NVIDIA SteerLM is ushering in a transformative era for language models, bridging the gap between unparalleled linguistic capabilities and user-centric customization. As the latest innovation from NVIDIA, SteerLM promises not only to redefine the boundaries of natural language processing tasks but also to empower developers and users alike with enhanced control and adaptability.
Traditionally, to elevate these models to their fullest potential, there’s been a blend of supervised fine-tuning (SFT) and reinforcement learning from human feedback (RLHF). Imagine it as meticulously calibrating a telescope to see distant galaxies in sharper focus. However, while RLHF can fine-tune the focus, it’s not without its challenges — like training intricacies and less-than-optimal user control.
But here’s where the narrative takes an exciting turn. The NVIDIA Research Team, always at the forefront of innovation, has introduced NVIDIA SteerLM. More than just a shiny new tool in the toolbox, SteerLM serves as a beacon, guiding LLMs to generate content more closely aligned with what you envision. Nestled within the NVIDIA NeMo framework, SteerLM is all about user empowerment, allowing dynamic customization of model outputs based on specified attributes.
What is NVIDIA SteerLM?
When it comes to the world of language models, control is the name of the game. Imagine being a conductor, directing an orchestra to adjust the tempo or emphasize certain instruments. This is where NVIDIA SteerLM takes center stage, granting you the baton to orchestrate responses during inference.
- NVIDIA SteerLM trains an attribute prediction model using human-annotated datasets. Think of this as laying the groundwork, understanding the nuances of attributes like helpfulness, humor, and creativity. It’s like tuning the instruments before a grand symphony.
- Using the predictive prowess from Movement 1, SteerLM annotates diverse datasets. It’s like adding more sheet music to our orchestra, broadening the range and variety of compositions.
- Here’s where the magic happens. The LLM undergoes attribute-conditioned SFT, generating responses based on specific attribute combinations. Imagine instructing the violin section to evoke feelings of nostalgia while the cellos imbue a sense of wonder.
- Bootstrapping involves generating the most harmonious responses and then refining them. Picturing a crescendo in music, SteerLM focuses on achieving the pinnacle of quality and then fine-tuning for even greater resonance.
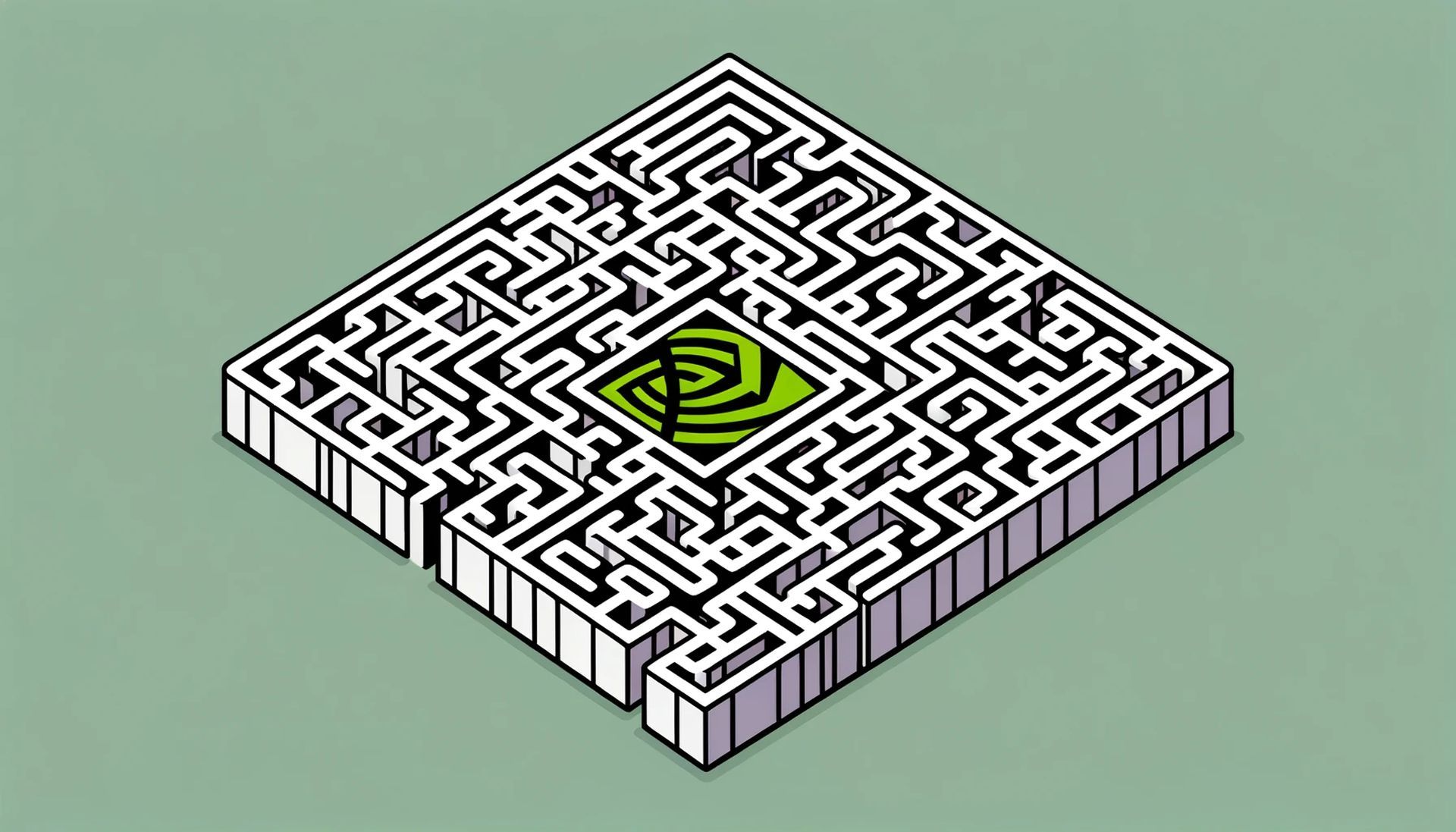
One of the standout features of SteerLM is its simplicity. By focusing on the core language modeling objective, it elegantly bypasses the complications of RLHF. And the encore? NVIDIA SteerLM supports a user-steerable AI. This means, as the developer, you’re in the director’s chair, adjusting attributes in real-time, ensuring the final act (or application) is tailored precisely to your vision, free from the constraints of pre-set preferences.
Promise and pitfalls of LLMs
Language models today stand at a fascinating crossroads of immense promise intertwined with potential challenges. By tapping into vast text reservoirs, LLMs don impressive linguistic caps and a treasure of worldly knowledge. While researchers have put these models to the test in a spectrum of NLP tasks – be it translation, answering questions, or spinning tales – there’s an Achilles’ heel. These models, every so often, sway away from our directives, opting for outputs that could be generic, repetitive, or even bewildering. In this dance of digital linguistics, the human touch – our feedback – remains an indispensable partner.
Exploring current avenues
Venturing further, supervised fine-tuning (SFT) does enhance the prowess of these models, but at times, it’s like asking a poet to pen a haiku; the responses can become brief and a tad robotic. On the other hand, RLHF tries to optimize by championing the human touch, prioritizing our preferred responses. Yet, it’s not all rosy; RLHF’s intricate training backdrop can sometimes feel like setting up a grand opera for just one note, making it a challenge for widespread embrace.
The era of user-directed AI
SteerLM heralds a new dawn, an era where the user takes the reins. Its brilliance lies in letting you, the user, dictate the flavor of the output. Want a touch of humor or a strict no-toxicity zone? Specify these attributes at the moment of inquiry. It’s akin to being a chef and adjusting the seasoning of a dish right before it’s served. With NVIDIA SteerLM, you’re not just limited to one tailored experience; it opens up a universe of customization, ready to cater to diverse needs in real-time.
The genius of SteerLM is in its adaptability. Let’s explore the myriad of applications it gracefully powers:
- Gaming: Ever wanted your in-game non-player characters to surprise you with their dialogue? SteerLM breathes life into them. Dive deeper into how NVIDIA ACE infuses emotion into NPCs using NeMo SteerLM.
- Education: Picture an AI that perfectly captures a formal, helpful persona, ready to assist students with their burning queries.
- Enterprise: In the corporate world, one size doesn’t fit all. SteerLM understands this, catering to diverse teams with pinpoint precision.
- Accessibility: With SteerLM, it’s possible to rein in undesirable biases, ensuring the output respects sensitive attributes.
Such flexibility promises to unlock a new generation of bespoke AI systems tailored to individual needs.
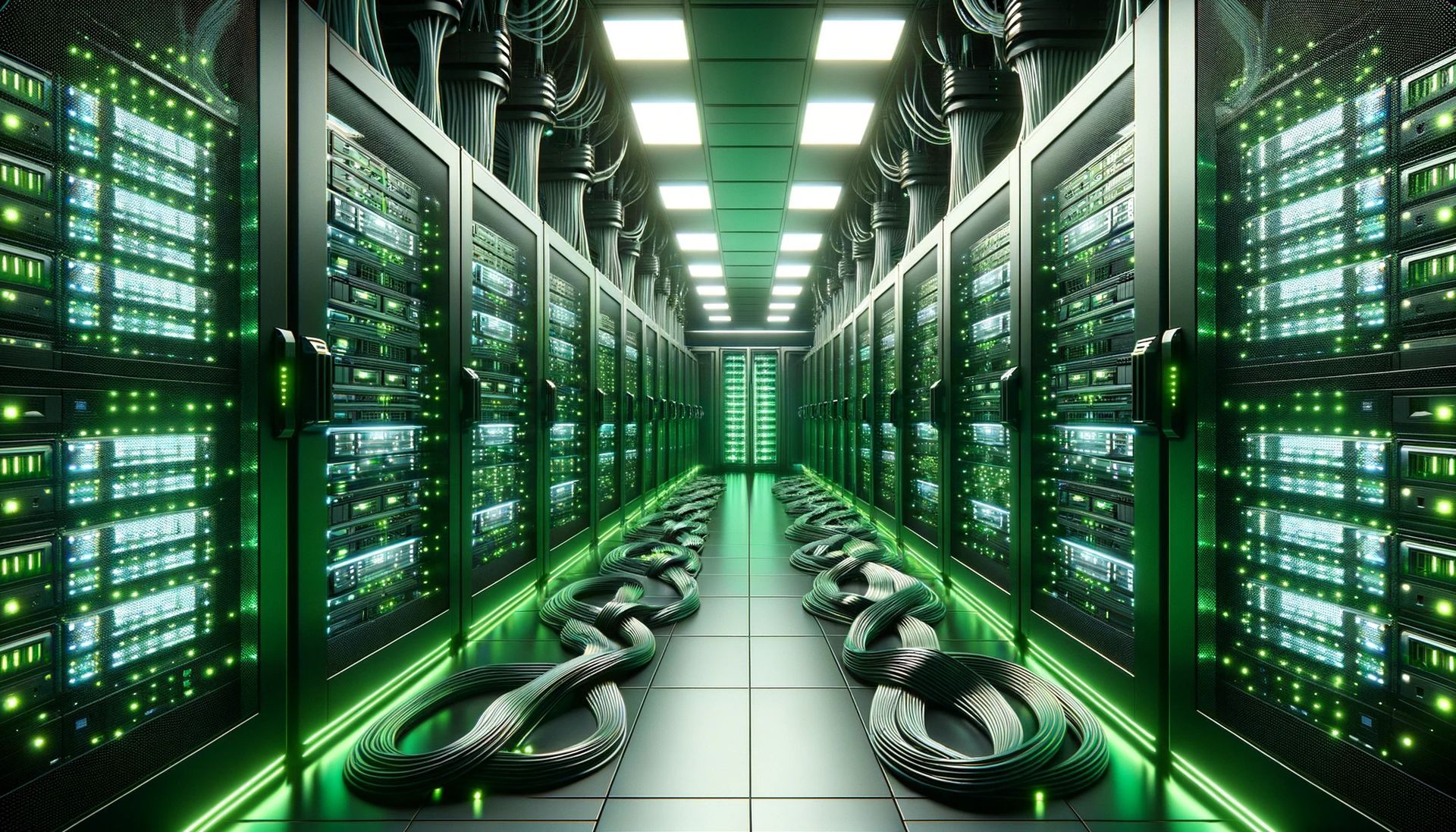
Simplifying mastery in customization
While some advanced customization techniques can feel like navigating a labyrinth, NVIDIA SteerLM is like a refreshing open courtyard. Instead of relying on specialized infrastructures, SteerLM embraces simplicity, making top-tier customization an inviting journey for developers.
And here’s the real kicker: traditional, heavy-duty techniques like reinforcement learning aren’t a necessity for impeccable instruction tuning. By leveraging time-tested methods such as SFT, the journey is simplified, demanding fewer tweaks and turns.
Results speak for themselves. NVIDIA SteerLM’s straightforward approach has yielded some spectacular outcomes. For the stats enthusiasts: In the Vicuna benchmark face-off, SteerLM 43B showcased its prowess, leaving even some RLHF models in its wake. SteerLM 43B notched an impressive average score of 655.75, outshining scores like 646.25 of Guanaco 65B and 612.75 of LLaMA 30B RLHF.
Meet Mistral 7B, Mistral’s first LLM that beats Llama 2
The takeaway? With NVIDIA SteerLM, achieving peak accuracy doesn’t demand a convoluted process. It’s about enabling more hands on deck, democratizing customization for a wider circle of developers.
For those seeking a deeper understanding, NVIDIA’s detailed paper, “SteerLM: Attribute Conditioned SFT as an (User-Steerable) Alternative to RLHF,” provides comprehensive insights. The research sheds light on the nuanced performance of the Llama 2 13B model when fine-tuned with the SteerLM technique.
For guidance on training a SteerLM model, NVIDIA’s official blog offers a step-by-step tutorial and valuable resources.
Featured image credit: Kerem Gülen/DALL-E 3