Generative artificial intelligence (AI) is arguably at risk of being pigeonholed. Despite the likes of McKinsey producing detailed charts and graphs illustrating its vast scope, noise around conversational bots is drowning out wider discussions — with nearly every business from law firms to travel agencies building chat tools; including Facebook’s newly launched “sassy” GenAI personas, aimed at engaging GenZ audiences via tongue-in-cheek interactions.
While these applications have their value, they are just one element of the current innovation story. Taking a broader view, among the most interesting (and lesser-celebrated) areas where advanced tools look set to drive major change is data management.
GenAI has significant potential to impact how data is accessed and evaluated, in a positive way. Far from replacing existing analytics platforms – or for that matter, analysts — smart solutions have the capacity to help ensure tasks across the data lifecycle are performed more efficiently by automating repetitive processes and making it easier for business users to get the insights they need, faster. Or in short, enabling data-driven teams to run even better.
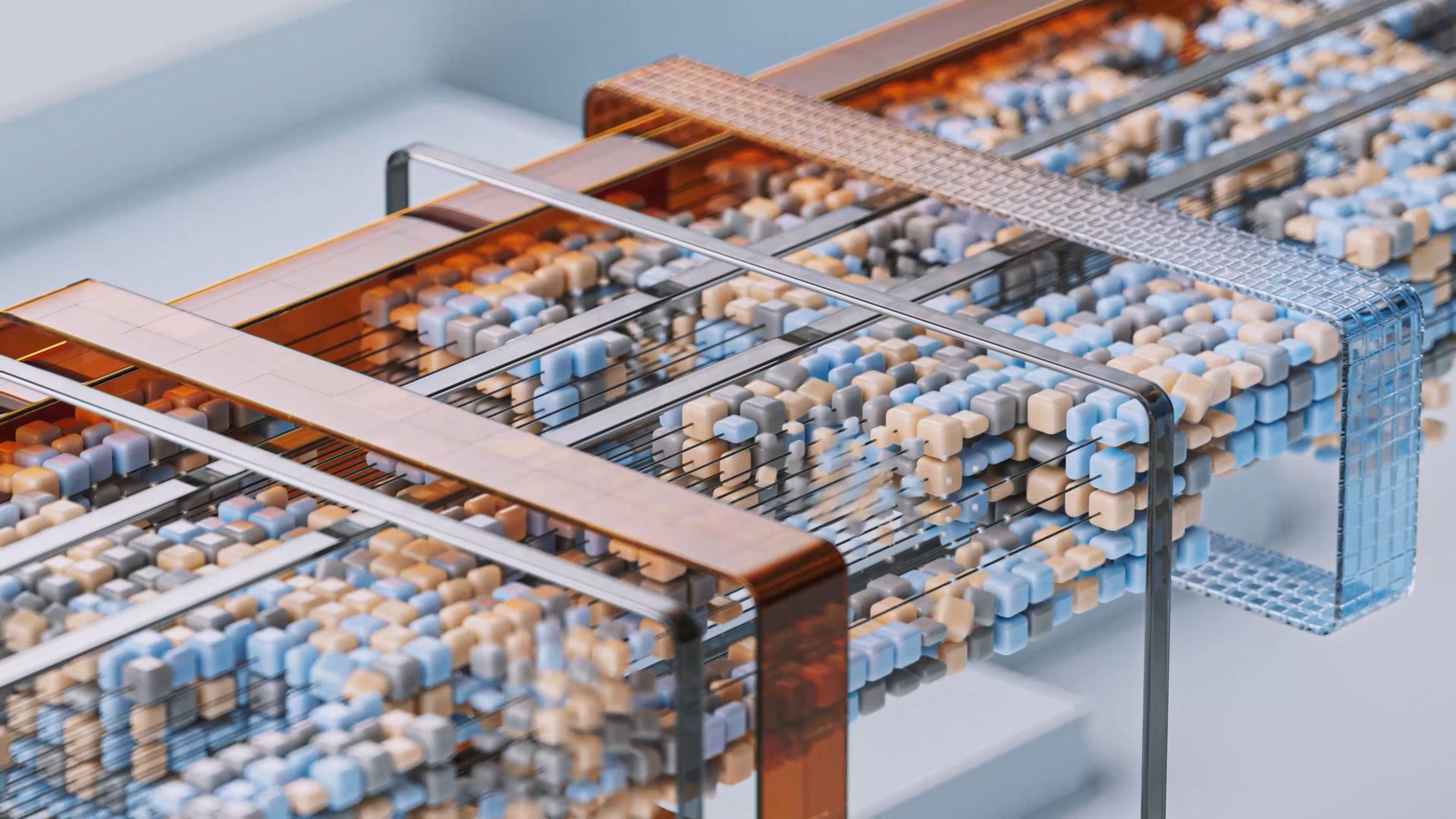
What exactly is GenAI?
Widespread buzz doesn’t guarantee universal understanding. For all the hype about GenAI, a common stumbling block for those aiming to harness its benefits is limited knowledge of key differentiators from other forms of AI.
In short, the main factor that sets this tech apart is what it can do: generating entirely new outputs using existing data, including text, code, images, and video. Grasping why this is an evolution from previous AI requires more explanation.
Traditionally, AI solutions have been trained on vast data sets to perform identification tasks in line with set rules and patterns. Years of deep learning development have now brought us to a point where foundation models are still steered by extensive training data, but their architecture is able to do more than identify objects and information. Thanks to advances in computing capacity, they can process bigger volumes of unstructured data and handle myriad tasks at once, which include creating content and answers on their own.
Deploying self-supervision, they also collect feedback from each interaction and leverage this insight for independent learning: making responses more accurate, valuable, and less robotic over time. Probably the best-known example of that is ChatGPT, which uses a large language model (LLM) to fulfill requests with increasingly nuanced and human-like responses.
One way to illustrate the differences is comparing Amazon’s original Alexa and the new, GenAI-enhanced upgrade. While the Mark One assistant could only react to pre-defined prompts with limited replies, its latest iteration taps a broader range of real-time information to meet open questions with tailored answers. Call it the super-charged version of Ask Jeeves.
How can it bolster data efficiency?
GenAI is a strong ally for many business users, but data experts are the most natural fit. As shown by our Alexa analogy, intelligent tools have been designed to take variable inputs and produce refined outputs — A.K.A the basic premise of analysis. Unsurprisingly, the number one data management use case is making doing so at scale easier and quicker.
Using GenAI to automate top-level data assessment and summarise key findings can bolster efficiency on multiple fronts. As well as eliminating labor-intensive manual wrangling and reducing the risk of human error, this includes enabling data teams to swiftly work through ad hoc requests; creating more time for focusing on the in-depth analysis that matters.
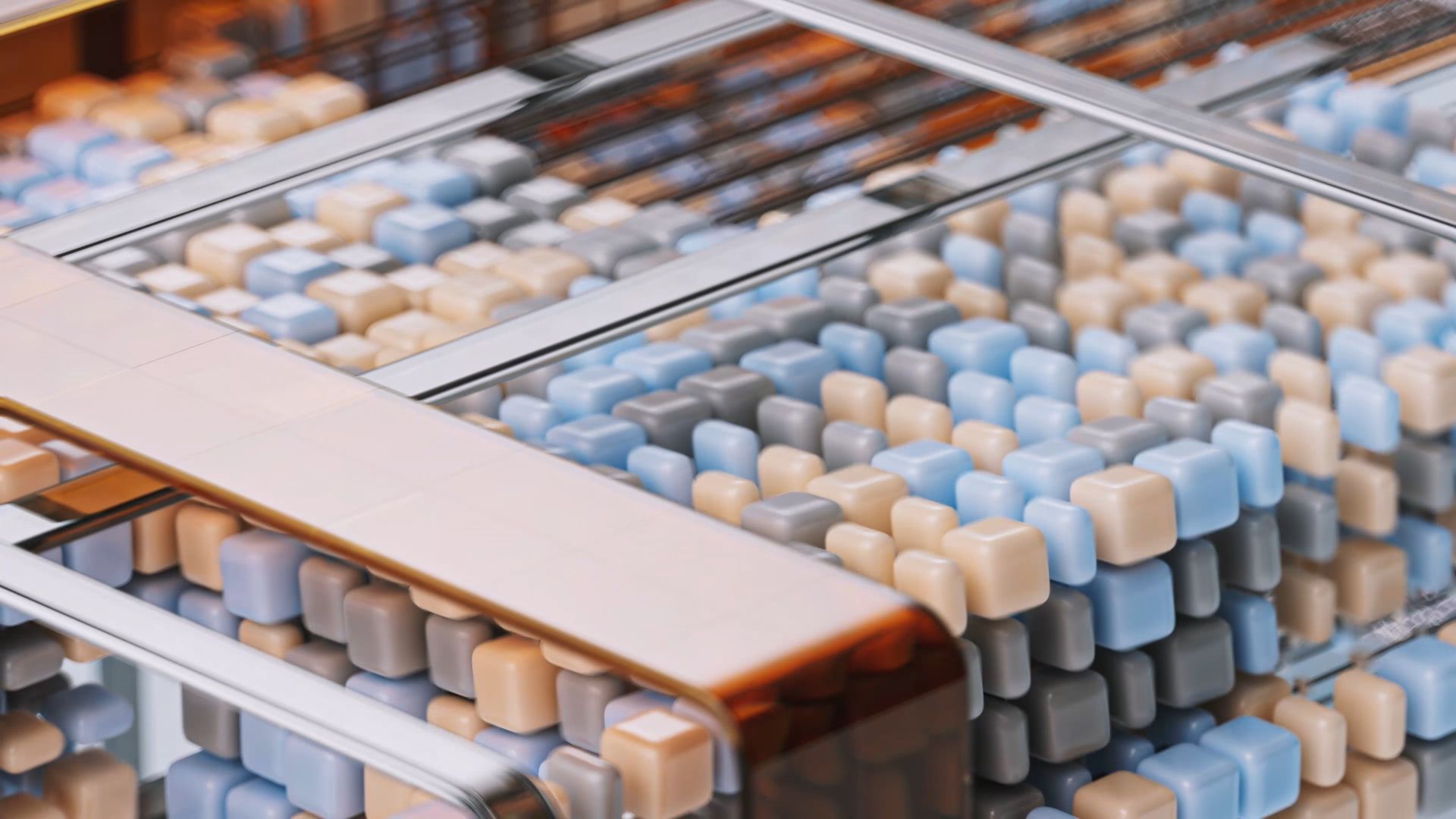
There are, however, additional opportunities for sophisticated tools to cut down complexity and enhance data usability when leveraged alongside existing analytics platforms. Right now, the brightest prospects lie in two core areas:
Self-service analytics
Aside from winning back vital bandwidth for data specialists, innovations in AI-supported evaluation have the potential to significantly bolster company-wide data accessibility and accelerate time to value. The smartest allow non-technical users to enter natural language (i.e., plainly worded) prompts and then take the data coordination wheel: instantly generating SQL queries that retrieve relevant data and presenting it via easily digestible visualizations.
To illustrate the benefits this drives, let’s look at a practical example. Say a marketer wants to compare lead acquisition costs for their latest campaign to the industry benchmark. Behind the scenes, solutions can make pre-cleansed and unified data from their analytics platform available through GenAI systems, such as ChatGPT or Bard, and combine it with data gathered from across the web. On the user side, this smooth integration makes it simple to ask for what they want and receive a clear graph that maps correlations and enables fast decision-making.
Bespoke data transformation
Of course, data needs aren’t restricted to answering singular questions — and nor are GenAI’s capabilities. Transformation assistants not unlike Alexa have begun to emerge that give users the freedom to specify how they want whole data sets to be configured and produce bespoke code for fulfilling their requests. Coupled with step-by-step implementation instructions, the result is a custom transformation kit for getting the right insights, in the right format.
The headline advantages here are flexibility and autonomy. Regardless of their experience level, users can manage and mold data in whichever way they like; determining how to extract useful information and quickly putting it into action. But it’s also worth noting that such tools can play a major part in improving cross-business maturity by empowering users to wield data for themselves, which means yet more time for data experts to spend on high-priority tasks. Or put another way, the realization of the low/no-code dream.
Which safety measures are most essential?
As with any new tech, a successful application will involve mitigating possible hazards. High on the list of obvious challenges is the tendency for GenAI to hallucinate, which will make it crucial to establish rigorous quality monitoring procedures to validate data and rapidly flag potential inaccuracies for deeper investigation. Similarly, addressing well-known security issues will call for robust internal policies and guardrails.
Businesses that already closely scrutinize solutions prior to adoption will be ahead of the risk prevention game, especially if procedures are cross-functional. Looping in users, legal, and security teams can ensure evaluation considers both the value tools provide and whether they offer stringent enough controls for protecting sensitive data. Combined with detailed policies outlining where GenAI features should and shouldn’t be used, such approaches can go a long way toward minimizing the chances of misuse and unforeseen problems.
On top of this, however, it will also be equally important to carefully control the data driving GenAI. Whatever analysis is produced is directly informed by the information feeding it – so, if the data teams provide is incorrect or incomplete, AI output AI will be unreliable and fragmented.
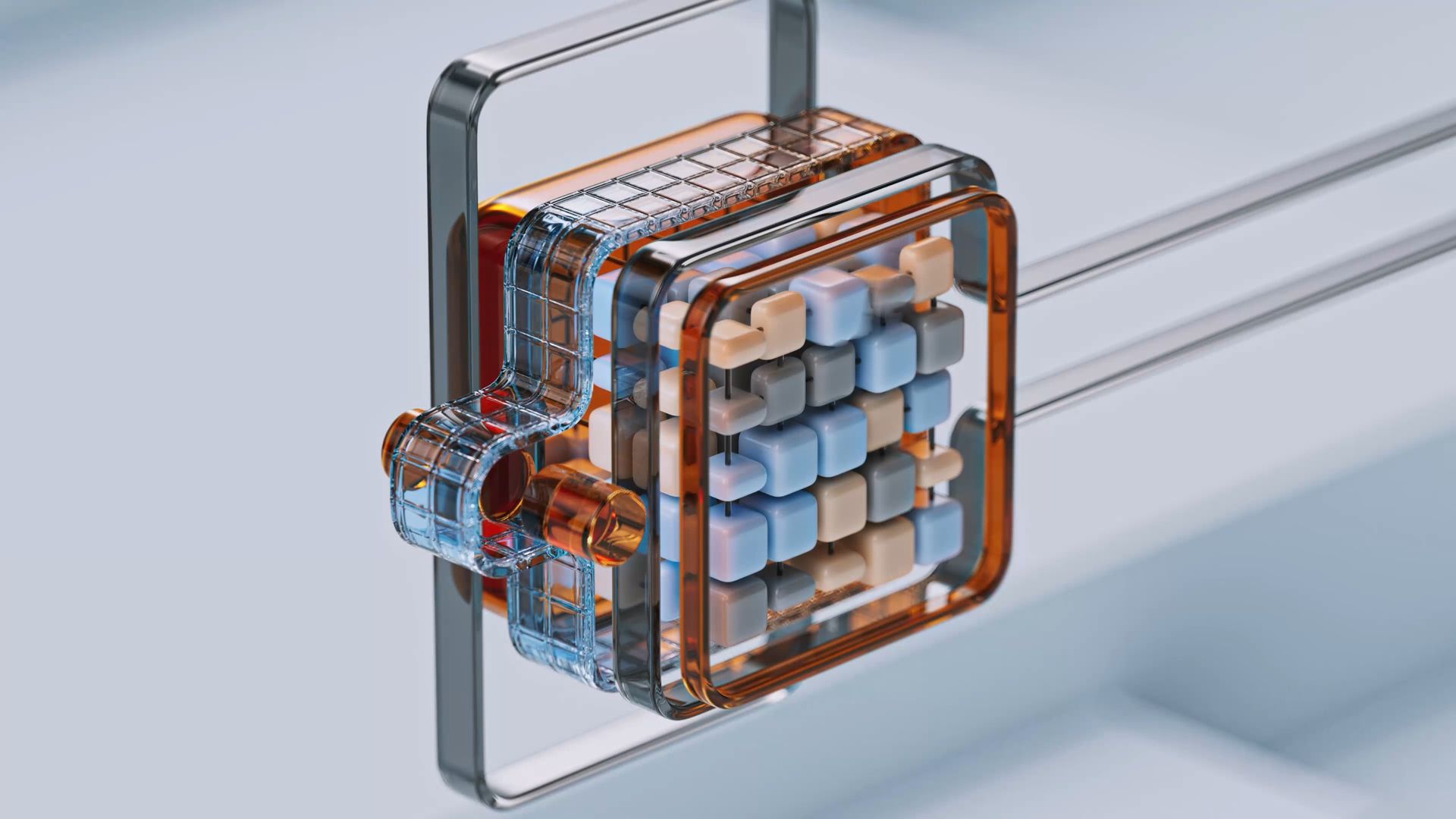
All of which makes solid foundations paramount. Specifically, companies need a streamlined infrastructure of pipelines that can supply clean, consolidated, and comprehensive data, in addition to a culture centered around embracing data-enabled productivity and continually honing data handling skills.
This is just the first stage of a long-term evolution that will allow teams to work smarter. Over the next few years, almost every role (including analyst positions) will have an AI-powered assistant that will liberate human employees from onerous manual tasks and allow them to make better use of their knowledge and abilities; with GenAI evolving from tool to teammate. Moreover, there will also very likely be even more demand for data specialists to not only take charge of running a slicker, AI-assisted show but also dive into the insights intelligent solutions produce and find better ways of using them to optimize productivity.
Featured image credit: Google Deepmind/Unsplash.