Spiking neural networks (SNNs) are artificial networks that may be used to build intelligent robots or to learn more about the mechanisms behind the brain. Despite their small size, our brains have a significant amount of computing power. Since the brain processes neural signals in this way, many academics have been interested in developing artificial networks that do the same.
The brain, on the other hand, has 100 billion tiny neurons, each of which is synaptically coupled to 10,000 other neurons and represents information through timed electrical spiking patterns. It has been difficult to mimic these neurons with hardware on a small device while also making sure that computation is carried out in an energy-efficient way. Recently, MIT researchers have built a new LEGO-like AI chip with sustainability concerns.
SNNs rely on a network of artificial neurons
In a recent study, scientists in India developed ultralow-energy artificial neurons that enable the SNNs to be placed more densely. IEEE Transactions on Circuits and Systems I: Regular Papers published the findings on May 25, according to IEEE Spectrum.
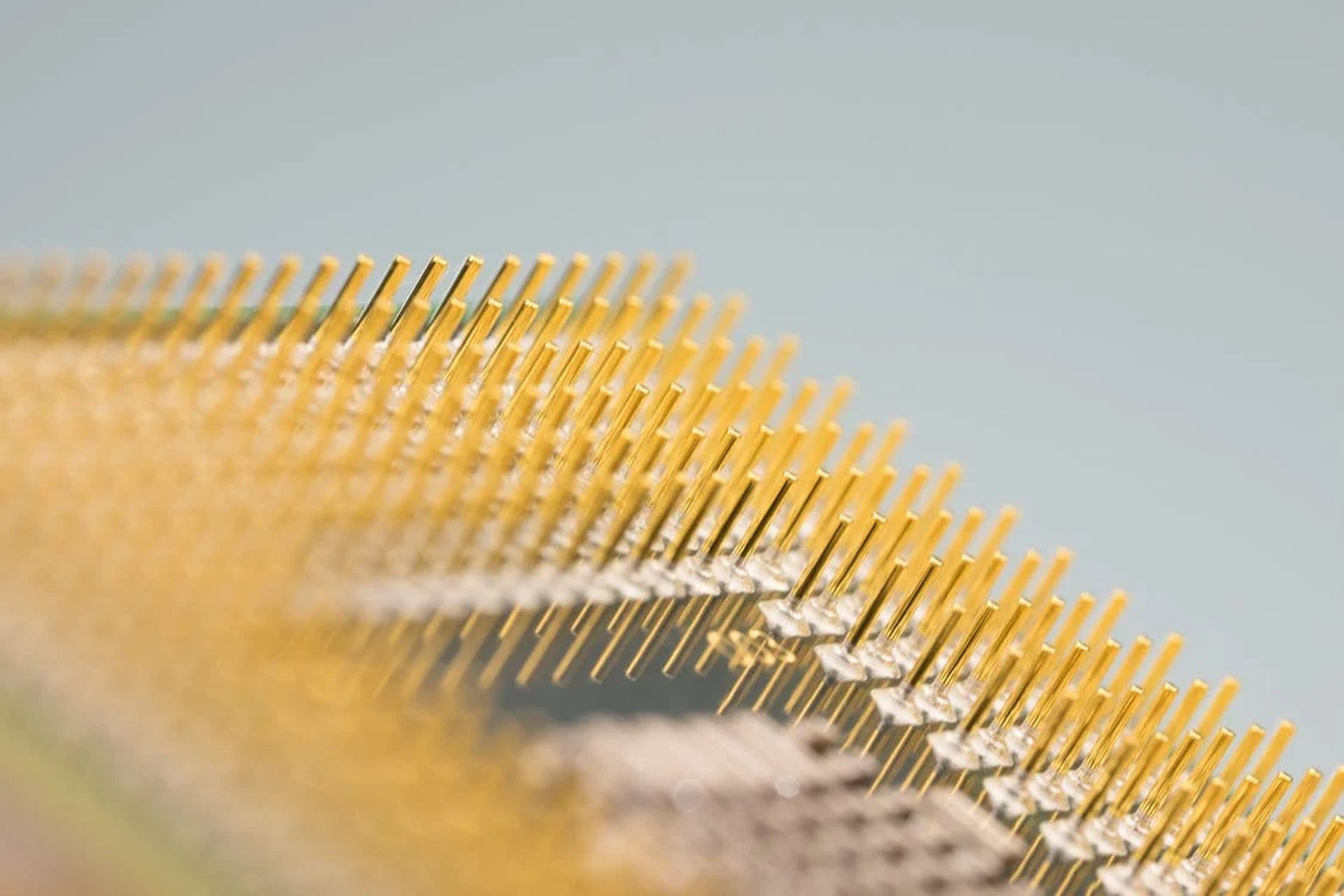
SNNs rely on a network of artificial neurons where a current source charges up a leaky capacitor until a threshold level is met and the artificial neurons fire. When the artificial neurons fire, the stored charge is reset to zero, just like neurons in the brain spike at a certain energy threshold. However, a lot of present SNNs need significant transistor currents to fill up their capacitors, which results in high power consumption or too-rapid artificial neuron firing.
In their research, Indian Institute of Technology, Professor Udayan Ganguly and his colleagues developed an SNN that uses band-to-band-tunneling (BTBT) current, a novel and portable current source, to charge capacitors.
Less energy is needed when using BTBT because quantum tunneling current charges the capacitor with ultralow current. The aim is to create an energy-efficient model, just like LEGO-like AI chip. In this instance, quantum tunneling refers to the phenomenon of quantum waves that allows current to pass through the artificial neuron’s silicon’s forbidding gap.
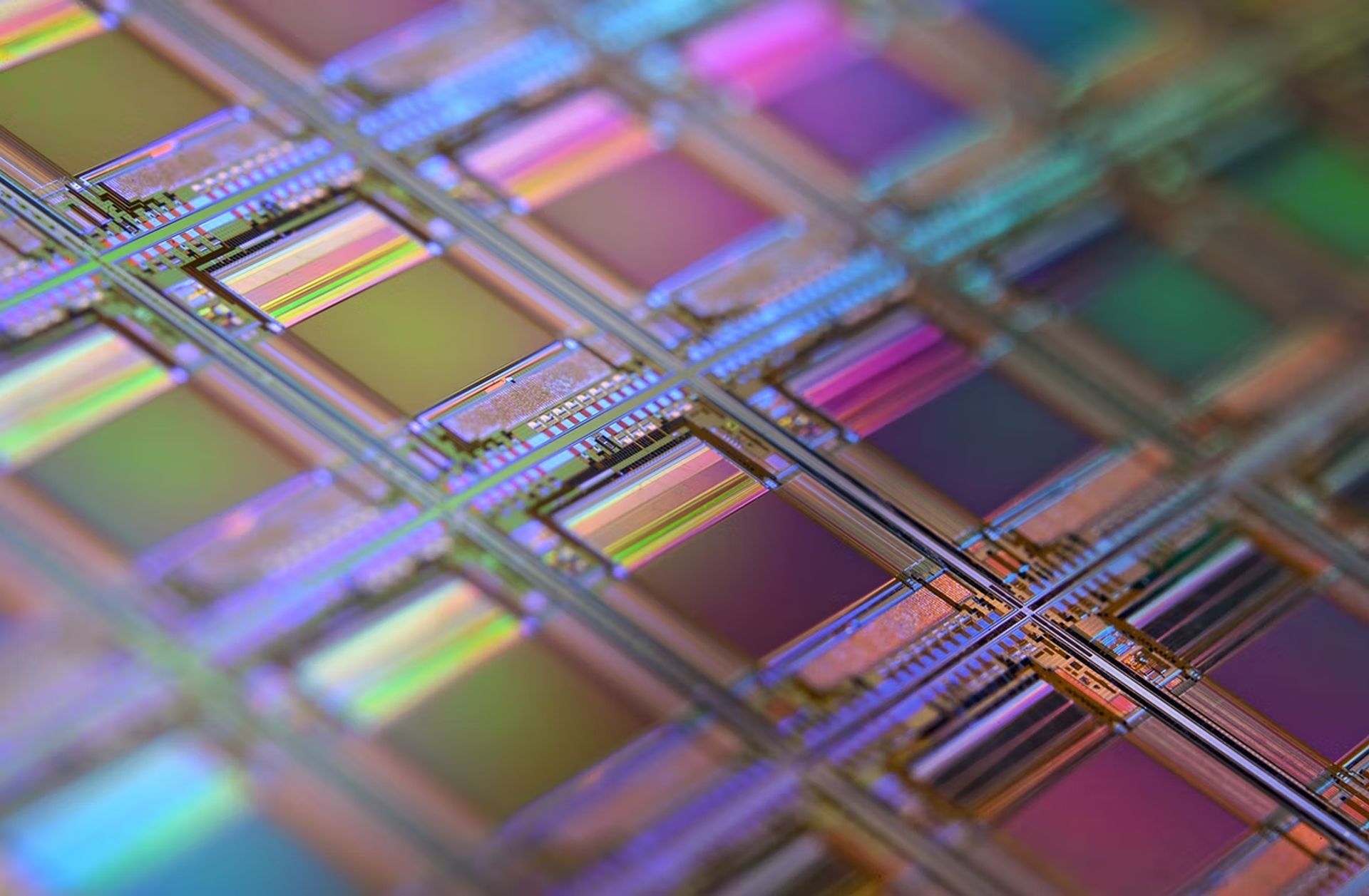
The BTBT method also eliminates the requirement for huge capacitors to store significant quantities of current, opening the door for smaller capacitors on a chip and resulting in space savings. The researchers saw significant energy and space reductions when they tested their BTBT neuron strategy utilizing 45-nanometer commercial silicon-on-insulator transistor technology.
“In comparison to state-of-art [artificial] neurons implemented in hardware spiking neural networks, we achieved 5,000 times lower energy per spike at a similar area and 10 times lower standby power at a similar area and energy per spike,” said Ganguly.
Following that, the researchers used their SNN to improve a speech recognition model based on the auditory cortex of the brain. The model successfully recognized spoken words with the aid of 36 extra artificial neurons and 20 artificial neurons for initial input coding, proving the approach’s viability in the real world.
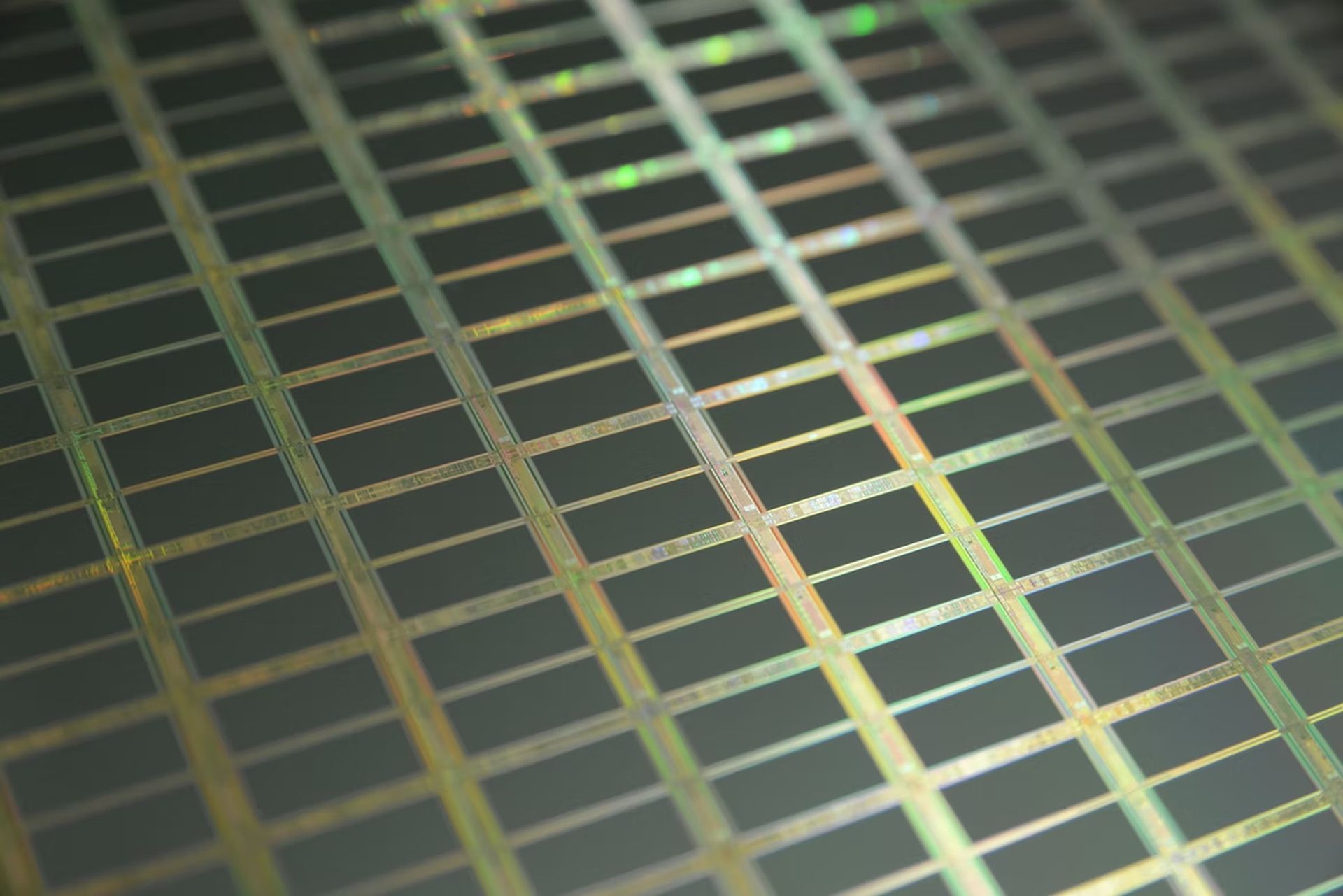
Notably, this kind of technology may be suitable for a variety of tasks, such as voice-activity detection, speech classification, motion-pattern identification, navigation, biological signals, and more. Ganguly further points out that while these applications can be run on existing servers and supercomputers, SNNs could make it possible to utilize them with edge devices, such mobile phones and Internet of Things sensors, especially in cases when energy is at a premium.
Although his team has shown the effectiveness of their BTBT approach for specific applications, such as keyword detection, he asserts that they are interested in showing off a general-purpose reusable neurosynaptic core for a variety of applications and clients and have set up a startup company called Numelo Tech to support commercialization.
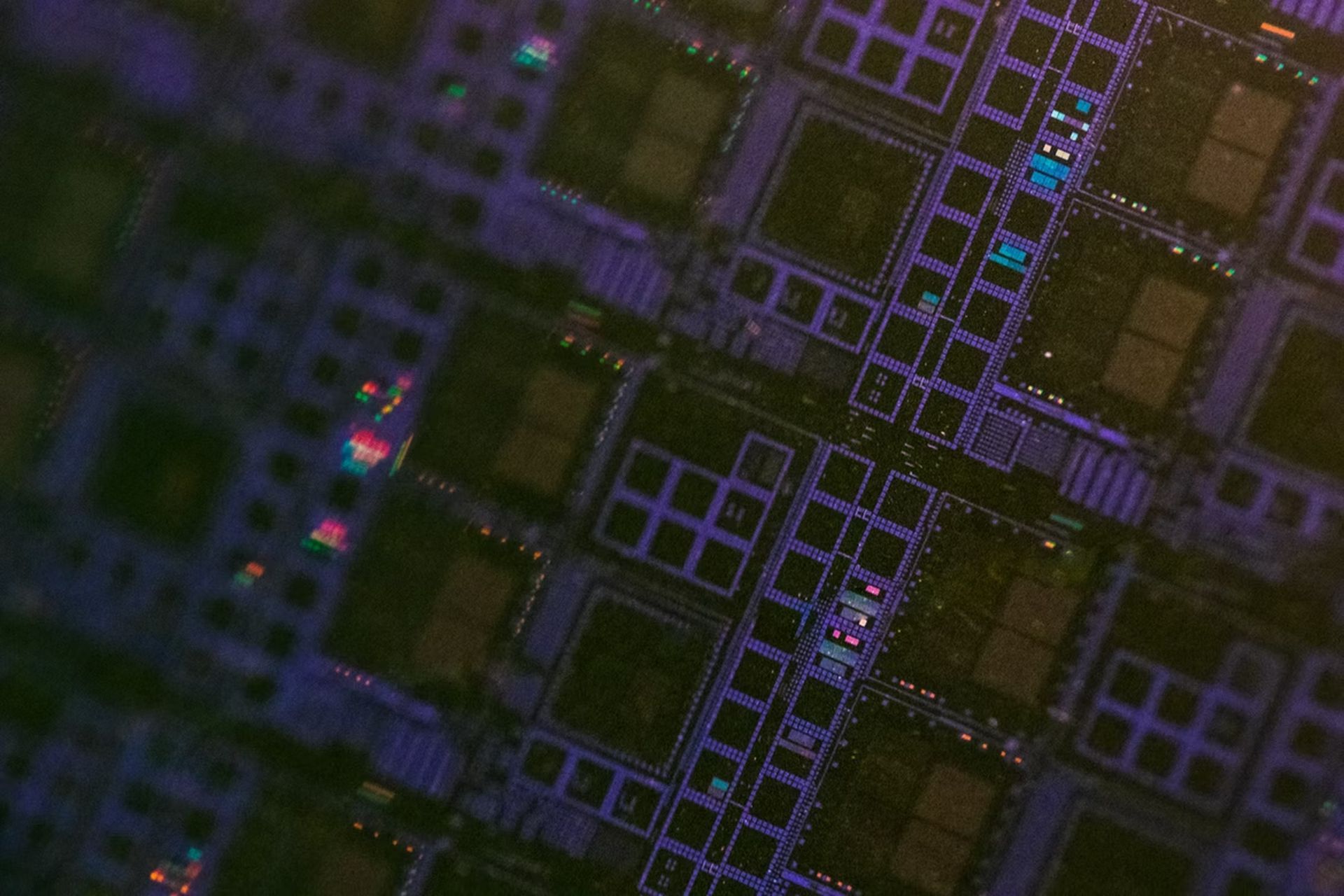
The ultimate goal is to develop “an extremely low-power neurosynaptic core and developing a real-time on-chip learning mechanism, which are key for autonomous biologically inspired neural networks. This is the holy grail.” These kind of efforts, just like LEGO-like AI chip, is very important for creating a sustainable world. The latest Schneider Electric research also points out that the sector professionals don’t meet their IT sustainability promises.