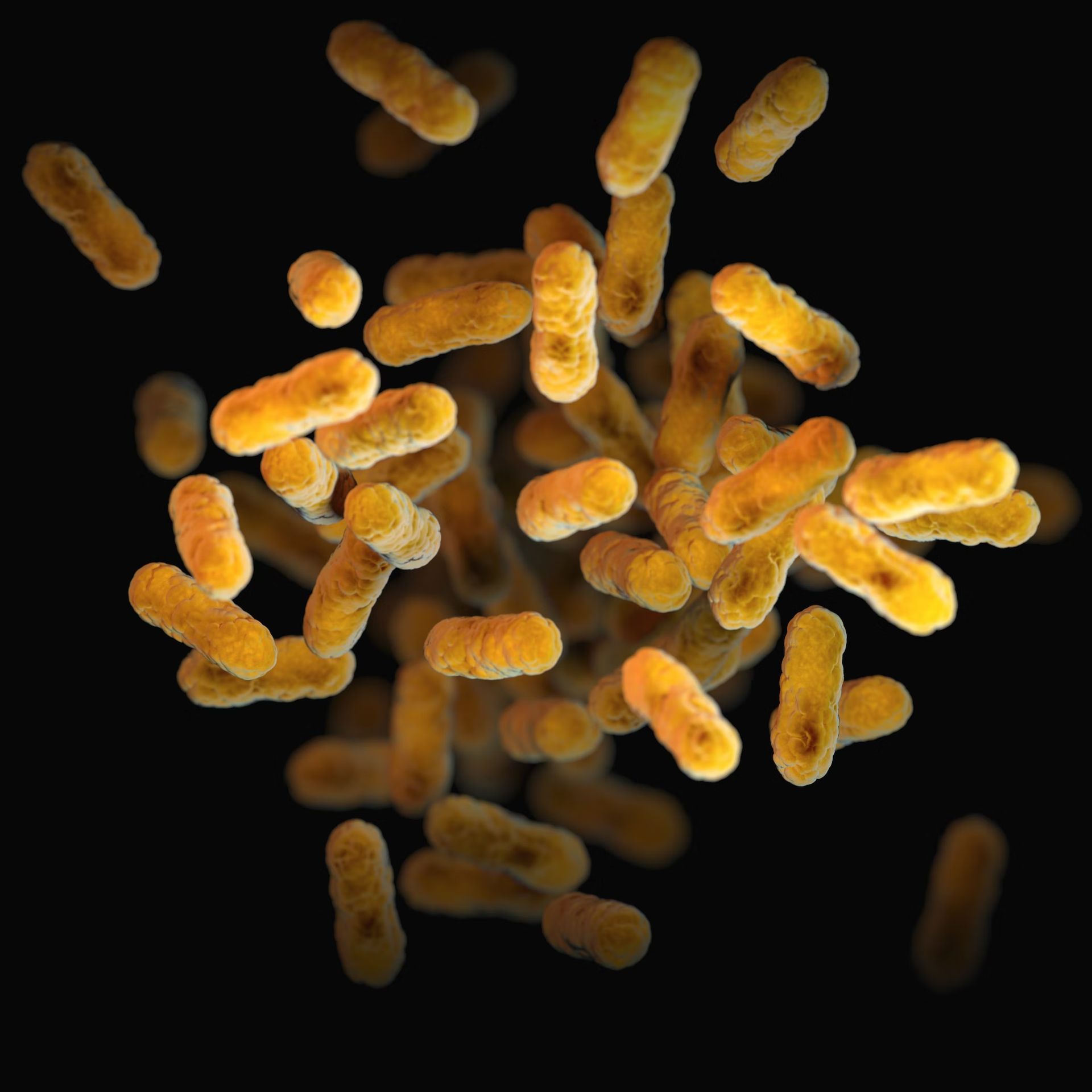
This time, deep reinforcement learning has been used effectively to teach microrobots how to swim by a team of researchers from Santa Clara University, New Jersey Institute of Technology, and the University of Hong Kong. The latest innovation significantly improves the capabilities of micro swimming.
The goal of scientists has been to develop artificial microswimmers that can move in a manner comparable to naturally occurring swimming microorganisms, such as bacteria. Future biological uses for these microswimmers could include microsurgery and tailored drug administration, among other things. Even with the emphasis on improvement, most artificial microswimmers on the market today can only carry out straightforward moves with fixed locomotory gaits.
AI-supported microswimmers can perform sophisticated tasks
The research team showed in their study published in Communications Physics that AI might instruct microswimmers to adjust to shifting environmental conditions. Similar to humans, microswimmers also need reinforcement learning and feedback to learn to swim, but they face different difficulties.
“Being able to swim at the micro-scale by itself is a challenging task. When you want a microswimmer to perform more sophisticated maneuvers, the design of their locomotory gaits can quickly become intractable,” said the study’s co-author and an associate professor of mechanical engineering at Santa Clara University, On Shun Pak.
Method
The team successfully trained a straightforward microswimmer to swim and navigate in the direction of an arbitrary direction by combining reinforcement learning and artificial neural networks (ANNs). The microswimmer receives input on its particular movements, which it uses to learn how to swim by interacting with its surroundings gradually.
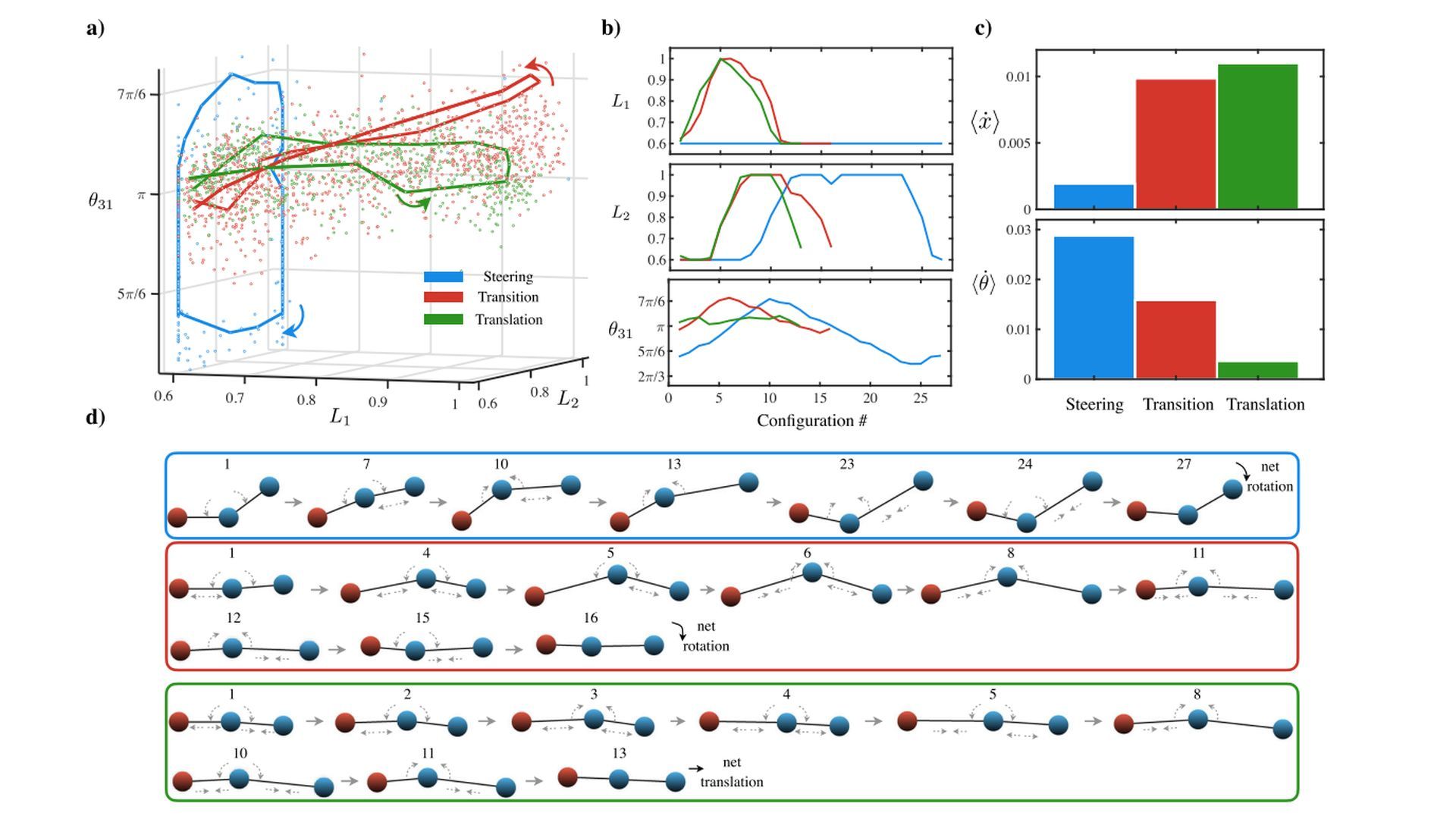
Swimming microorganisms alternate between locomotory gaits to use intricate navigational methods like run-and-tumble to explore their habitats and look for specific targets. Targeted medication distribution and microsurgery are two challenging biomedical tasks that intelligent artificial microswimmers can carry out autonomously. This capability of targeted navigation via adaptive gait-switching is particularly important in this context.
Researchers applied a deep reinforcement learning approach to enable a model microswimmer to self-learn efficient locomotory gaits for translation, rotation, and combination motions. The AI-powered swimmer may adaptively transition between several locomotory gaits to go in the direction of its destination. Similar gait-switching patterns swimming microorganisms use are suggestive of the multimodal navigation method.
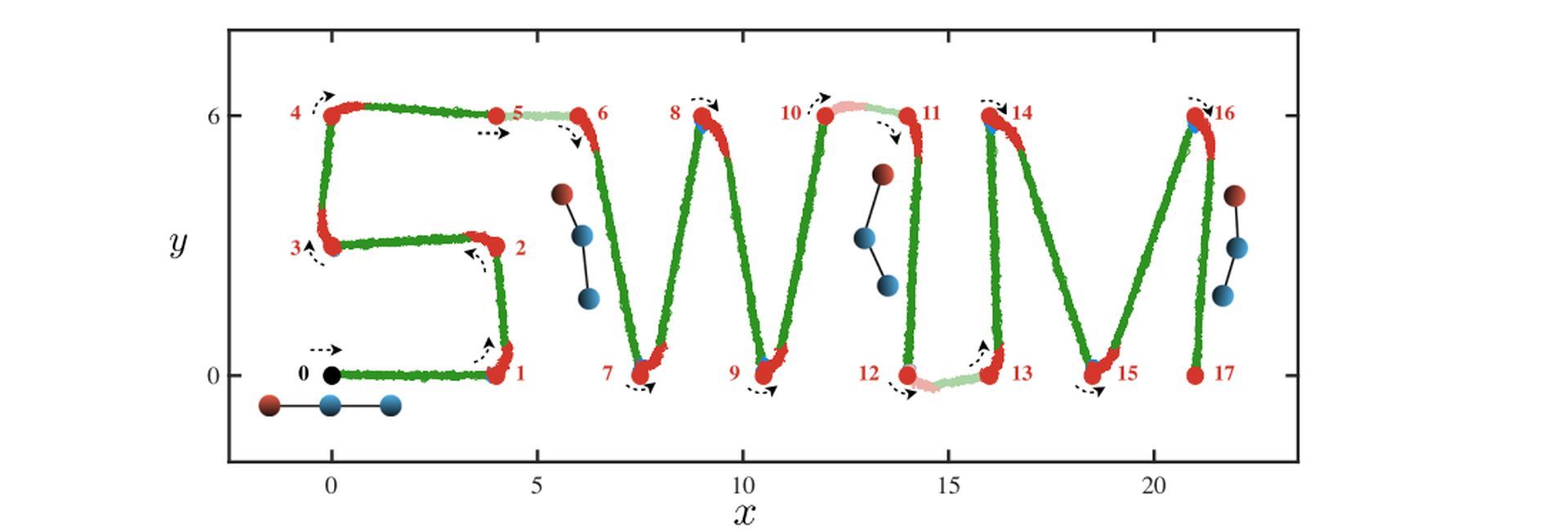
“Similar to a human learning how to swim, the microswimmer learns how to move its ‘body parts’ — in this case three microparticles and extensible links — to self-propel and turn. It does so without relying on human knowledge but only on a machine learning algorithm,” the co-author of the study and an assistant professor of mechanical engineering at the University of Hong Kong, Alan Tsang.
The AI-powered swimmer may adaptively flip between various locomotory gaits, allowing it to navigate to any target place autonomously.
Researchers are developing an AI pilot capable of navigating crowded airspaces
The ability of the microswimmer to follow a complex path without being explicitly programmed was used by the researchers to illustrate its strength.
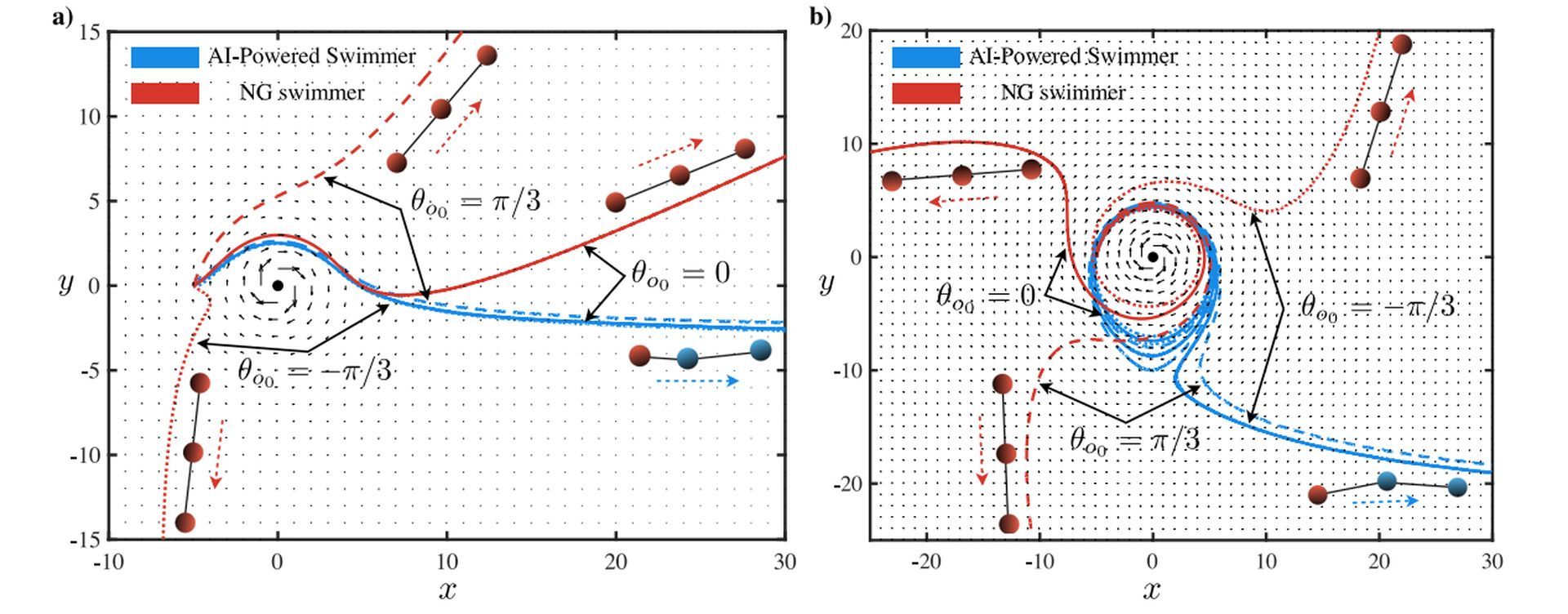
“This is our first step in tackling the challenge of developing microswimmers that can adapt like biological cells in navigating complex environments autonomously,” said the study’s co-author, Yuan-nan Young, professor of mathematical sciences at the New Jersey Institute of Technology.
These adaptive actions are what offer the most potential for biological applications.
“This work is a key example of how the rapid development of artificial intelligence may be exploited to tackle unresolved challenges in locomotion problems in fluid dynamics. The integration between machine learning and microswimmers in this work will spark further connections between these two highly active research areas,” commented Arnold Mathijssen, an expert on microrobots and biophysics at the University of Pennsylvania who wasn’t involved in the study.