Google DeepMind’s latest innovation, GraphCast weather forecast AI, marks a significant advancement in weather prediction technology. The impact of weather is ubiquitous, influencing everything from daily wardrobe choices to energy production, and in extreme cases, creating storms that have profound effects on communities. As global weather patterns become increasingly volatile, the demand for swift and reliable weather forecasts has escalated.
A recent publication in Science introduces Google DeepMind’s GraphCast, an AI model that sets new standards in medium-range weather forecasting. GraphCast excels in predicting weather conditions up to 10 days in advance, surpassing the accuracy and speed of the established industry standard—the High Resolution Forecast (HRES), developed by the European Centre for Medium-Range Weather Forecasts (ECMWF).
Beyond its remarkable forecasting precision, GraphCast is adept at providing earlier warnings for severe weather events. It boasts of advanced capabilities in predicting cyclone paths, identifying atmospheric rivers that indicate potential flooding, and forecasting extreme temperature events, all of which are crucial for effective disaster preparedness and potentially life-saving interventions.
GraphCast represents a significant stride in applying AI to weather prediction, delivering forecasts that are not only more accurate but also more efficient. This breakthrough is pivotal for informed decision-making across various industries and societies. In a move to democratize AI-powered weather forecasting, Google DeepMind has open-sourced the GraphCast model code, enabling scientists and forecasters worldwide to enhance daily life for billions. Notably, weather agencies like ECMWF are already utilizing GraphCast, conducting live experiments with the model’s forecasts on their platform.
Google DeepMind’s GraphCast tackles the complexity of medium-range weather prediction
Weather forecasting stands as one of humanity’s most enduring and intricate scientific challenges. The ability to make medium-range predictions accurately is crucial for a myriad of sectors, from renewable energy generation to planning large-scale events. However, achieving accuracy and efficiency in these forecasts has always been a formidable task.
Traditionally, weather forecasts have relied on Numerical Weather Prediction (NWP). This method starts with meticulously crafted physics equations, subsequently converted into algorithms for supercomputer processing. While this approach has been a monumental achievement in science and engineering, crafting these equations and algorithms demands extensive expertise, time, and substantial computing resources to yield precise predictions.
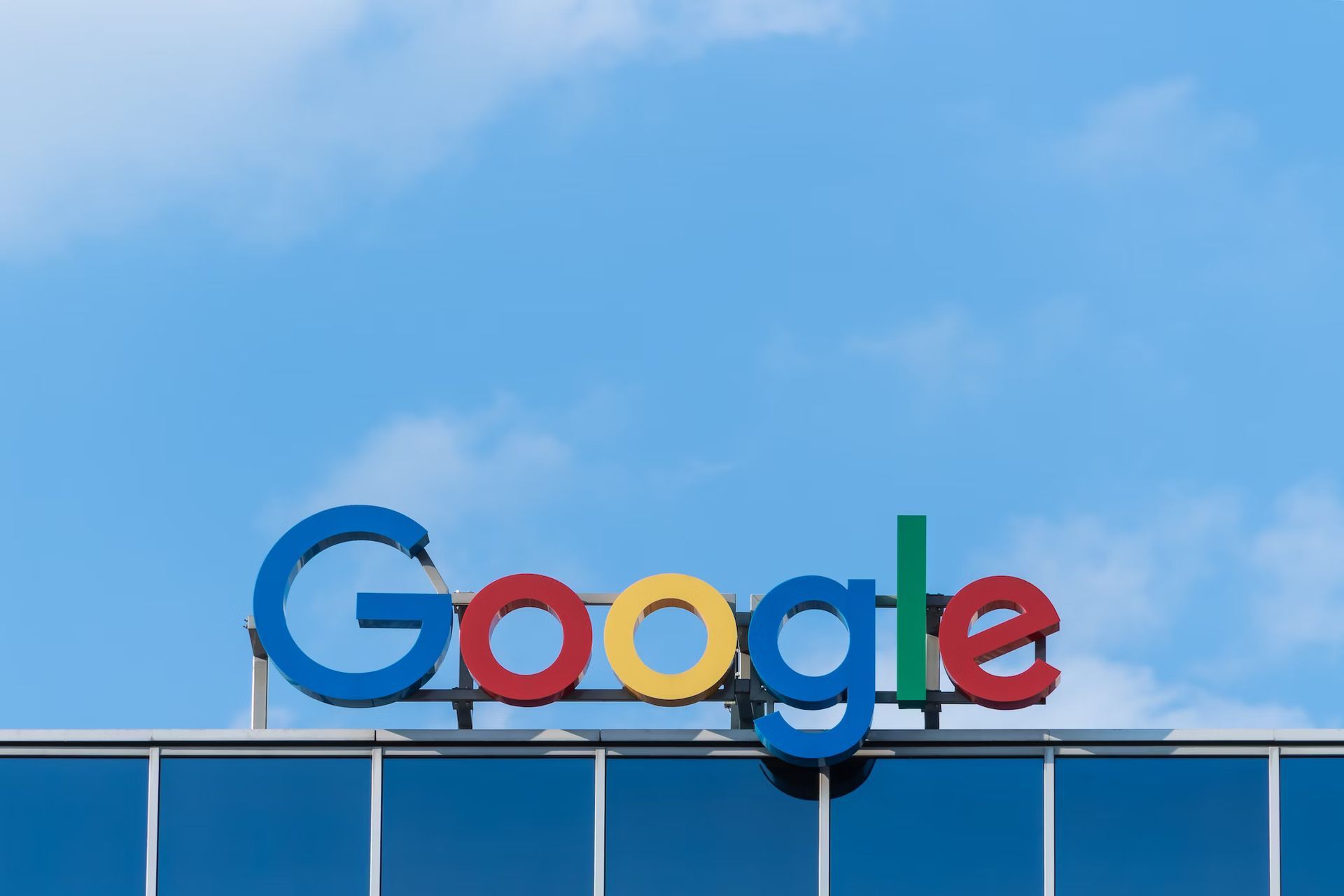
Deep learning tech is presenting an alternate route: leveraging data over physical equations to construct a weather forecasting system. Google DeepMind’s GraphCast weather forecast AI harnesses decades of historical weather data, learning the complex causal relationships that dictate the evolution of Earth’s weather. This method provides insights into weather patterns from the present extending into the future.
Notably, GraphCast doesn’t operate in isolation but works in synergy with traditional methods. GraphCast was trained using four decades of weather reanalysis data from ECMWF’s ERA5 dataset. This extensive collection, comprising historical weather observations like satellite imagery, radar, and readings from weather stations, utilizes traditional NWP models to create comprehensive records of global historical weather, filling in gaps where direct observations might be lacking.
How does GraphCast Weather forecast AI work?
The integration of machine learning and Graph Neural Networks (GNNs) in Google DeepMind’s GraphCast weather forecast AI marks a transformative approach in meteorological prediction. This innovative system specializes in processing spatially structured data, an essential factor for accurate weather modeling.
GraphCast weather forecast AI operates at an extraordinary resolution of 0.25 degrees longitude/latitude, translating to a detailed 28km x 28km grid at the equator. This high level of precision covers over a million grid points across the Earth’s surface. At these points, Google DeepMind’s model comprehensively predicts critical Earth-surface variables, including temperature and wind dynamics, alongside six atmospheric factors across 37 altitude levels, such as humidity and temperature variations.
Despite the intense computational demands during its training phase, the GraphCast weather forecast AI emerges as a highly efficient forecasting tool. The AI can complete 10-day weather forecasts in less than a minute when run on a single Google TPU v4 machine. This efficiency is a significant improvement over traditional methods like HRES, which require several hours and a vast array of supercomputers.
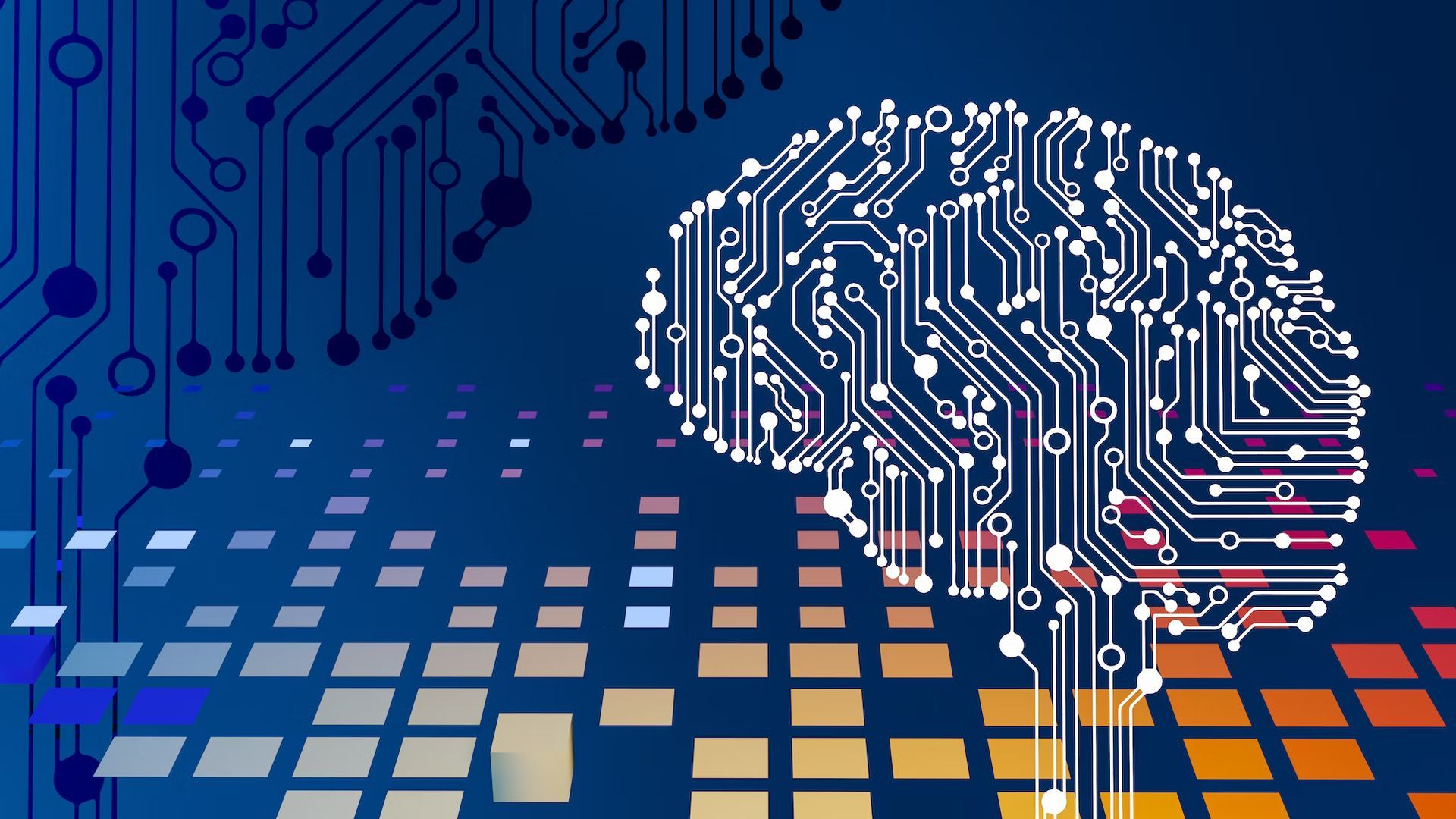
In a rigorous performance test against the established HRES system, Google DeepMind’s GraphCast weather forecast AI demonstrated superior accuracy in over 90% of 1380 test variables and forecasting periods. The model’s performance is even more striking within the troposphere, the crucial atmospheric layer closest to Earth. Here, GraphCast outperformed HRES on 99.7% of test variables, showcasing its exceptional capability in predicting future weather conditions.
GraphCast Weather forecast AI requires just two data sets to function: the weather state from six hours prior and the current weather conditions. With this information, it accurately forecasts the upcoming six-hour weather scenario. This process can be sequentially extended in 6-hour increments, enabling Google DeepMind’s model to provide state-of-the-art forecasts up to a remarkable 10 days in advance.
Early detection of severe weather with GraphCast
Google DeepMind’s GraphCast weather forecast AI has demonstrated an exceptional ability to identify severe weather events earlier than conventional models, a feature not explicitly trained for. This capability exemplifies how GraphCast could significantly enhance preparedness, potentially saving lives and mitigating the impact of storms and extreme weather on communities.
By integrating a simple cyclone tracker into GraphCast forecasts, the model achieves superior accuracy in predicting cyclone movements compared to the HRES model. Notably, in a live demonstration on the ECMWF website, GraphCast accurately forecasted Hurricane Lee’s landfall in Nova Scotia nine days in advance, a prediction more precise and earlier than those made by traditional forecasting models.
DeepMind Sparrow is a new AGI that is safer and more precise
In the context of a warming world, predicting extreme temperatures is increasingly critical. Google DeepMind’s GraphCast excels in identifying potential heatwaves, anticipating when temperatures are likely to exceed historical highs for any given location. This predictive capability is vital for preparing for heatwaves, disruptive and dangerous events that are occurring with greater frequency.
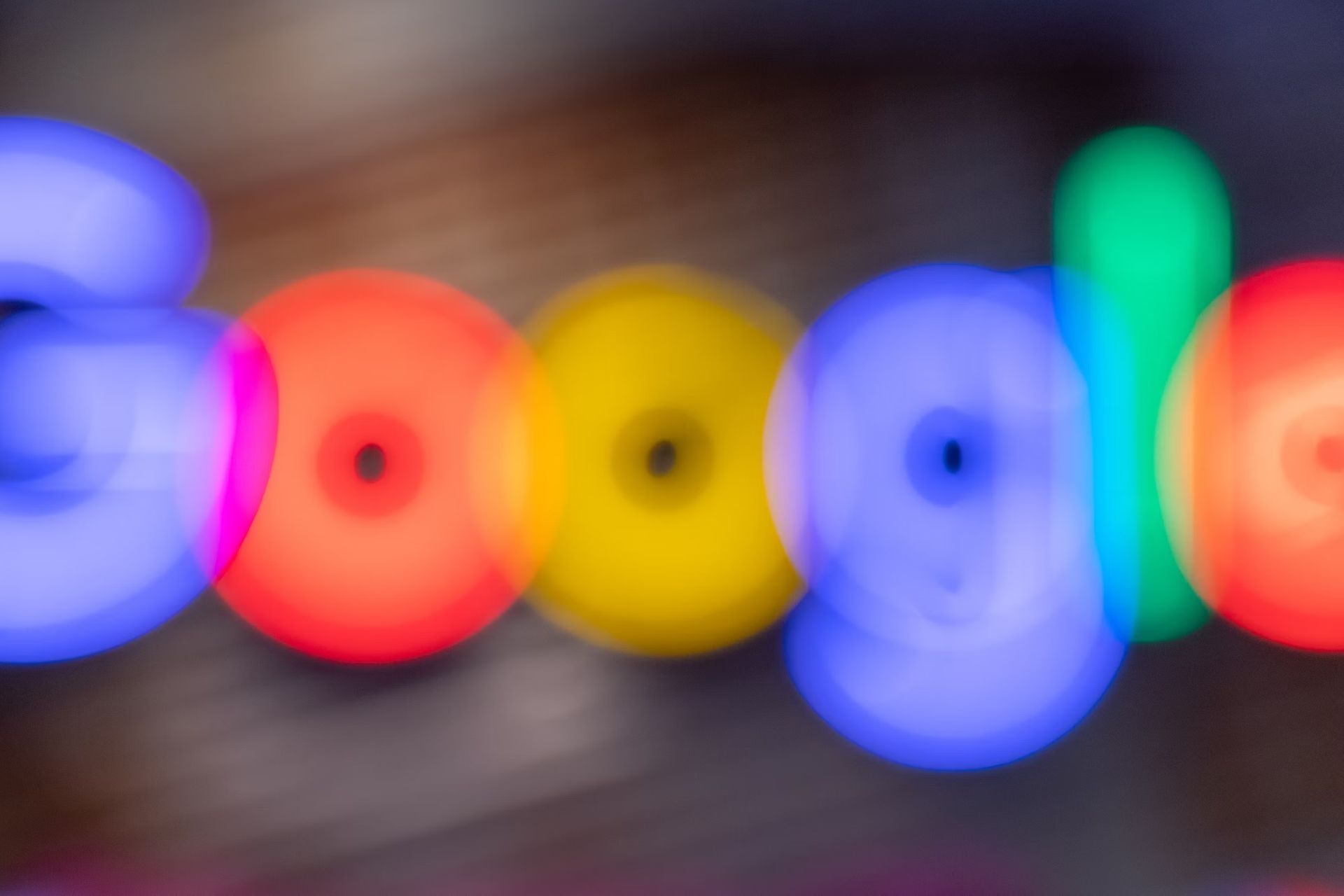
AI-powered weather forecasting
Google DeepMind’s GraphCast weather forecast AI now claims to be the world’s most precise system for 10-day global weather forecasting, offering unprecedented capabilities in predicting extreme weather events well into the future. As climate change continues to reshape weather patterns, GraphCast is poised to adapt and enhance its performance with the integration of increasingly high-quality data.
Featured image credit: Wolfgang Hasselmann/Unsplash