P-computers might change the future of information technologies for good. There is an urgent demand for additional technology that is scalable and energy-efficient due to the advent of artificial intelligence (AI) and machine learning (ML). Making judgments based on insufficient data is a crucial step in both AI and ML, and the optimal strategy is to output a probability for each potential response.
P-computers are powered by probabilistic bits
Due of the inability of current classical computers to do that task in an energy-efficient manner, researchers are looking for new computing paradigms. Qubit-based quantum computers may be able to assist in overcoming these difficulties, but they are still in the early phases of research and are very sensitive to their environment.
It is an inevitable fact that artificial intelligence will completely change the future. Apart from scientific developments, legal regulations seem to pave the way for the use of artificial intelligence, for instance, UK eases restrictions on data mining laws to facilitate AI industry growth.
Kerem Camsari, an assistant professor of electrical and computer engineering (ECE) at UC Santa Barbara, believes that probabilistic computers (p-computers) are the solution. P-computers are powered by probabilistic bits (p-bits), which interact with other p-bits in the same system. Unlike the bits in classical computers, which are in a 0 or a 1 state, or qubits, which can be in more than one state at a time, p-bits fluctuate between positions and operate at room temperature. In an article published in Nature Electronics, Camsari and his collaborators discuss their project that demonstrated the promise of p-computers.
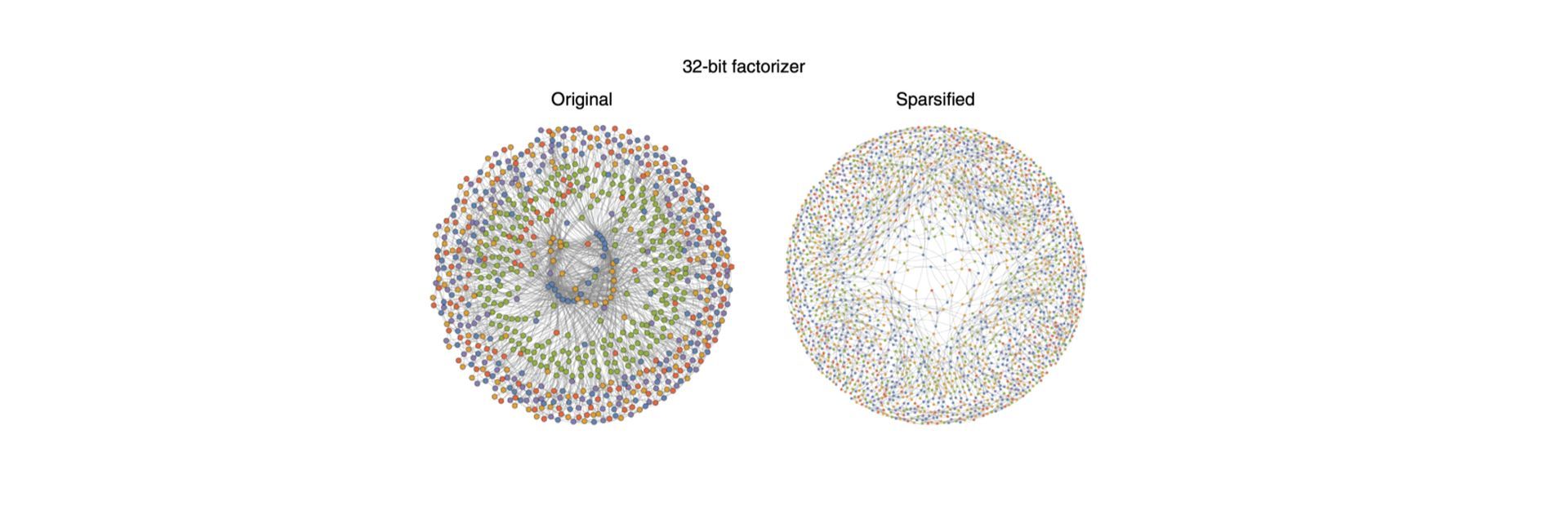
“We showed that inherently probabilistic computers, built out of p-bits, can outperform state-of-the-art software that has been in development for decades,” said Camsari.
Researchers from the University of Messina in Italy, vice chair of the UCSB ECE department Luke Theogarajan, and physics professor John Martinis, who oversaw the group that created the first quantum computer to attain quantum supremacy, all worked with Camsari’s team. Together, the researchers produced their encouraging results utilizing domain-specific architectures built on traditional hardware. They created a special sparse Ising machine (sIm), a cutting-edge computing system designed to address optimization issues and reduce energy usage.
According to Camsari, the sIm is a group of probabilistic bits that may be compared to individuals. Additionally, each individual only has a tiny group of close friends, or “sparse” relationships, in the system.
“The people can make decisions quickly because they each have a small set of trusted friends and they do not have to hear from everyone in an entire network. The process by which these agents reach consensus is similar to that used to solve a hard optimization problem that satisfies many different constraints. Sparse Ising machines allow us to formulate and solve a wide variety of such optimization problems using the same hardware,” explained Camsari.
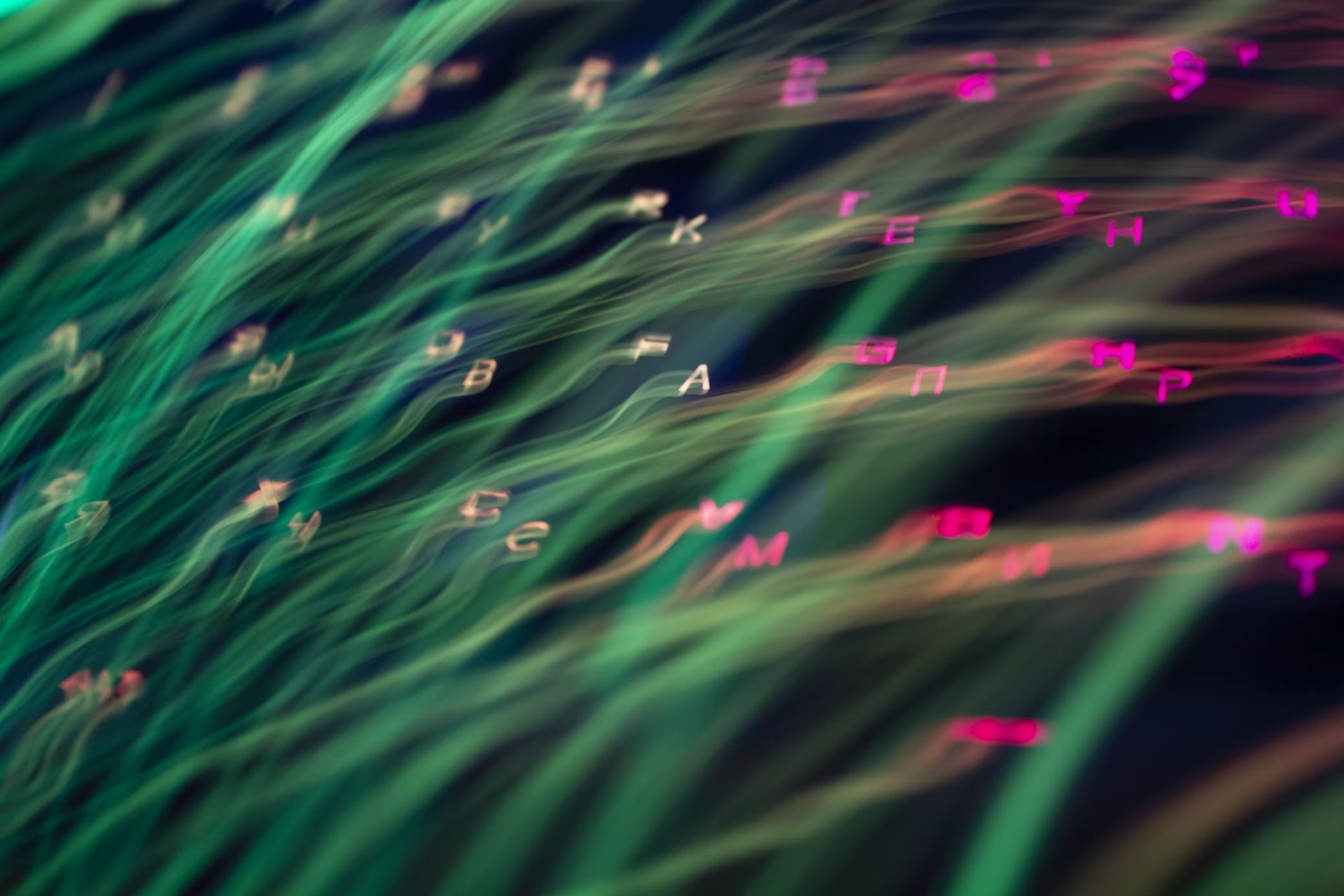
Field-programmable gate arrays (FPGAs), a potent piece of hardware that offers far more flexibility than application-specific integrated circuits, were a component of the team’s prototyped design.
“Imagine a computer chip that allows you to program the connections between p-bits in a network without having to fabricate a new chip,” said Camsari.
The researchers demonstrated that their sparse design on FPGAs has boosted sampling speed five to eighteen times quicker than those attained by optimized methods employed on conventional computers, which was up to six orders of magnitude faster.
Additionally, they stated that their sIm achieves huge parallelism where the number of p-bits grows linearly with the number of flips per second, the fundamental metric used to determine how rapidly a p-computer can make an educated decision. Camsari returns to the image of two reliable friends attempting to decide.
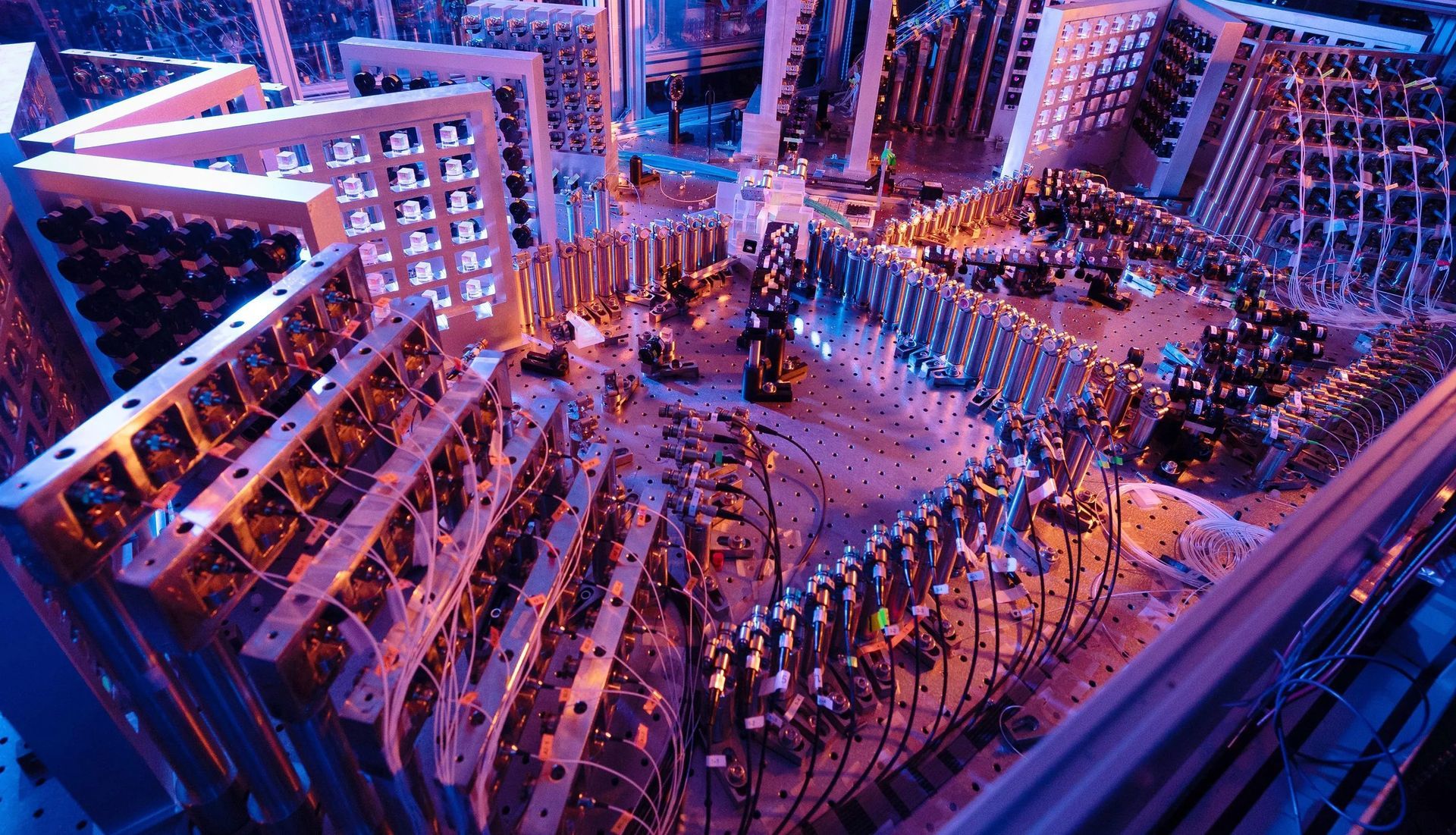
“The key issue is that the process of reaching a consensus requires strong communication among people who continually talk with one another based on their latest thinking. If everyone makes decisions without listening, a consensus cannot be reached and the optimization problem is not solved,” added Camsari.
In other words, it is important to increase the flips per second while making sure that everyone listens to each other since the faster the p-bits communicate, the faster a consensus may be formed.
“This is exactly what we achieved in our design. By ensuring that everyone listens to each other and limiting the number of ‘people’ who could be friends with each other, we parallelized the decision-making process,” explained Camsari.
While acknowledging that their ideas are only one part of the p-computer jigsaw, Camsari finds their work incredibly promising because it demonstrated the capacity to grow p-computers up to 5,000 p-bits.
“To us, these results were the tip of the iceberg. We used existing transistor technology to emulate our probabilistic architectures, but if nanodevices with much higher levels of integration are used to build p-computers, the advantages would be enormous. This is what is making me lose sleep,” added Camsari.
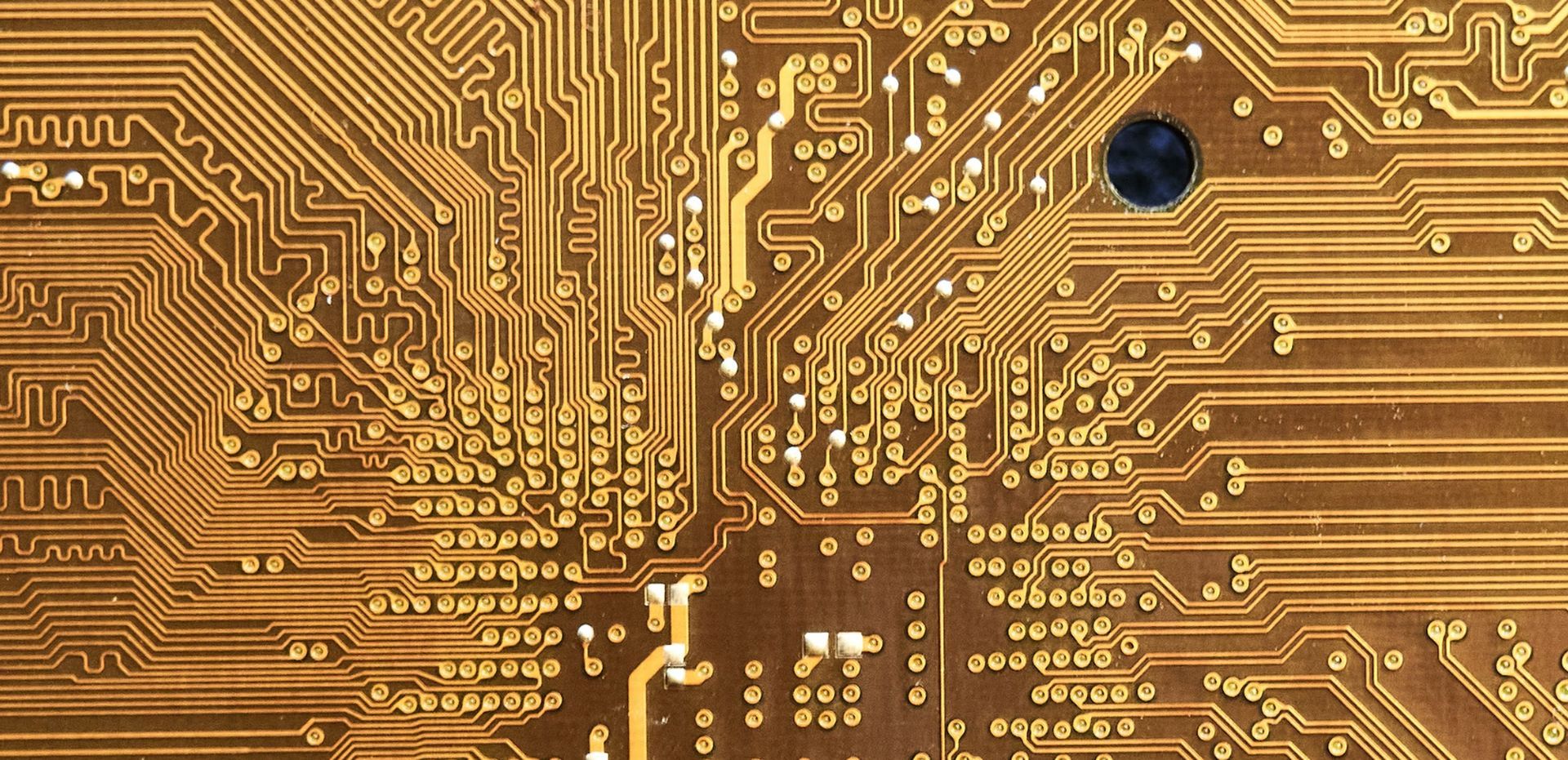
The device’s potential was originally demonstrated by an 8 p-bit p-computer created by Camsari and his partners while he was a graduate student and postdoctoral researcher at Purdue University. Their article, which was published in 2019 in Nature, detailed a ten-fold decrease in the energy it used and a hundred-fold decrease in the area footprint. Camsari and Theogarajan were able to further their p-computer research thanks to seed funding from UCSB’s Institute for Energy Efficiency, which supported the study published in Nature Electronics.
“The initial findings, combined with our latest results, mean that building p-computers with millions of p-bits to solve optimization or probabilistic decision-making problems with competitive performance may just be possible,” said Camsari.
The study team anticipates that one day, p-computers will be more quicker and more effective at handling a certain class of tasks, ones that are inherently probabilistic. If you liked this article check out how the latest study showed it is possibe to improve the interpretability of ML features for end-users.