Hugging Face has released two new AI models, SmolVLM-256M and SmolVLM-500M, claiming they are the smallest of their kind capable of analyzing images, videos, and text on devices with limited RAM, such as laptops.
Hugging Face launches compact AI models for image and text analysis
A Small Language Model (SLM) is a neural network designed to produce natural language text. The descriptor “small” applies not only to the physical dimensions of the model but also to its parameter count, neural structure, and the data volume used during training.
SmolVLM-256M and SmolVLM-500M consist of 256 million parameters and 500 million parameters, respectively. These models can perform various tasks, including describing images and video clips, as well as answering questions about PDFs and their contents, such as scanned text and charts.
Sam Altman to brief officials on ‘PhD-level’ super AI
To train these models, Hugging Face utilized The Cauldron, a curated collection of 50 high-quality image and text datasets, alongside Docmatix, a dataset comprising file scans with detailed captions. Both datasets were created by Hugging Face’s M4 team, focused on multimodal AI technologies.
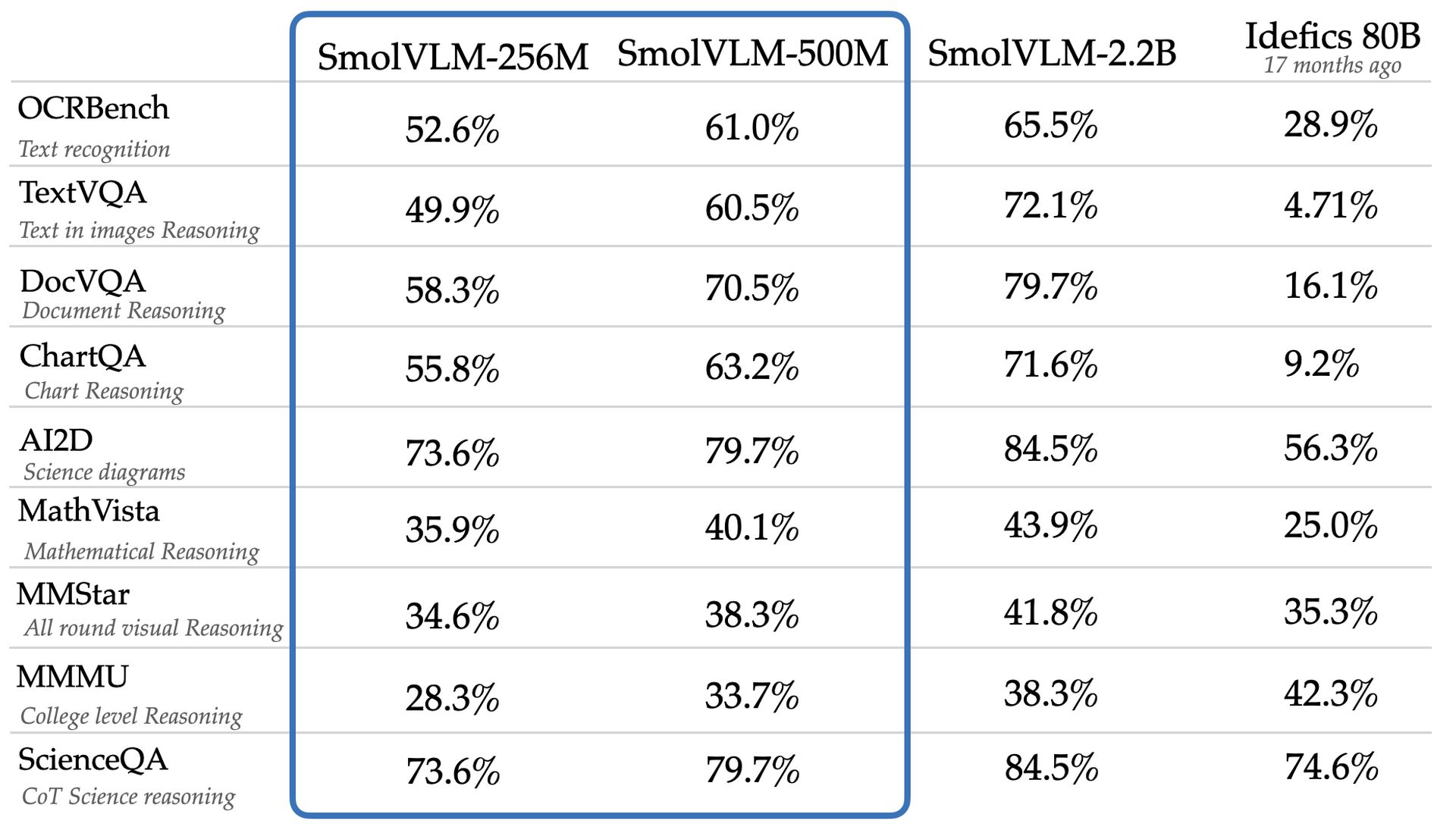
The team asserts that SmolVLM-256M and SmolVLM-500M outperform a significantly larger model, Idefics 80B, in benchmarks such as AI2D, which assesses models’ abilities to analyze grade-school-level science diagrams. The new models are available for web access and download under an Apache 2.0 license, which allows unrestricted use.
Despite their versatility and cost-effectiveness, smaller models like SmolVLM-256M and SmolVLM-500M may exhibit limitations not observed in larger models. A study from Google DeepMind, Microsoft Research, and the Mila research institute highlighted that smaller models often perform suboptimally on complex reasoning tasks, potentially due to their tendency to recognize surface-level patterns rather than applying knowledge in novel contexts.
Hugging Face’s SmolVLM-256M model operates with less than one gigabyte of GPU memory and outperforms the Idefics 80B model, a system 300 times larger, achieving this reduction and enhancement within 17 months. Andrés Marafioti, a machine learning research engineer at Hugging Face, noted that this achievement reflects a significant breakthrough in vision-language models.
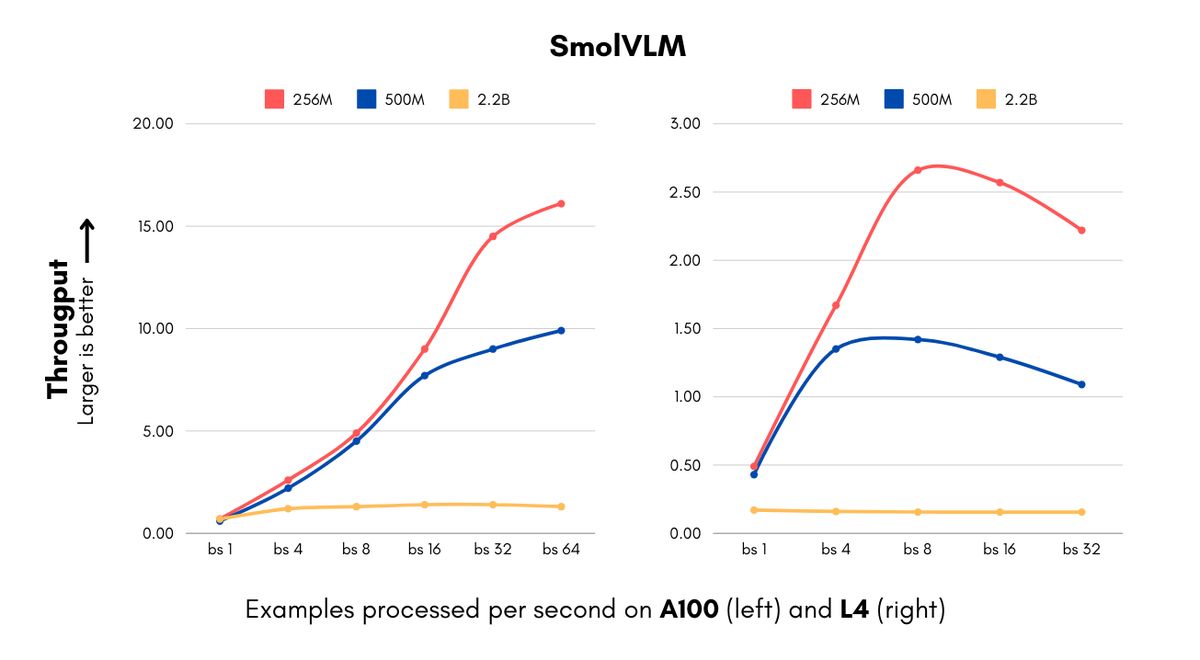
The introduction of these models is timely for enterprises facing high computing costs associated with AI implementations. The SmolVLM models are capable of processing images and understanding visual content at unprecedented speeds for models of their size. The 256M version can process 16 examples per second while consuming only 15GB of RAM with a batch size of 64, leading to considerable cost savings for businesses handling large volumes of visual data.
IBM has formed a partnership with Hugging Face to incorporate the 256M model into its document processing software, Docling. As Marafioti explained, even organizations with substantial computing resources can benefit from using smaller models to efficiently process millions of documents at reduced costs.
Hugging Face achieved size reductions while maintaining performance through advancements in both vision processing and language components, including a switch from a 400M parameter vision encoder to a 93M parameter version and the use of aggressive token compression techniques. This efficiency opens new possibilities for startups and smaller enterprises, enabling them to develop sophisticated computer vision products more rapidly and reduce their infrastructure costs.
The SmolVLM models enhance capabilities beyond cost savings, facilitating new applications like advanced document search through an algorithm named ColiPali, which creates searchable databases from document archives. According to Marafioti, these models nearly match the performance of models 10 times their size while significantly increasing the speed of database creation and search, making enterprise-wide visual search feasible for various businesses.
The SmolVLM models challenge the conventional belief that larger models are necessary for advanced vision-language tasks, with the 500M parameter version achieving 90% of the performance of a 2.2B parameter counterpart on key benchmarks. Marafioti highlighted that this development demonstrates the usefulness of smaller models, suggesting that they can play a crucial role for businesses.
Featured image credit: Hugging Face