A new AI model called VASA-1 promises to transform your photos into videos and give them a voice. This exciting technology from Microsoft uses a single portrait photo and an audio file to create a talking face video with realistic lip-syncing, facial expressions, and head movements.
The power of VASA-1
VASA-1’s capabilities lie in its ability to generate lifelike facial animations. Unlike its predecessors, VASA-1 minimizes errors around the mouth, a common telltale sign of deepfakes. Additionally, it boasts high-quality realism through its nuanced understanding of facial expressions and natural head movements.
Demo videos published by Microsoft in a blog post showcase the impressive results, blurring the lines between reality and AI-generated content.
Where VASA-1 could shine you ask? Well,
- Enhanced gaming experiences: Imagine in-game characters with perfectly synchronized lip movements and expressive faces, creating a more immersive and engaging gameplay experience.
- Personalized virtual avatars: VASA-1 could change social media by allowing users to create hyper-realistic avatars that move and speak just like them.
- AI-powered filmmaking: Filmmakers could use VASA-1 to generate realistic close-up shots, intricate facial expressions, and natural dialogue sequences, pushing the boundaries of special effects.
How VASA-1 works?
VASA-1 tackles the challenge of generating realistic talking face videos from a single image and an audio clip. Let’s delve into the technical aspects of how it achieves this remarkable feat.
Imagine a photo of someone and an audio recording of a different person speaking. VASA-1 aims to combine these elements to create a video where the person in the photo appears to be saying the words from the audio. This video should be realistic in several key aspects:
- Image clarity and authenticity: The generated video frames should look like real footage and not exhibit any artificial-looking artifacts.
- Lip-sync accuracy: The lip movements in the video must perfectly synchronize with the audio.
- Facial expressions: The generated face should display appropriate emotions and expressions to match the spoken content.
- Natural head movements: Subtle head movements should enhance the realism of the talking face.
VASA-1 can also accept additional controls to tailor the output, such as main eye gaze direction, head-to-camera distance, and a general emotion offset.
Overall framework
Instead of directly generating video frames, VASA-1 works in two stages:
Motion and Pose Generation: It creates a sequence of codes that represent the facial dynamics (lip movements, expressions) and head movements (pose) conditioned on the audio and other input signals.
Video Frame Generation: These motion and pose codes are then used to generate the actual video frames, taking into account the appearance and identity information extracted from the input image.
Technical Breakdown
Here’s a breakdown of the core components of VASA-1:
1. Expressive and disentangled face latent space construction
VASA-1 starts by building a special kind of digital space called a “latent space” specifically designed for representing human faces. This space has two key properties:
- Expressiveness: It can capture the full range of human facial expressions and movements with high detail.
- Disentanglement: Different aspects of the face, such as identity, head pose, and facial dynamics, are represented separately in this space. This allows for independent control over these aspects during video generation.
VASA-1 achieves this by building upon existing 3D face reenactment techniques. It decomposes a face image into several components:
- 3D Appearance Volume (Vapp): This captures the detailed 3D shape and texture of the face.
- Identity Code (z_id): This represents the unique characteristics of the person in the image.
- Head Pose Code (z_pose): This encodes the orientation and tilt of the head.
- Facial Dynamics Code (z_dyn): This captures the current facial expressions and movements.
To ensure proper disentanglement, VASA-1 employs specialized loss functions during training. These functions penalize the model if it mixes up different aspects of the face representation.
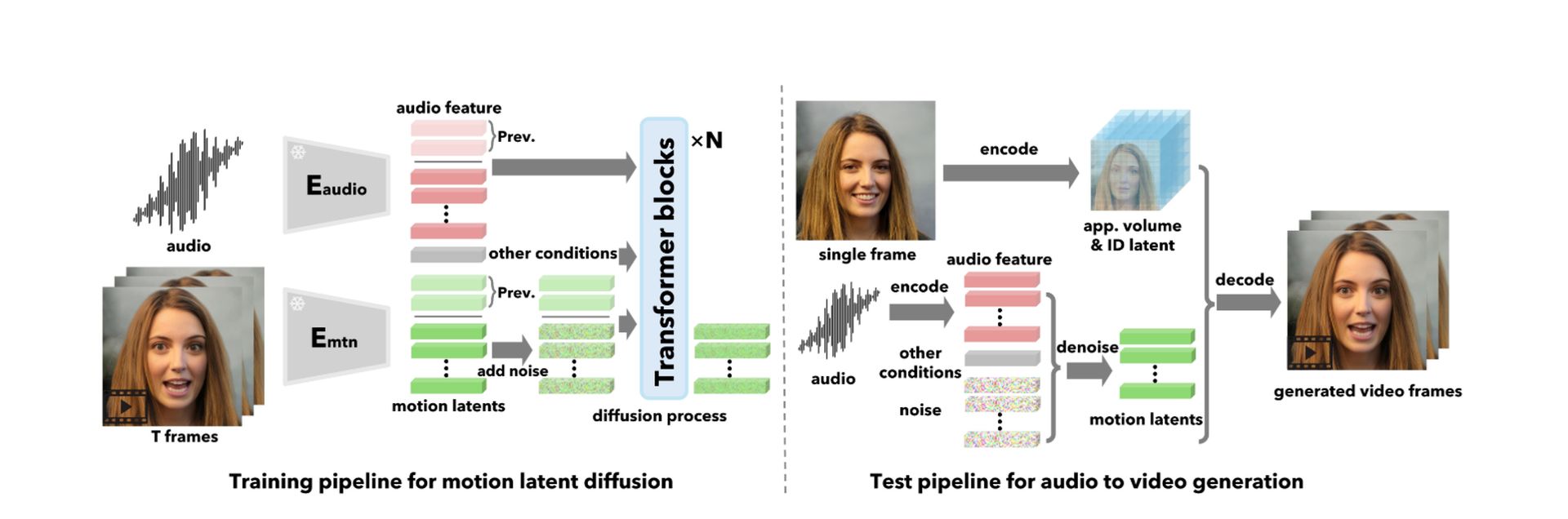
2. Holistic facial dynamics generation with diffusion transformer
Once VASA-1 has a well-trained latent space, it needs a way to generate the motion and pose codes for a talking face sequence based on an audio clip. This is where the “Diffusion Transformer” comes in.
- Diffusion model: VASA-1 leverages a diffusion model, a type of deep learning architecture, to achieve this. Diffusion models work by gradually adding noise to a clean signal and then learning to reverse this process. In VASA-1’s case, the clean signal is the desired motion and pose sequence, and the noisy signal is a random starting point. The diffusion model essentially learns to “de-noise” its way back to the clean motion sequence based on the provided audio features.
- Transformer architecture: VASA-1 utilizes a specific type of diffusion model called a “transformer”. Transformers excel at sequence-to-sequence learning tasks, making them well-suited for generating a sequence of motion and pose codes corresponding to the audio sequence.
VASA-1 conditions the diffusion transformer on several inputs:
- Audio features: Extracted from the audio clip, these features represent the audio content and inform the model about the intended lip movements and emotions.
- Additional control signals: These optional signals allow for further control over the generated video. They include:
- Main eye gaze direction (g): This specifies where the generated face is looking.
- Head-to-camera distance (d): This controls the apparent size of the face in the video.
- Emotion offset (e): This can be used to slightly alter the overall emotional expression displayed by the face.
3. Talking face video generation
With the motion and pose codes generated, VASA-1 can finally create the video frames. It does this by:
- Decoder Network: This network takes the motion and poses codes along with the appearance and identity information extracted from the input image as input. It then uses this information to synthesize realistic video frames that depict the person in the image making the facial movements and expressions corresponding to the audio.
- Classifier-free Guidance (CFG): VASA-1 incorporates a technique called Classifier-free Guidance (CFG) to improve the robustness and controllability of the generation process. CFG involves randomly dropping some of the input conditions during training.
This forces the model to learn how to generate good results even when not all information is available. For example, the model might need to generate the beginning of the video without any preceding audio or motion information.
See further explanation in the research paper here.
The looming shadow of deepfakes
Deepfakes, highly realistic AI-generated videos that manipulate people’s appearances and voices, have become a growing source of concern. Malicious actors can use them to spread misinformation, damage reputations, and even sway elections. VASA-1’s hyper-realistic nature intensifies these anxieties.
Herein lies the crux of VASA-1’s uncertain future.
Microsoft’s decision to restrict access, keeping it away from both the public and some researchers, suggests a cautious approach. The potential dangers of deepfakes necessitate careful consideration before unleashing such powerful technology.
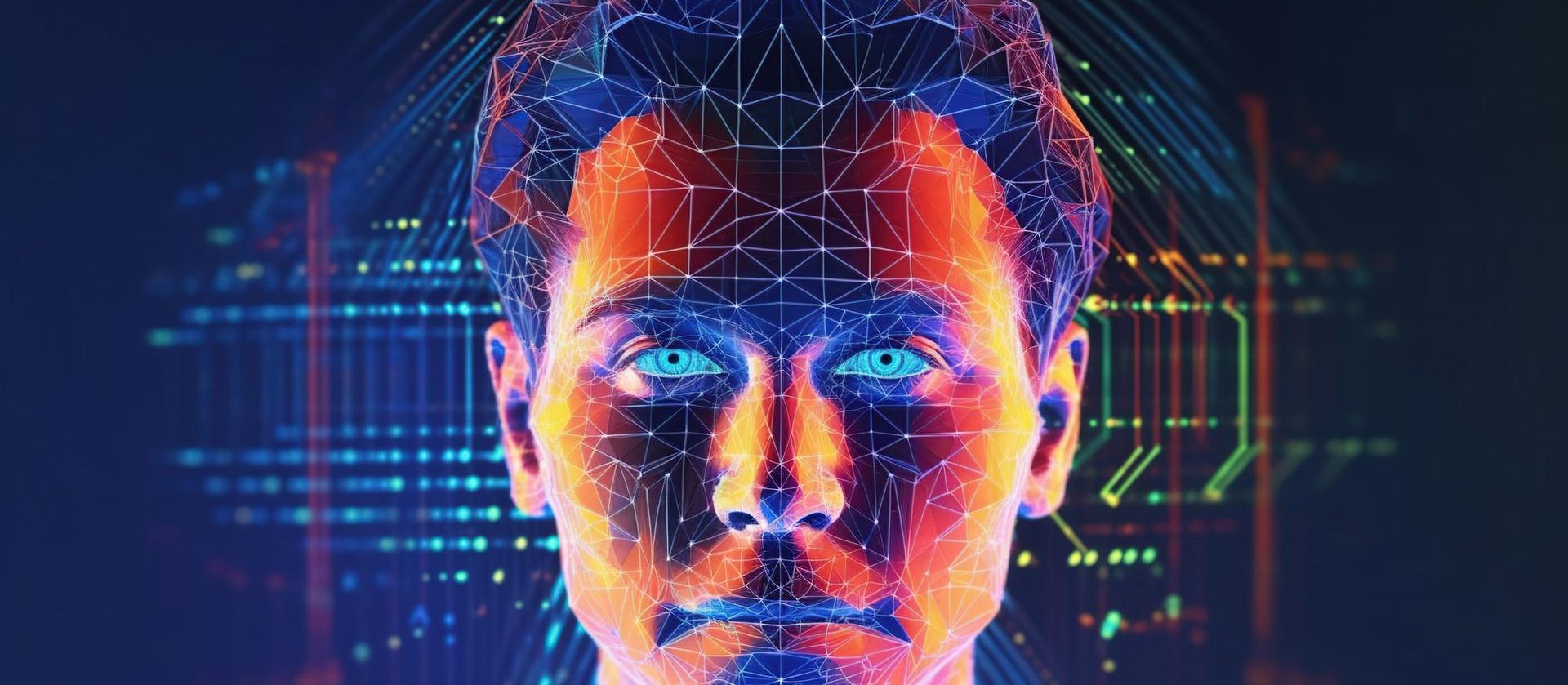
Balancing innovation with responsibility
Moving forward, Microsoft faces a critical challenge: balancing innovation with responsible development. Perhaps the path forward lies in controlled research environments with robust safeguards against misuse. Additionally, fostering public education and awareness about deepfakes can empower users to discern genuine content from AI-manipulated ones.
VASA-1 undeniably represents a significant leap in AI’s ability to manipulate visual media. Its potential applications are nothing short of revolutionary.
However, the ethical considerations surrounding deepfakes necessitate a measured approach. Only through responsible development and public education can we unlock the true potential of VASA-1 while mitigating the potential for harm.
Featured image credit: Microsoft