Could artificial intelligence (AI) be the answer to a devastating disease like Alzheimer’s? New research suggests that the answer may be a resounding yes.
Alzheimer’s disease is a progressive neurodegenerative disorder that slowly erodes memory, thinking skills, and the ability to perform everyday tasks. It is the most common form of dementia and has been a major healthcare challenge worldwide for over 100 years. The heartbreaking reality is that there is no current cure for Alzheimer’s.
One of the most significant issues with Alzheimer’s is that by the time symptoms are clear enough for a diagnosis, the disease has already done substantial damage to the brain. This delay greatly complicates effective treatment.
Thankfully, a groundbreaking study has revealed that AI predicts Alzheimer’s disease up to seven years before noticeable symptoms emerge. Let’s take a deep dive into this revolutionary finding and what it implies for the future of Alzheimer’s detection and treatment.
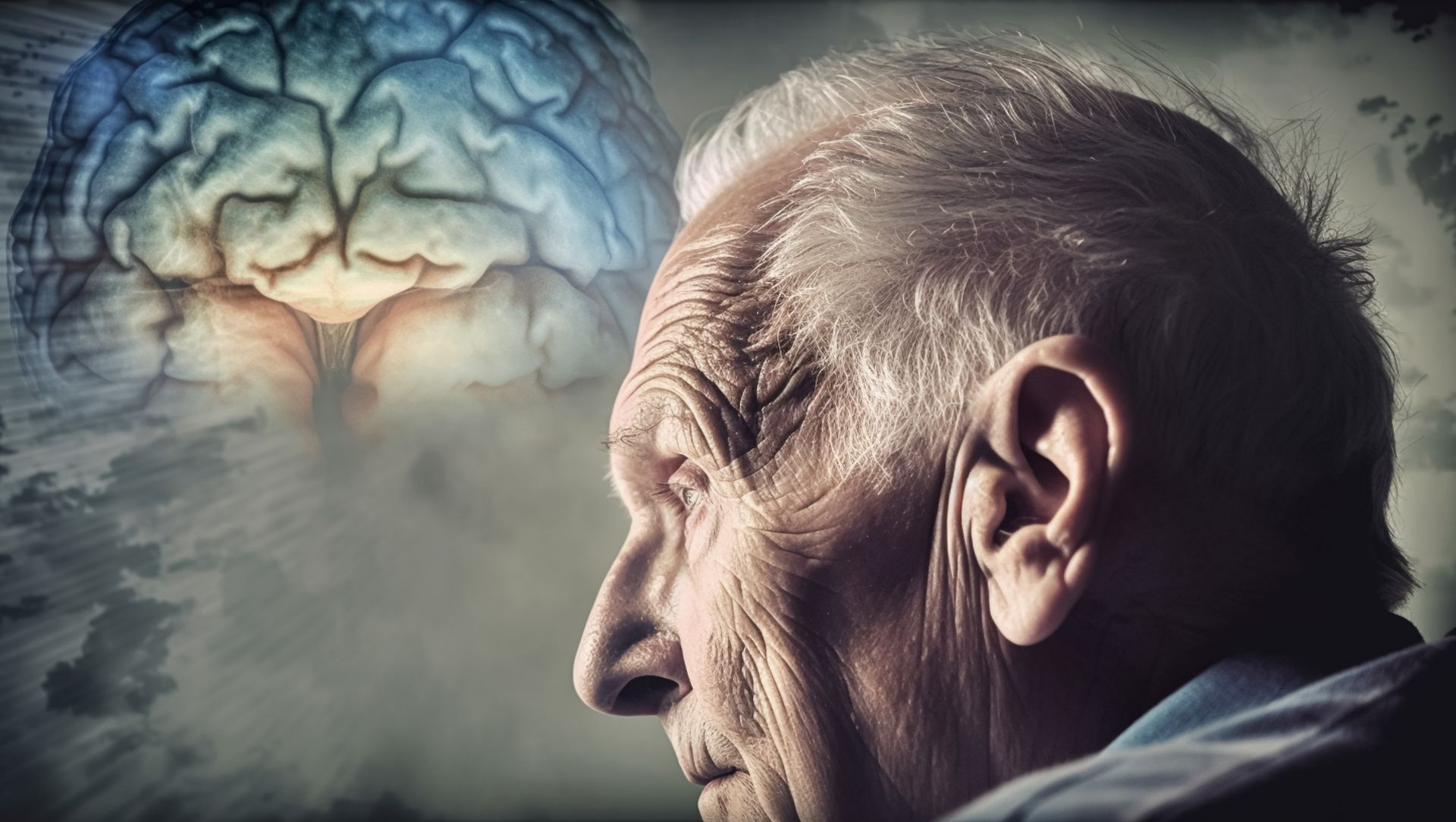
AI can be the game changer in the early detection of Alzheimer’s
Machine learning, a field of artificial intelligence, allows computers to learn and identify patterns from massive quantities of data. Researchers are leveraging this strength to train AI algorithms on vast datasets of medical information, including brain scans, cognitive tests, and genetic data. These AI models pick up on subtle changes and patterns associated with Alzheimer’s disease long before traditional diagnostic methods.
A recent study published in Nature Aging highlights the incredible potential of AI to predict Alzheimer’s. Researchers at the University of California, San Francisco (UCSF) developed an AI algorithm that successfully predicted Alzheimer’s disease with a noteworthy 72% accuracy up to seven years in advance. The findings suggest that AI models can detect signs of the disease much earlier than standard diagnostic tools.
How did they achieve such remarkable success?
The researchers used a type of study design called a retrospective cohort study. This means they looked back on existing historical data from electronic health records (EHRs).
They collected a wide variety of data from EHRs, including:
- Brain scans: Different types of brain scans can show changes associated with Alzheimer’s
- Cognitive tests: Tests evaluating memory, thinking, and problem-solving abilities
- Diagnoses from doctors: Previous diagnoses with conditions that may be linked to Alzheimer’s risk
- Demographic info: Age, sex, education, etc
This study primarily used Random Forest (RF) models. Imagine a collection of decision trees working as a team to make a “diagnosis”. Each tree asks a series of questions about the patient’s health data, and their combined answers lead to a prediction about their Alzheimer’s risk.
To train the AI models, researchers fed them a large dataset containing patients both with and without Alzheimer’s. This teaches the AI the patterns in the data that signal Alzheimer’s risk. Afterwards, the model’s performance is tested on a completely separate “held-out” dataset. Since the AI has never seen this data before, it shows how well it has actually learned to predict Alzheimer’s in new patients.
To assess how well the model does, researchers use metrics like AUROC and AUPRC. AUROC measures how good the model is at telling the difference between those who will and won’t develop Alzheimer’s. AUPRC focuses on how many of the model’s positive predictions (saying someone will get Alzheimer’s) are actually correct.
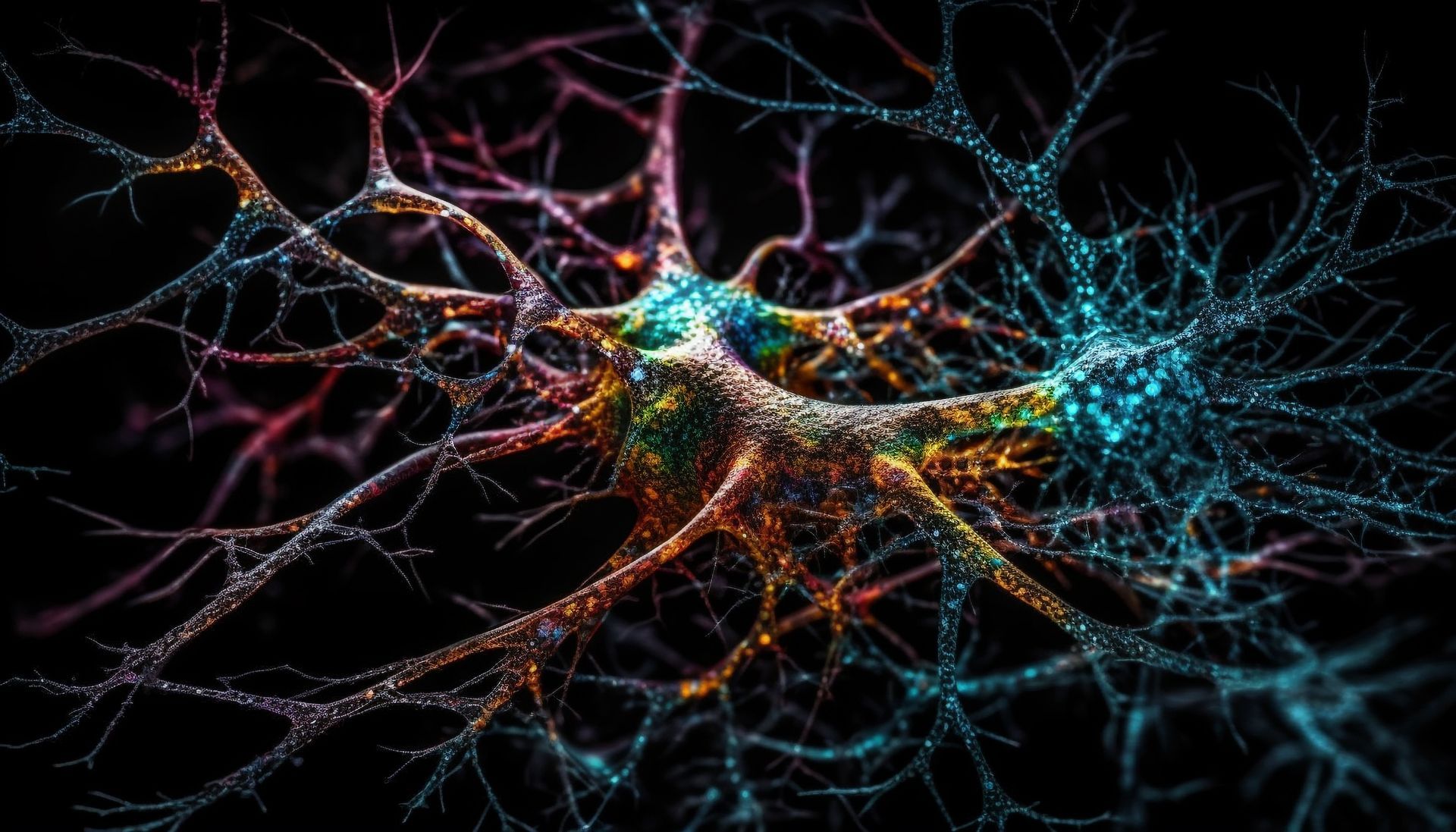
Importantly, the researchers took these findings further and validated them:
- HLD and APOE: They looked at other, large EHR datasets and confirmed that people with Hyperlipidemia (HLD) had a greater risk of developing Alzheimer’s. Further, the APOE gene (a known Alzheimer’s risk factor) had variants linked to both HLD and Alzheimer’s
- Osteoporosis link: They found women with osteoporosis in other datasets also had faster progression to Alzheimer’s. They further identified a link to the MS4A6A gene in women that influences both bone density and Alzheimer’s risk
The researchers also shared all the details about the code of the algorithm used publicly in a GitHub post titled ”Leveraging Electronic Health Records and Knowledge Networks for Alzheimer’s Disease Prediction and Sex-Specific Biological Insights”.
AI’s undeniable potential
These techniques, while specifically used for Alzheimer’s prediction here, showcase the undeniable potential of AI in medicine. Imagine a future where AI can help doctors sift through mountains of complex medical data, identifying subtle patterns that humans might miss. This could lead to earlier diagnoses across a range of diseases, more personalized treatment plans, and potentially even ways to prevent illnesses before they start.
However, while the promise is enormous, so are the challenges. We need massive amounts of high-quality data to train reliable AI models. We must carefully address privacy concerns and ensure AI doesn’t worsen existing healthcare disparities. Most importantly, AI should remain a powerful tool in the hands of doctors, aiding their judgment, not replacing it.
The study we’ve discussed is a significant step forward. It shows how responsibly developed AI can unlock the potential hidden within our own medical records, ultimately leading to better health outcomes for us all.
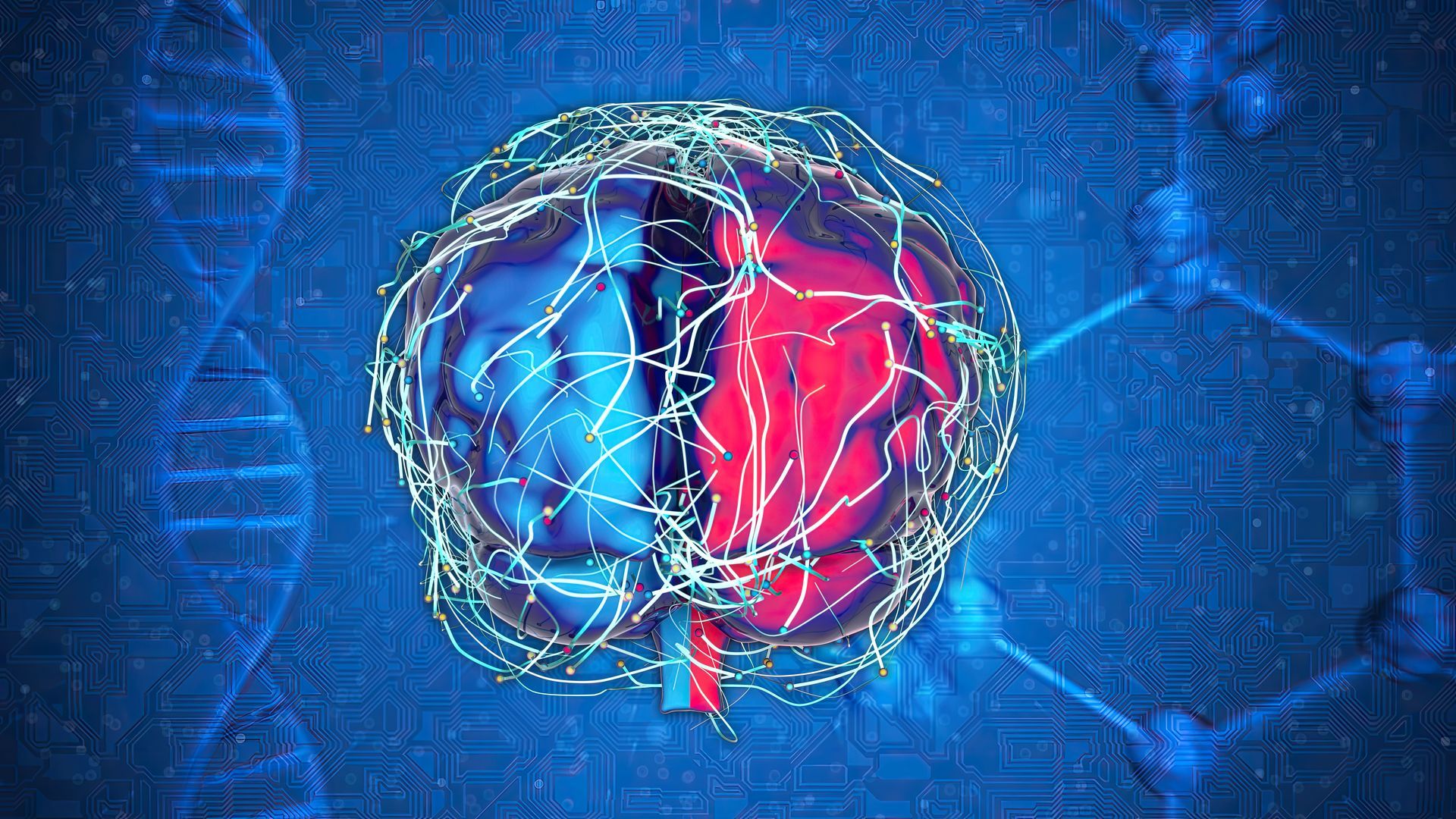
What if…
While studies like this highlight AI’s potential, it’s essential to acknowledge the risks involved when technology this powerful meets the sensitive domain of healthcare. Misleading results from poorly trained AI models could lead to misdiagnoses, inappropriate treatments, and potentially even patient harm. Moreover, the black-box nature of some AI algorithms means it can be hard to know why they arrive at certain conclusions, making it harder for doctors to trust and integrate the results into their decision-making.
There’s also the looming issue of privacy. Medical data is incredibly valuable and vulnerable as we have seen in the recent Change Healthcare cyberattack case. AI systems need massive datasets to learn, raising concerns about data breaches and the use of such sensitive information without proper patient consent. Additionally, AI could worsen existing disparities in healthcare. If AI models are trained on biased or incomplete datasets, they might perpetuate those biases, leading to less effective care for certain populations.
It’s vital to not let the excitement over AI overshadow these very real risks. Responsible development means rigorous testing, transparency in how AI models work, and safeguards for patient privacy and fairness. Only then can we confidently harness AI’s potential while minimizing the potential for harm.
Featured image credit: atlascompany/Freepik.