Artificial intelligence (AI) has transformative potential. But as with any potent technology, the data quality of its input directly impacts its output.
Foundational, a company recently out of stealth mode, understands this crucial point.
Armed with $8 million in fresh funding, they’re aiming to tackle the often-overlooked problems of data quality and AI readiness.
Why data quality is a big deal for AI?
We’ve all heard the adage “garbage in, garbage out“.
This applies with special force to AI models. These models learn from vast datasets during the training process. If the data they’re fed is inconsistent, incomplete, biased, or simply wrong, the AI’s output will likely reflect those flaws. In high-stakes fields like healthcare or finance, inaccurate outputs due to poor data can have disastrous consequences.
Foundational’s approach focuses on improving the reliability and integrity of datasets used for AI training and operation. This is vital work, ensuring that AI models produce the best and safest possible results.
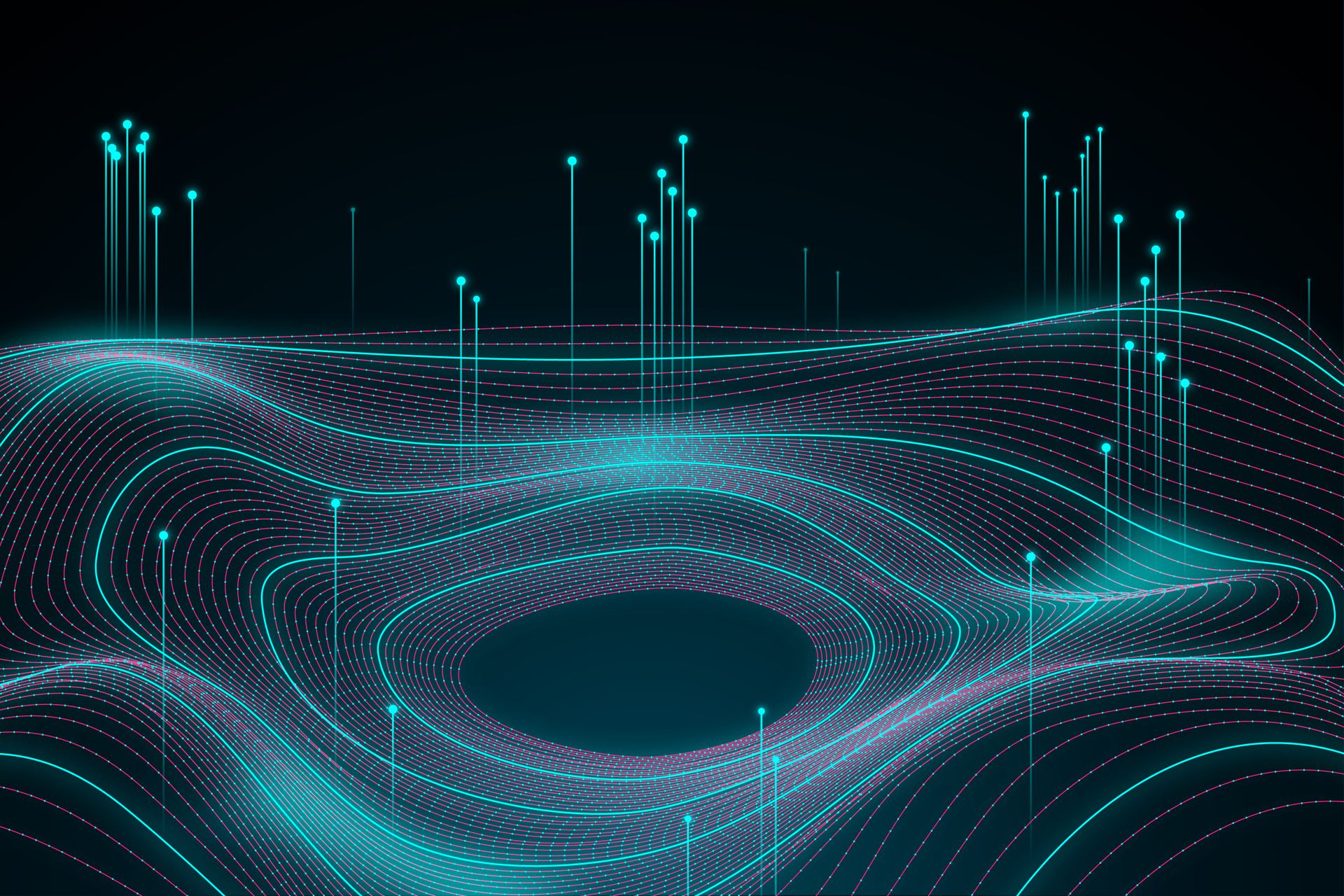
Getting data AI-ready
“They will see our insights or warnings or suggestions directly in the interface that they already have,” explains Foundational’s CEO, Michelangelo Nafta to VentureBeat. Importantly, the platform works by scrutinizing the metadata within the code itself. It sidesteps direct contact with the sensitive data, reducing privacy and security risks.
The Foundational platform integrates seamlessly with tools like GitHub, offering developers actionable feedback within their existing workflow.
The power of analysis
Foundational harnesses a combination of techniques to build a detailed map of an organization’s data flow:
- Static code analysis: The platform dissects code structure to uncover relationships and dependencies
- Dynamic runtime analysis: Monitors code execution to identify real-world data patterns and potential bottlenecks
- AI-powered techniques: These help to make connections, spot anomalies, and identify optimization opportunities
This comprehensive understanding becomes the foundation for powerful automation. “Once we have this full map of your data ecosystem, there are all kinds of powerful automation we can apply on top,” Nafta states. Notifications about potential downstream disruption due to code changes, performance optimization tips, and even automated generation of documentation and data catalogs are all within reach.
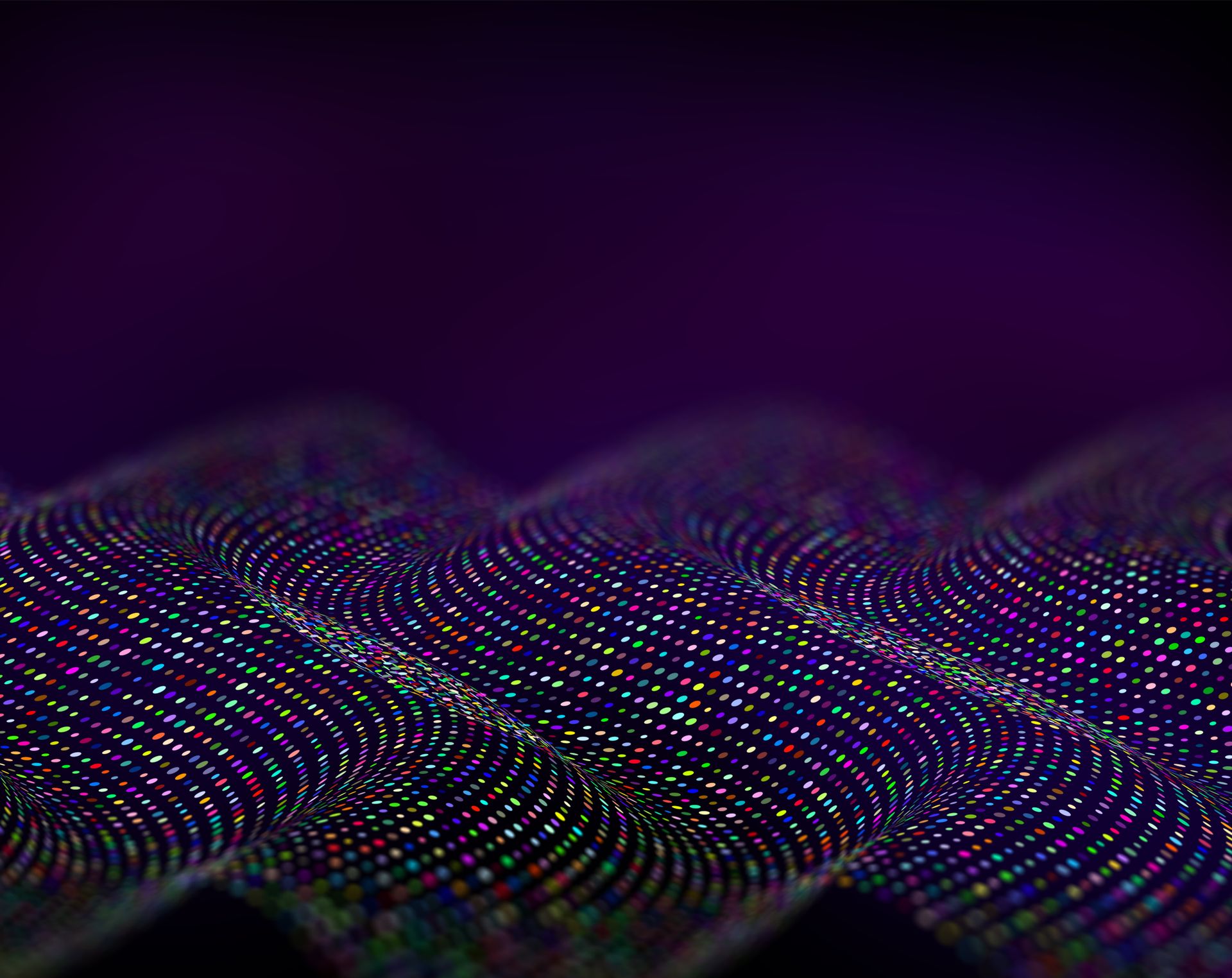
Streamlining more than data
Foundational’s approach offers advantages beyond data quality alone. It targets potential problems like circular references and cloud-cost spiking queries, addressing cost efficiency alongside accuracy. Additionally, by identifying unused fields, the platform promotes leaner, more maintainable data pipelines.
The people problem
Foundational recognizes that technology alone won’t solve AI’s data woes. They offer a strong focus on collaboration with domain experts. It’s these experts who understand the nuances of their field’s specific datasets; this partnership allows for fine-tuning solutions and ensuring they align with real-world needs.
Governance by design
Foundational positions code analysis as a pillar of proactive data governance. In an era of ever-expanding datasets and increasingly complex AI, a tool that helps maintain data health by design is a valuable asset. The company’s emphasis on developer-friendly integration and focus on metadata privacy are also shrewd moves, likely to build confidence in their approach.
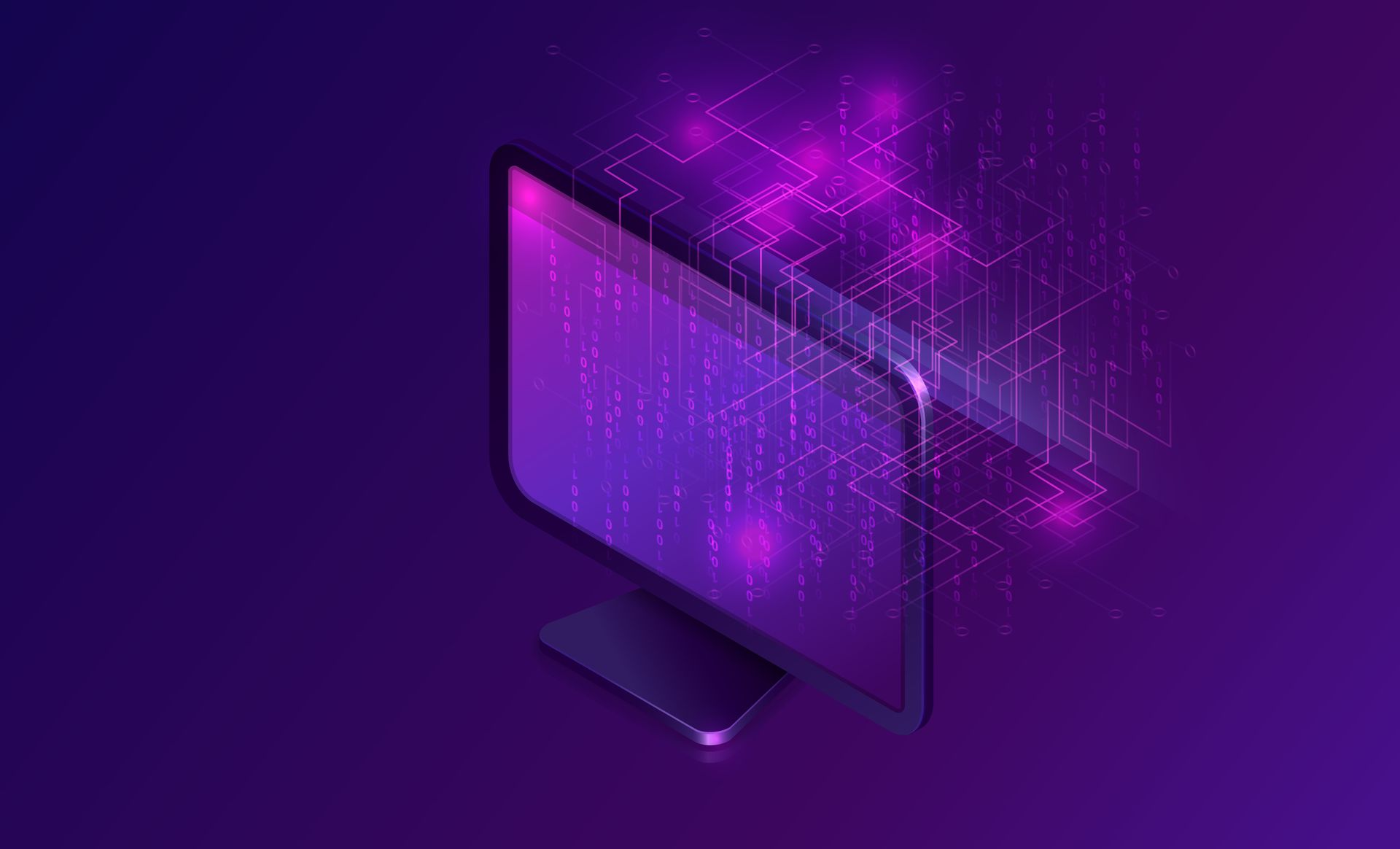
The road ahead is accepting data quality as a baseline
The emergence of companies like Foundational signals a welcome shift in the industry. It highlights an increasing awareness of data quality as a non-negotiable prerequisite for effective AI deployment. As organizations grapple with growing volumes and complexity of data, services facilitating accurate and trustworthy AI models will be in high demand.
Foundational’s entry into this arena is timely. Businesses can no longer afford to treat AI projects as purely technological endeavors. By placing data quality at the forefront, Foundational is poised to make a meaningful impact on the success, and safety, of AI applications across industries.
Featured image credit: rawpixel.com/Freepik