In a groundbreaking study conducted by Cambridge scientists, a fascinating parallel has emerged between artificial intelligence (AI) systems and the intricate workings of the human brain.
By imposing physical constraints on artificial intelligence models, reminiscent of the biological constraints shaping human brains, researchers have witnessed the spontaneous development of features akin to those found in the brains of complex organisms.
This revelation opens a new chapter in artificial intelligence research, shedding light on how these systems, when subjected to limitations, can autonomously evolve to solve tasks, mirroring the adaptive capabilities of the human brain.
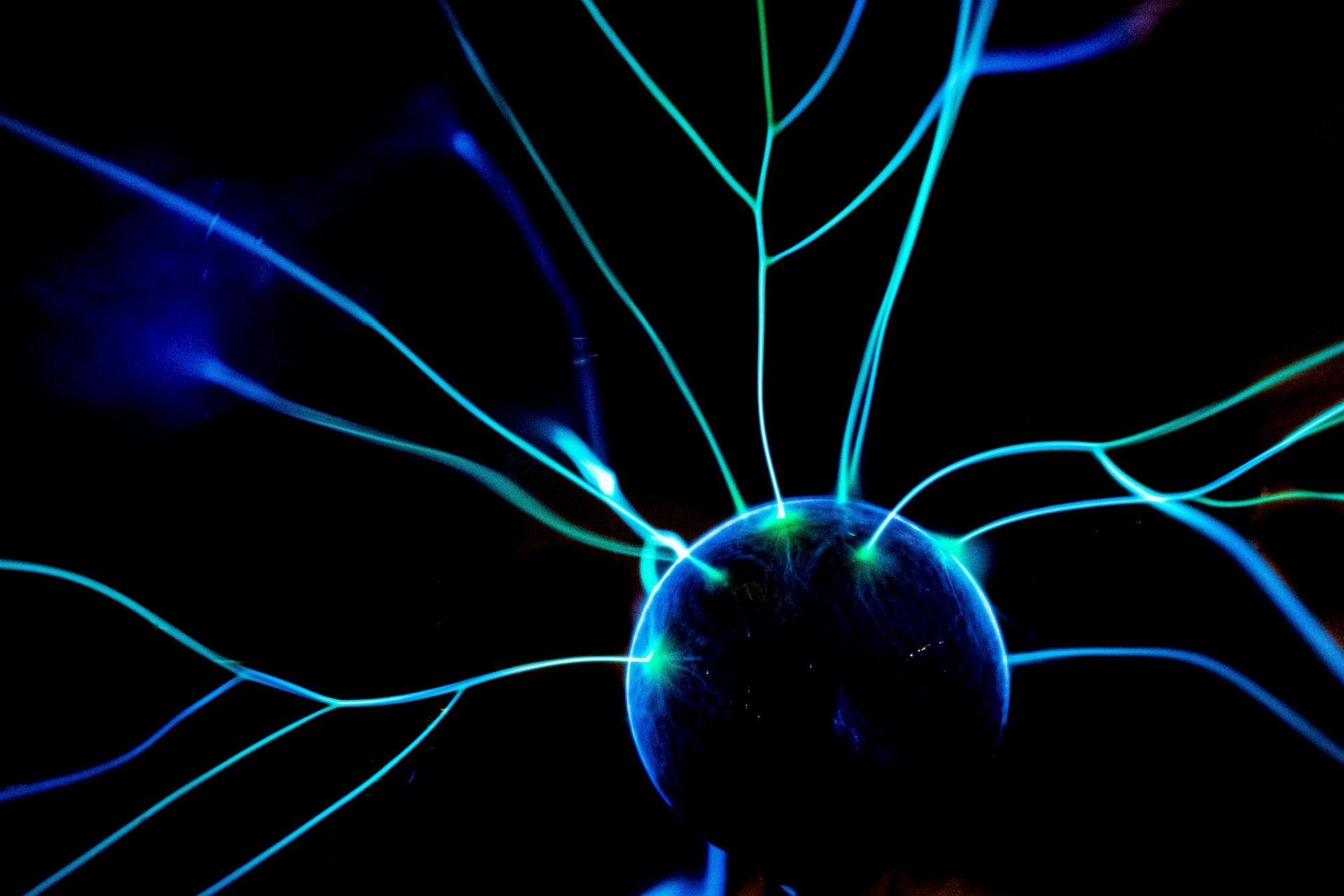
Biological touch to the artificial intelligence
As the study unfolds, it becomes evident that neural systems, including the human brain, must navigate a delicate balance between various demands. Energy and resources are crucial for both sustaining the physical network and optimizing it for efficient information processing.
This delicate trade-off, observed across species, prompts the question of why many biological brains converge on similar organizational solutions. Jascha Achterberg, a Gates Scholar from the Medical Research Council Cognition and Brain Sciences Unit at the University of Cambridge, notes, “Not only is the brain great at solving complex problems, it does so while using very little energy”.
The researchers, led by Achterberg and co-author Dr. Danyal Akarca, designed an artificial system intended to emulate a simplified version of the human brain. In a departure from traditional neural network models, their system introduced a ”physical” constraint, assigning specific locations to computational nodes within a virtual space.
The farther apart the two nodes were, the more challenging it became for them to communicate – a reflection of the spatial organization seen in biological brains.
The study’s focus on the physical distance between nodes in the artificial system offers valuable insights for designing bio-inspired AI architectures. The notion that the system developed hubs – highly connected nodes – aligns with the spatial organization seen in biological brains.
This concept holds substantial promise for the development of more efficient AI systems, especially in real-world scenarios where physical constraints play a significant role. The potential applications extend beyond traditional computing tasks, envisioning AI systems that navigate physical spaces with energy-efficient structures inspired by the human brain.
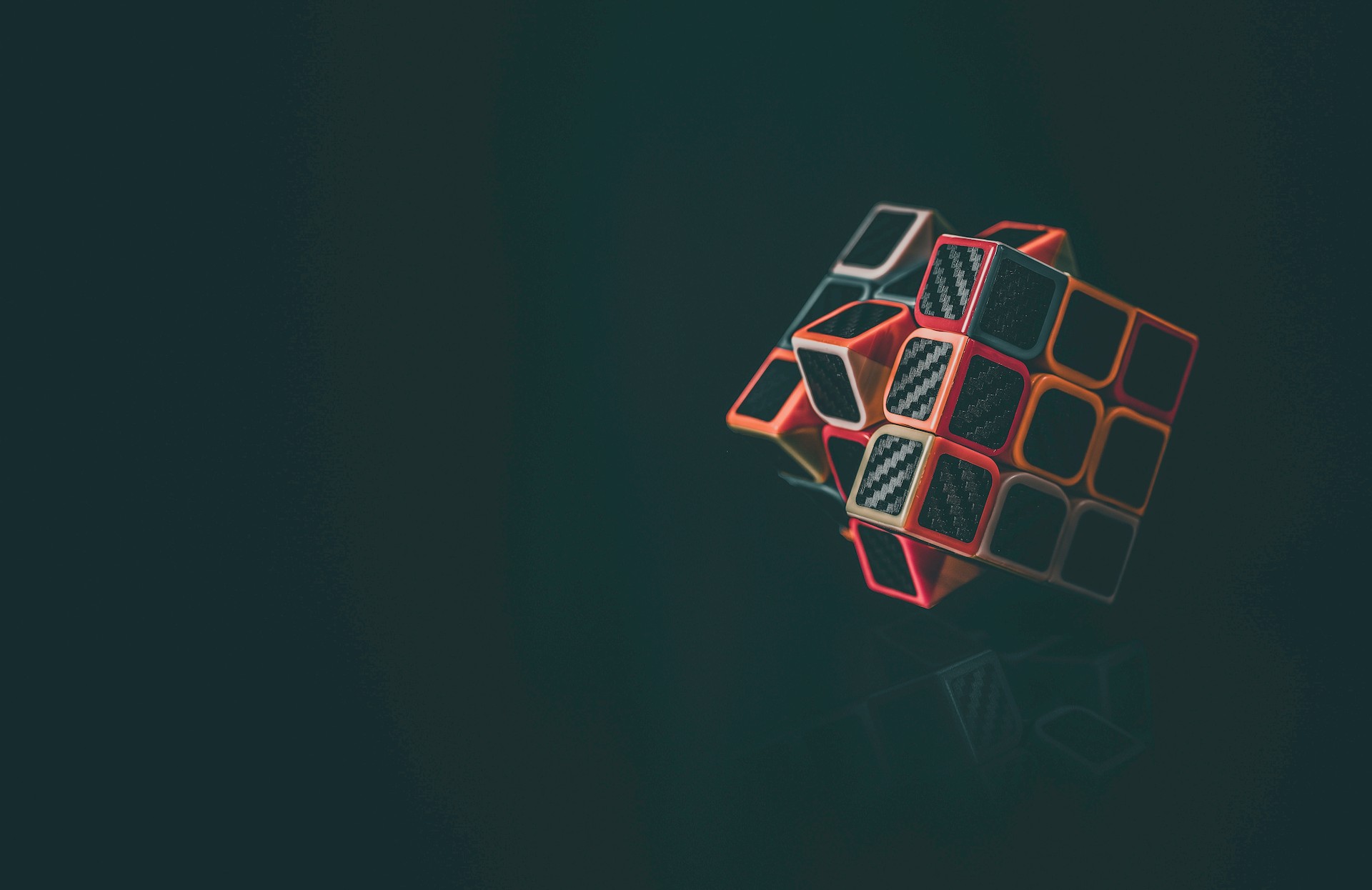
Remarkable advancement
To put their bio-inspired artificial intelligence system to the test, the researchers assigned it a maze navigation task, a simplified version of challenges given to animals in behavioral neuroscience. The AI system, armed with computational nodes instead of real neurons, initially grappled with the task but learned and improved over time through feedback.
The physical constraint introduced a layer of complexity, forcing the system to develop hubs – highly connected nodes that mimicked the information conduits observed in human brains.
Remarkably, the researchers observed a shift in the response profiles of individual nodes. Instead of rigidly coding for specific properties of the maze, nodes in the AI system developed a flexible coding scheme. This flexibility, where nodes could encode multiple aspects of the task at different moments, mirrors a characteristic observed in the brains of complex organisms.
We still have so much to learn from nature
Delving deeper into the study’s findings, the physical constraint imposed on the artificial intelligence system prompted the development of solutions strikingly similar to those evolved by biological systems. In particular, the limitations on wiring nodes that are physically distant compelled the artificial system to produce intricate characteristics reminiscent of the human brain.
This suggests a fundamental connection between the constrained environment and the emergence of elegant solutions, shedding light on the evolutionary forces that shape both artificial intelligence and biological neural architectures.
Fasten your seatbelts
A significant implication of the study lies in its relevance to AI systems operating in dynamic environments. The concept that robots mimicking the brain’s structure could efficiently process changing information while managing limited energy supplies addresses a critical challenge in robotics.
This insight could pave the way for the development of robots capable of real-time adaptation, processing sensory inputs, and navigating through physical spaces with efficiency comparable to the adaptive nature of the human brain.
As we witness the evolution of AI systems mirroring biological features, ethical considerations come to the forefront. The study prompts a reflection on the autonomy granted to evolving AI structures and underscores the importance of responsible innovation.
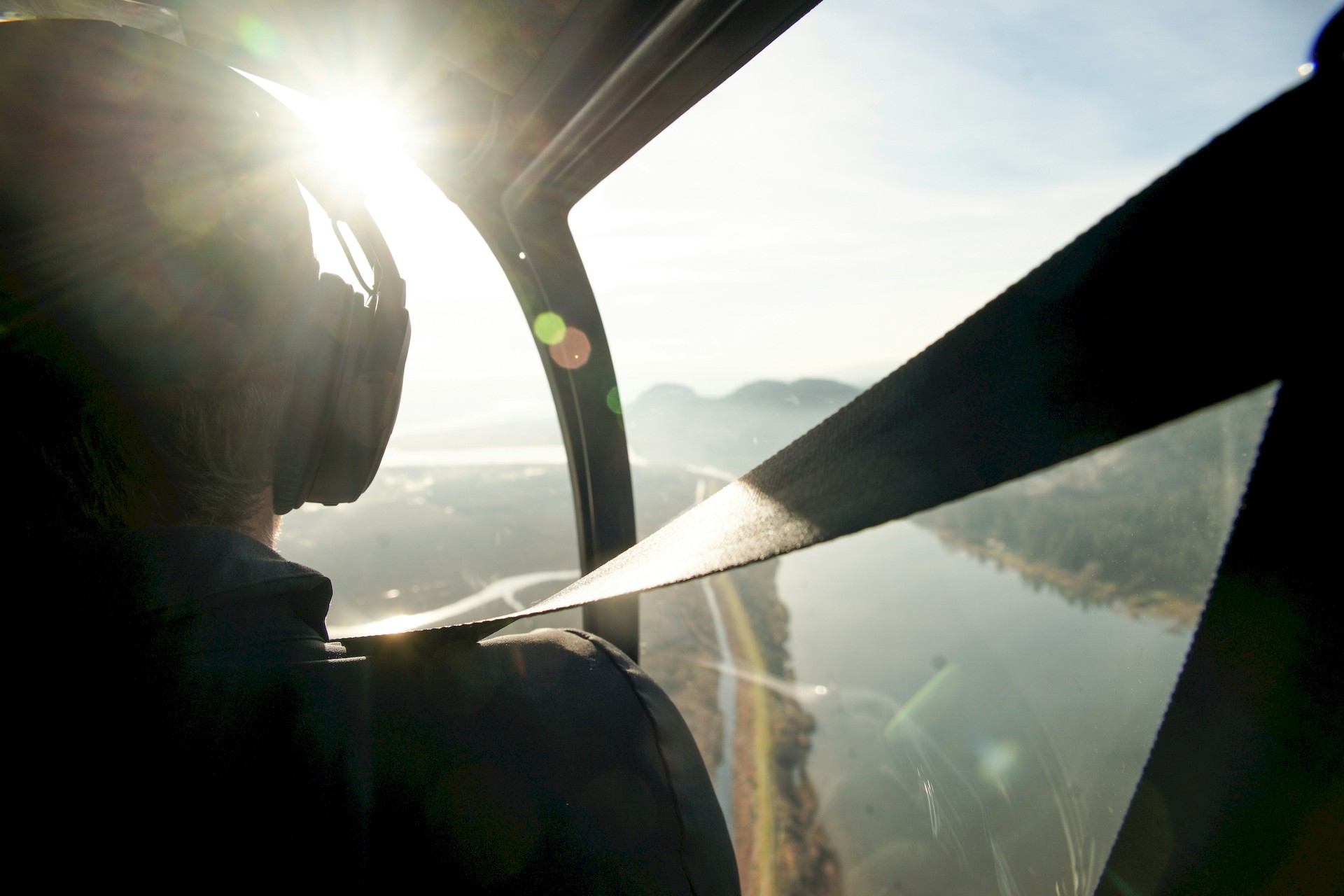
Balancing technological advancements with ethical frameworks becomes imperative to ensure that AI technologies align with societal values. The ethical implications extend beyond the laboratory, resonating in the broader discourse on the responsible development and deployment of artificial intelligence.
The study not only unveils the self-organizing capabilities of AI systems but also offers a spectrum of implications for diverse fields. From enhancing our understanding of neural coding to inspiring bio-mimetic AI architectures and addressing real-world challenges in robotics, the study propels us into a future where the synergy between technology and biology reshapes the landscape of artificial intelligence.
Featured image credit: Milad Fakurian/Unsplash.