For some time, there have been whispers that Google might introduce a fee for AI-enhanced search results, particularly through a proposed premium search service that incorporates generative AI. The future of this development is still uncertain, yet a clear shift is evident as Google discontinues free access to its Gemini API, marking a strategic pivot in its AI financial approach.
Google initially provided free access to its AI offerings to attract developers and compete with OpenAI’s early market presence. OpenAI had already begun to capitalize on its APIs and large language models. Now, Google aims to follow suit by monetizing services within its cloud and AI Studio offerings, suggesting that the era of unrestricted free access may be drawing to a close.
PaLM API is long gone
In an email directed at developers reported by Techradar, Google announced that it would discontinue the PaLM API, a precursor to the Gemini model used for crafting custom chatbots, via AI Studio as of August 15. This API was already phased out in February. Google’s strategy is to transition users from the free service by promoting the more reliable Gemini 1.0 Pro. The email advises:
“We encourage testing prompts, tuning, inference, and other features with stable Gemini 1.0 Pro to avoid interruptions. You can use the same API key you used for the PaLM API to access Gemini models through Google AI SDKs.”
The cost structure for the paid version starts at $7 for one million input tokens and escalates to $21 for the same quantity of output tokens. An exception exists in Google’s plan; both PaLM and Gemini will continue to be available to clients who subscribe to Vertex AI within Google Cloud.
PaLM and Gemini will continue to be available to those who have invested in Vertex AI on Google Cloud. In contrast, AI Studio remains the more accessible choice for developers working with tighter budgets who find Vertex AI too costly.
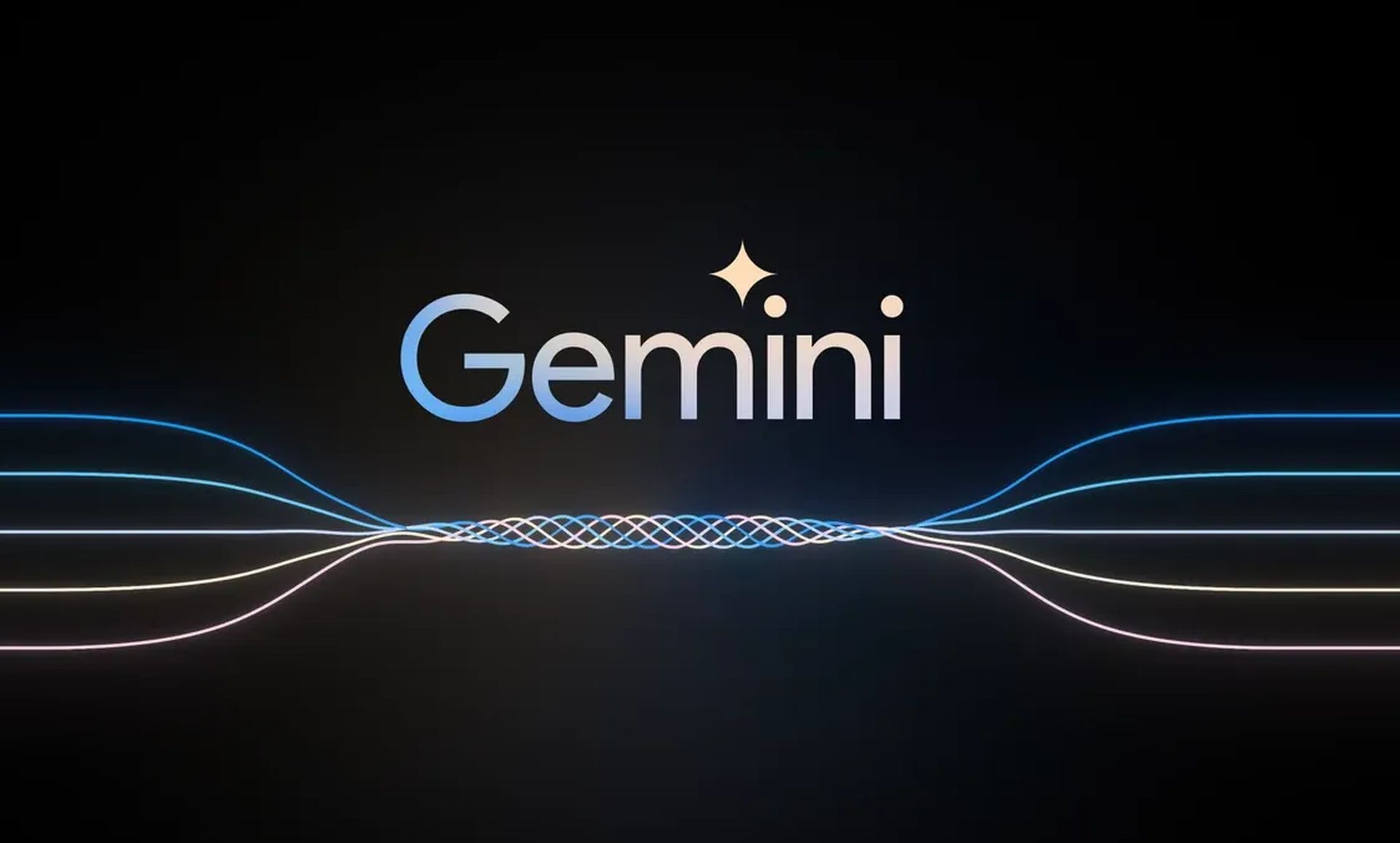
Google’s APIs are powered by the hardware within the company’s own data centers, with Gemini operating on TPUs that are specifically designed for both training and inferencing tasks.
In a significant investment into infrastructure, Google has allocated billions towards the construction of new data centers, including a recent $1 billion commitment to a facility in the UK.
The substantial investments into data centers to support AI operations represent a considerable risk, given the absence of established AI revenue models. However, as the adoption of large language models (LLMs) expands, modest revenue streams from services like APIs could help offset the substantial costs associated with developing the necessary hardware and data centers.
Similarly, other AI companies are making multi-billion dollar investments in new data centers, aiming to generate sufficient AI-driven revenue to cover these substantial expenses.
Featured image credit: Mitchell Luo/Unsplash