Google has rolled out an innovative AI framework termed “Social Learning,” designed to boost the collaborative prowess of language models while safeguarding user privacy. This new framework permits AI models to engage in natural language exchanges, sharing insights and enhancing their collective ability on diverse tasks without breaching privacy.
- The concept draws inspiration from Bandura and Walters’ 1977 theory of social learning, emphasizing learning through observation, either by verbal instruction or by mimicking observed behaviors.
- Unlike traditional collaborative learning methods that may involve direct data sharing, this approach enables LLMs to teach and learn from each other using natural language, circumventing privacy concerns.
- The paper discusses the framework’s application across various tasks, including spam detection, solving grade school math problems, and text-based question answering, showcasing its versatility.
“Large language models (LLMs) have significantly improved the state of the art for solving tasks specified using natural language, often reaching performance close to that of people. As these models increasingly enable assistive agents, it could be beneficial for them to learn effectively from each other, much like people do in social settings, which would allow LLM-based agents to improve each other’s performance.”
How does Google’s Social Learning work?
Within the Social Learning setup, “teacher” models convey knowledge to “student” models without the need to transmit sensitive or private information, striking a balance between effective learning and privacy preservation. These student models draw lessons from a variety of teacher models, each specialized in particular tasks like detecting spam, solving mathematical puzzles, or providing answers based on textual content.
By utilizing examples vetted by humans, teacher models can educate student models without exchanging the original data, thereby addressing the privacy concerns linked to data sharing. Furthermore, teacher models have the ability to craft new scenarios or create specific task instructions, enriching the educational journey.
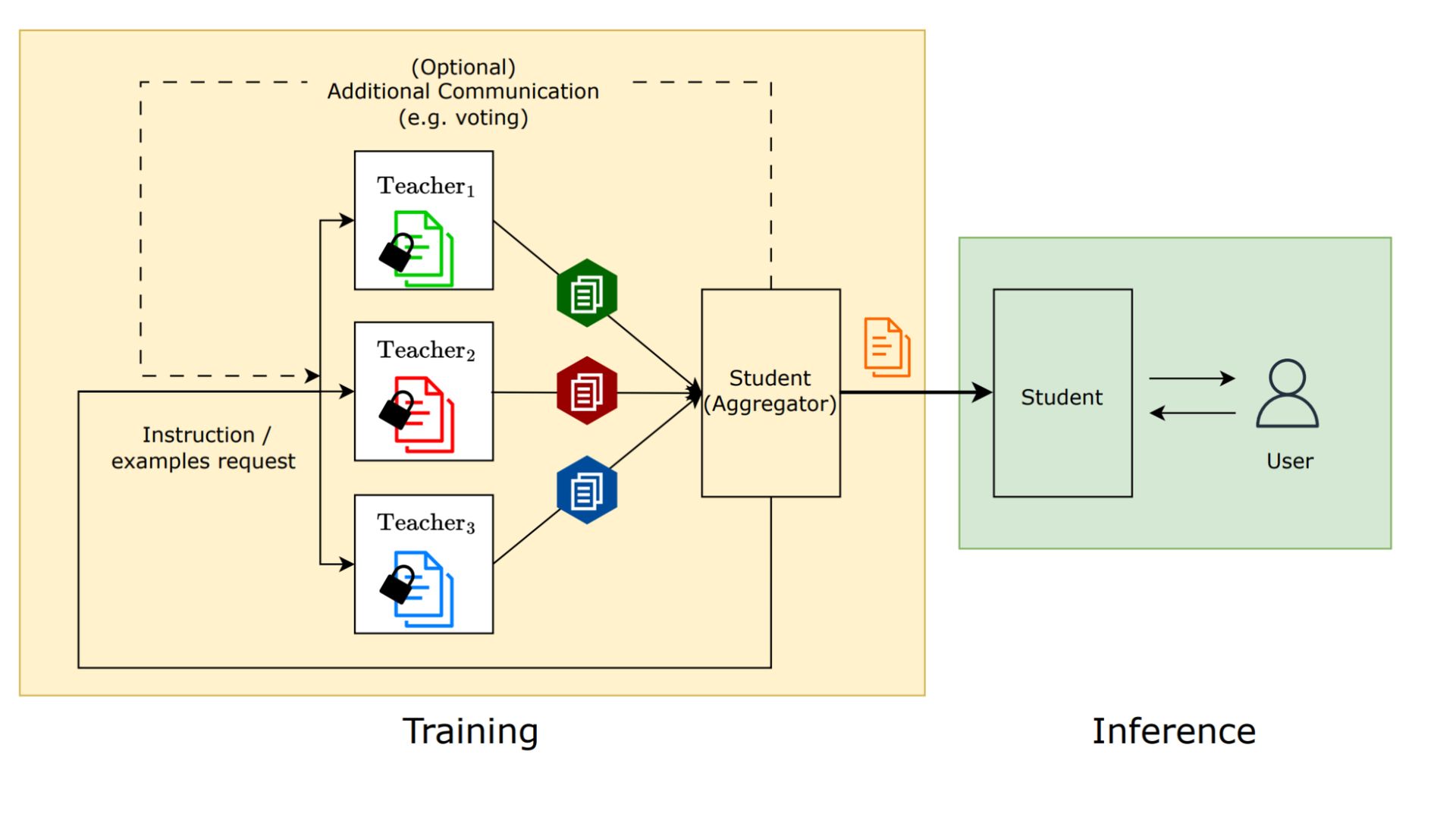
Research trials underscore the success of social learning in elevating student models’ competencies across a range of tasks. The synthetic scenarios concocted by teacher models have been found just as impactful as the authentic data, all while drastically diminishing privacy hazards. Likewise, task instructions fashioned by teacher models have been instrumental in boosting student model performances, showcasing the language models’ versatility in adhering to directives.
To anchor privacy protection, the research community has adopted measures like the Secret Sharer metric to evaluate the extent of data exposure during the learning phase. The findings reveal negligible privacy data seepage, validating the framework’s capacity to instruct without divulging details from the original data pool.
How AI improves education with personalized learning at scale and other new capabilities
By emulating the human process of social learning, these models are adept at exchanging know-how and collectively advancing their capabilities, all while prioritizing user privacy. This strategy opens new avenues for the creation of privacy-conscious AI technologies across varied fields. Looking ahead, the research teams are set on further polishing the Social Learning framework and delving into its utility in assorted tasks and data contexts.
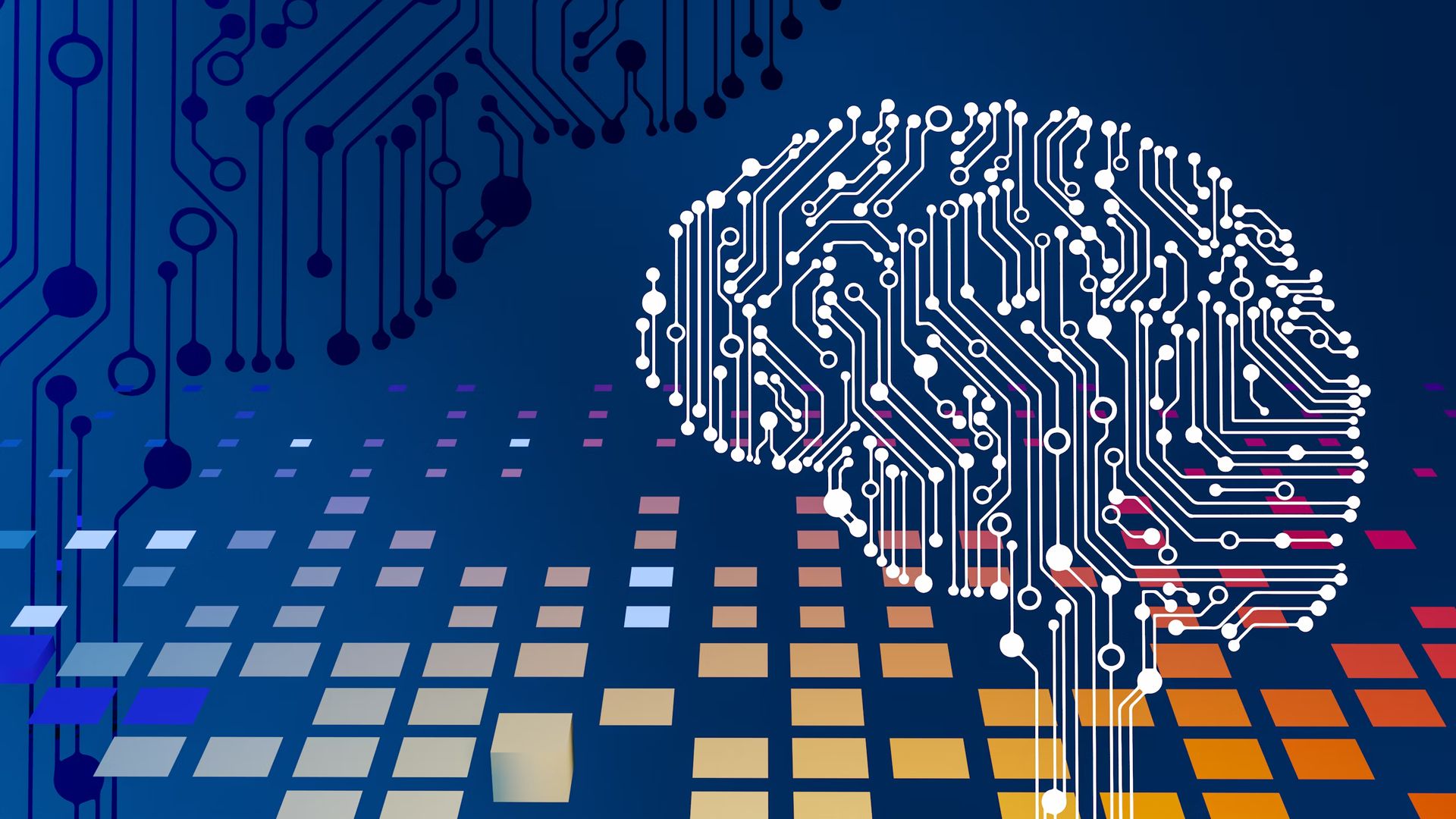
“We introduced a framework for social learning that allows language models with access to private data to transfer knowledge through textual communication while maintaining the privacy of that data. In this framework, we identified sharing examples and sharing instructions as basic models and evaluated them on multiple tasks. Furthermore, we adapted the Secret Sharer metric to our framework, proposing a metric for measuring data leakage,” Google stated in its blog post.
“As next steps, we are looking for ways of improving the teaching process, for example by adding feedback loops and iteration. Furthermore, we want to investigate using social learning for modalities other than text,” the blog post further reads.
Featured image credit: Mitchell Luo/Unsplash