One of the most significant challenges present in project management is the variety of ways that a project can be managed and handled. With different teams, it may be necessary to adopt several different methodologies to get the most efficient outcome for your team.
When contemporary businesses are increasingly driven by data, project managers must understand how the intersection between team members, data, and strategies can come together. Sometimes, it’s assumed that the role of data science and project management is much the same – while data can help inform decisions, it’s not typically a field that exclusively runs projects.
Regardless of whether you’re an experienced data scientist or a student completing a Master of Project Management, the differences between data science and project management must be well understood before undertaking any major project. Let’s take a moment to explore how data can complement contemporary project methodologies to get the best practical outcome out of a project with the available data.
Data-driven decision making – Transforming projects
The introduction of modern data collection through digital systems has increasingly transformed the way that data can be used to inform decision-making. Take, for example, the Census, a national demographic survey conducted every five years by the Australian Bureau of Statistics. Initially tabulated using mechanical machine equipment, it has evolved with the introduction of computer technology in 1966 to increasingly online Census participation in the current era.
The way data is collated, stored, and analysed can help transform the way that projects are planned and implemented. Instead of waiting multiple years to take on a plan, adept data science teams use their knowledge to provide rapid, meaningful, and useful insights to project managers, helping align priorities with the data that is available and known.
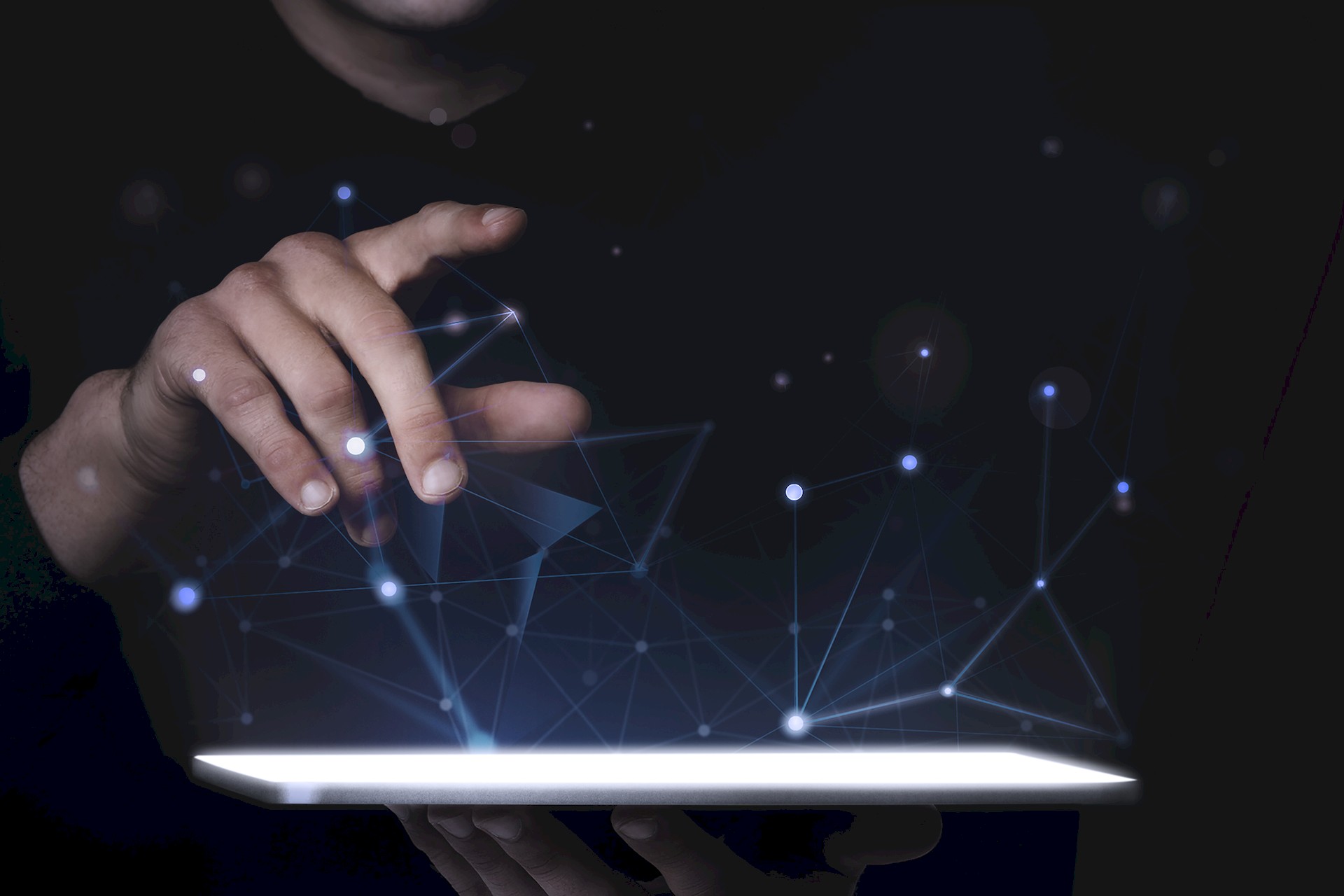
Key stages of The data science lifecycle
There are a number of stages that are essential to the lifecycle of any data science project. After all, while data is useful, it’s important that meaning is extracted from raw data inputs. With an estimated 120 billion terabytes of data generated annually, according to the latest reports, it’s important to understand that raw data is, by itself, not particularly useful without some form of analysis.
Three key stages of the data science lifecycle include data mining, cleaning, and exploration. These processes are vital for any data science project – and skipping any one of these steps can be potentially perilous when undertaking data projects.
Firstly, data mining takes an understanding of operational requirements to dig into potential data sources. For example, a project that seeks to understand the relative performance of a mailout program may seek to gather information on returned mail, payments from contacted customers, as well as financial information, such as the cost to mail or return a flyer.
Data cleaning is another crucial stage of the data science lifecycle. Data on its own may be raw and untidy – for example, a data source with addresses may include data structured in different or historical formats, meaning that any exploration conducted without cleaning the structure of the data first could be potentially misleading or wrong.
Once data mining and cleaning are undertaken, comprehensive data exploration must be done. Data-driven outcomes don’t happen straight away – sometimes it can take days or even weeks of digging into data to understand how data ties together. The outcomes found in this discovery stage can then be used to inform further investigation and complement the project delivery design phase.
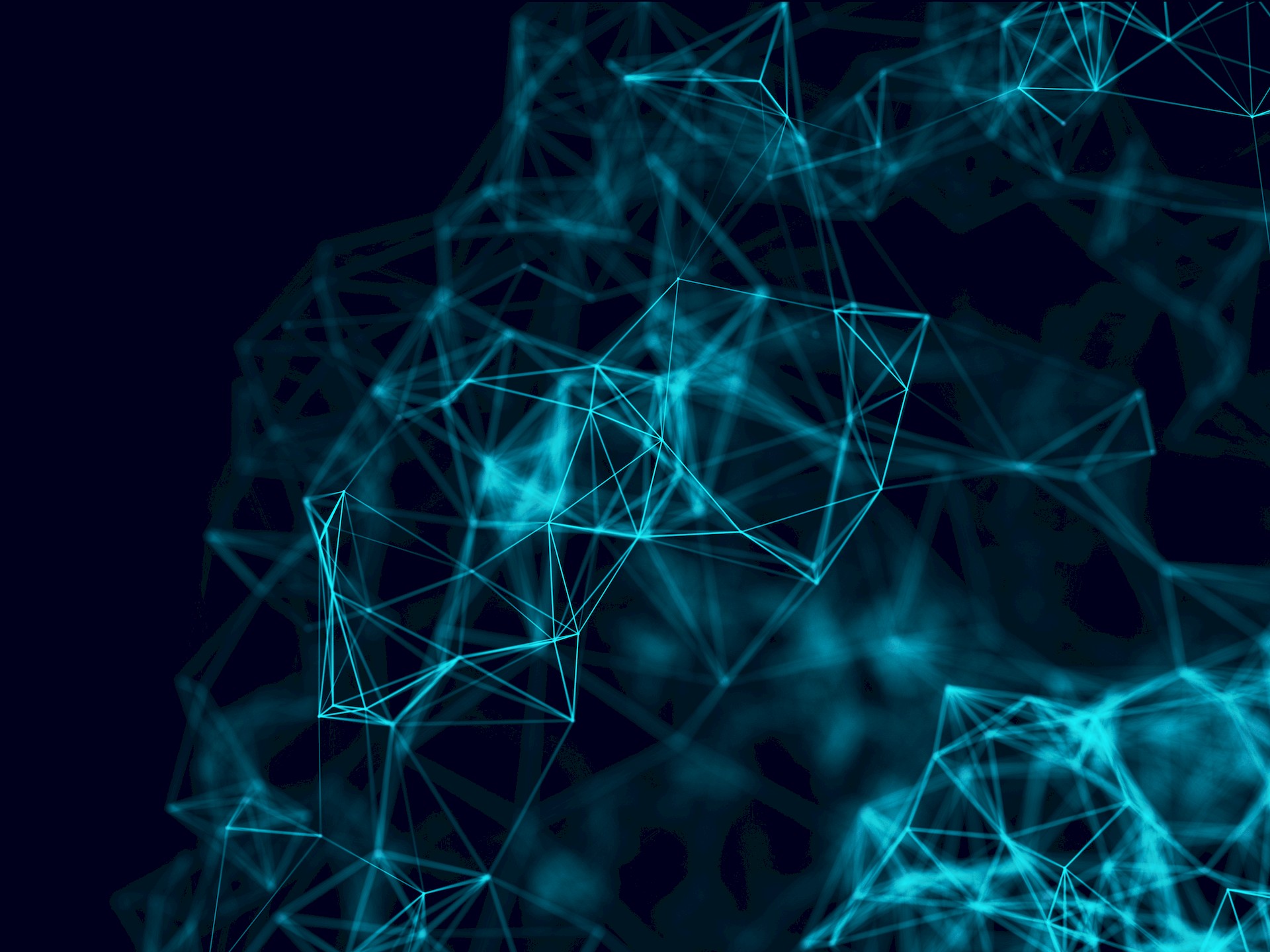
Common project management methodologies
There are many different project management methodologies. Traditional methods such as the waterfall method are well known. However, more recent methodologies such as the agile method have gained prominence in recent years as a way of evolving the way that projects are managed, in alignment with improved data availability.
A development methodology common in projects is known as the waterfall methodology. This orthodox strategy, common in software development, involves a five-step process (Requirements, Design, Implementation, Testing, Deployment) where steps are taken sequentially. While this may be useful for some projects, it is sometimes seen as difficult to manage when working with data-supported projects.
A contemporary methodology that commonly appears when working with rapidly evolving data is known as the agile methodology. This method allows for rapid repositioning as corporate requirements change – and is typically considered best practice when working on projects that require constant pivoting or adjustment to manage business needs.
The Intersection of Project Management and Data Science
Project management and data science can intersect in interesting ways – much like Ouroboros, the increasingly symbiotic relationship between project management and data science can make one wonder which was around first.
For the adept project leader, being able to understand which combination of project methodology and data science strategy is best can go a long way toward informing strategic decision-making. This, in turn, can help align current or future project goals – transforming project management from being solely reliant on business requirements to something that is far more fluid and versatile. With data and project management so closely intertwined, it’s exciting to imagine what these two roles will bring together in the years ahead.
Featured image credit: kjpargeter/Freepik.