A recent investigation by Anthropic has revealed a new method for circumventing the protective measures of LLMs, termed “many-shot jailbreaking.” This approach exploits the extensive context windows utilized by cutting-edge LLMs to steer the models towards generating responses that are potentially dangerous or harmful.
The advancement of large language models brings with it increased avenues for misuse…
New Anthropic research paper: Many-shot jailbreaking.
We study a long-context jailbreaking technique that is effective on most large language models, including those developed by Anthropic and many of our peers.
Read our blog post and the paper here: https://t.co/6F03M8AgcA pic.twitter.com/wlcWYsrfg8
— Anthropic (@AnthropicAI) April 2, 2024
What really is many-shot jailbreaking?
The essence of many-shot jailbreaking involves inundating the model with numerous question-answer pairs that demonstrate the AI providing unsafe or harmful answers. By employing hundreds of such instances, perpetrators can effectively bypass the model’s safety protocols, leading to the production of unwelcome content. This flaw has been identified in not just Anthropic’s models but also in those created by leading AI entities like OpenAI.
At its core, many-shot jailbreaking leverages the concept of in-context learning, where a model tailors its responses based on the input examples given in its immediate environment. This connection indicates that devising a strategy to counter such tactics without adversely affecting the model’s ability to learn is a complex challenge.
This technique exploits the extensive context windows of advanced LLMs, enabling manipulative prompts to bypass the models’ ethical and safety guidelines, leading to potentially harmful outcomes.
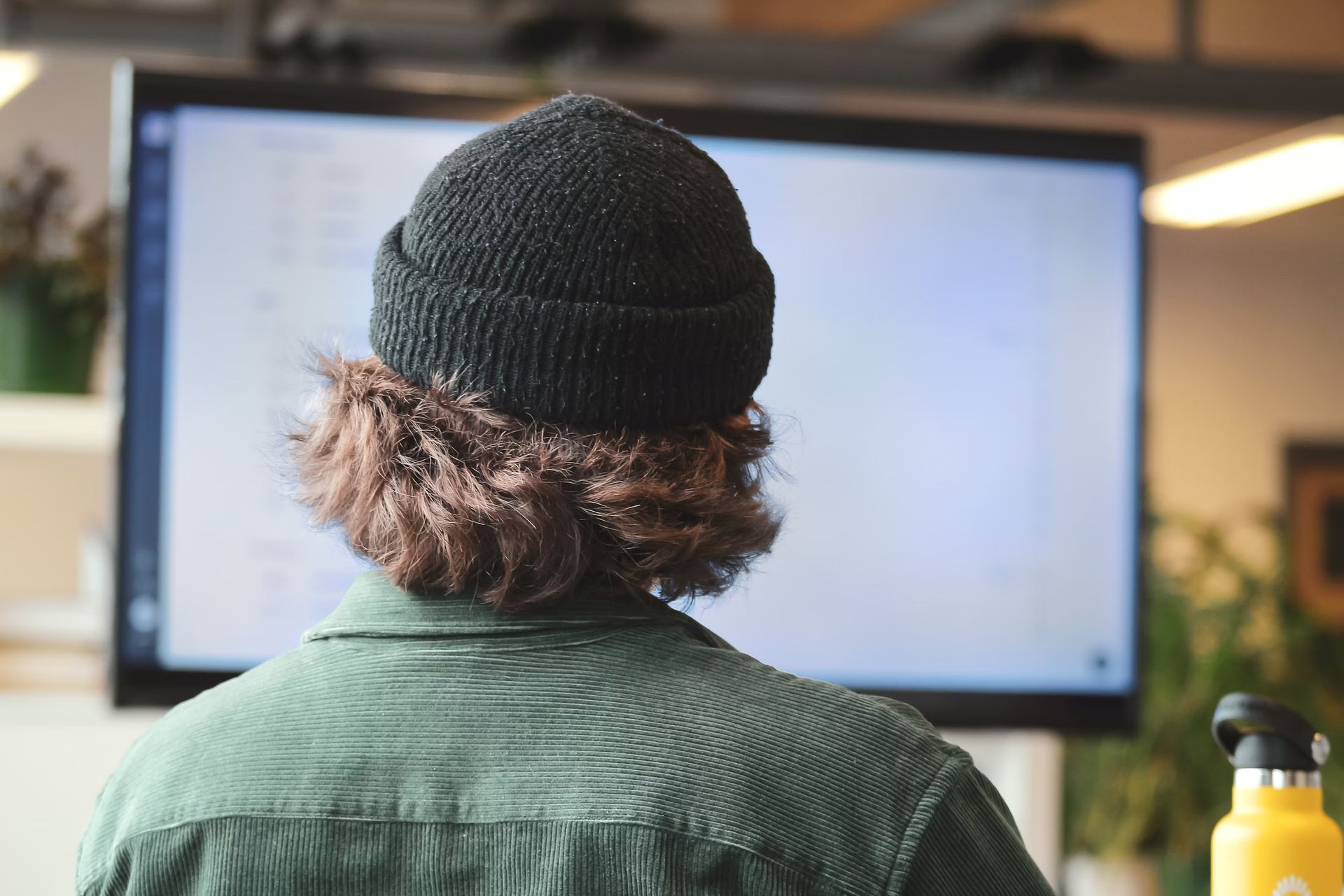
The crux of this technique lies in its use of numerous examples of undesirable behavior within a single prompt, leveraging the vast context capabilities of modern LLMs to encourage them to replicate this behavior. This is a significant departure from previous approaches that relied on shorter contexts, marking a worrying evolution in the sophistication of attacks against AI safety measures.
This study specifically targeted top-tier LLMs, including Claude 2.0, GPT-3.5, GPT-4, Llama 2, and Mistral 7B, across a range of tasks. The findings were alarming; with sufficient ‘shots’ or examples, these models began displaying a wide array of undesired behaviors, such as issuing insults or instructions for creating weapons. The effectiveness of these attacks scaled predictably with the number of examples provided, underscoring a profound vulnerability in LLMs to this new form of exploitation.
Amazon invests whooping $4B in AI venture Anthropic
The research sheds light on the scaling laws of in-context learning, suggesting that as the number of manipulative examples increases, the likelihood of a model producing harmful content does too, following a power-law distribution. This relationship holds across different tasks, model sizes, and even with changes in the prompt’s format or style, indicating a robust and versatile method for circumventing LLM safety protocols.
Critically, the study also explored various mitigation strategies, including standard alignment techniques and modifications to the training data. However, these approaches showed limited effectiveness in curbing the potential for harmful outputs at scale, signaling a challenging path ahead for securing LLMs against such sophisticated attacks.
Featured image credit: Markus Spiske/Unsplash