If you are interested in technology at all, it is hard not to be fascinated by AI technologies. Artificial intelligence, one of the most talked about topics in today’s technology world, has played a huge role in bringing many things into our lives, especially in the last five years. Whether it’s pushing the limits of creativity with its generative abilities or knowing our needs better than us with its advanced analysis capabilities, many sectors have already taken a slice of the huge AI pie. But does that mean artificial intelligence is perfect?
Even though AI has managed to get all the attention for what it can do, we sometimes forget to look at the other side of the scale. While we as humans have difficulty in interpreting life and emotions, AI technologies that we feed with the data we provide are not very successful in this regard either. Interpreting the unpredictable movements of living beings, which make most of their decisions based on hormonal impulses, and hoping that a machine that never experiences the effects of these hormones can do it is unfortunately a big dilemma, taking into account today’s technology.
We’ve already talked about the challenges of artificial intelligence, now let’s talk about the challenges we face in accepting and using artificial intelligence in the most important aspects of our lives.
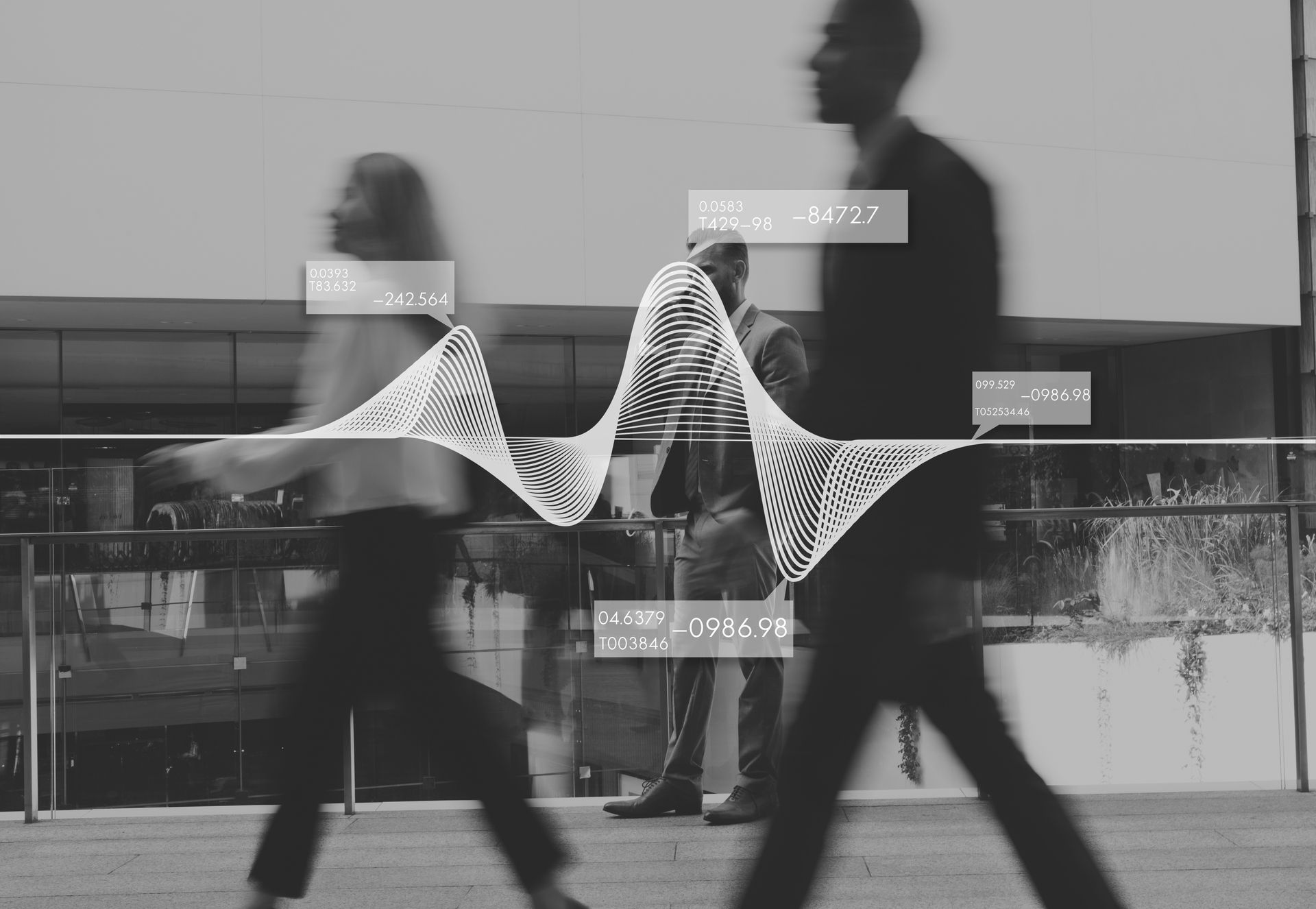
How do AI technologies learn from the data we provide?
AI technologies learn from the data we provide through a structured process known as training. This process is fundamental to machine learning, a subset of AI, and involves several distinct steps.
Firstly, data collection is essential. AI systems require a substantial and diverse dataset that is relevant to the specific problem they aim to solve. This dataset comprises input data (features) and corresponding output or labels, which represent the desired predictions or classifications. For example, in image recognition, the dataset would consist of images and their associated labels, such as identifying whether an image contains a cat or a dog.
Once the data is collected, it undergoes preprocessing. This step ensures that the data is in a suitable format for training. Data preprocessing tasks can include data cleaning to remove errors or inconsistencies, normalization to bring data within a consistent range, and feature engineering to extract meaningful information from raw data.
The next critical step is model selection. AI practitioners choose an appropriate machine learning model or algorithm that aligns with the problem at hand. Common choices include neural networks (used in deep learning), decision trees, support vector machines, and more.
With the model selected, the initialization of parameters takes place. These parameters are the model’s coefficients or weights that dictate its behavior. They are initialized with random values.
The training loop is where the model truly learns. It consists of several iterative steps:
- In the forward pass, the model takes input data and generates predictions based on its current parameters
- A loss function quantifies the disparity between these predictions and the actual labels. The objective is to minimize this loss
- Backpropagation is employed to adjust the model’s parameters using an optimization algorithm like gradient descent. This step ensures that the model continuously refines its predictions
This iterative training process is repeated for multiple epochs, allowing the model to fine-tune its parameters further.
Validation and testing are crucial stages. Validation assesses how well the model generalizes to new, unseen data, while testing evaluates its performance and generalization capabilities more rigorously.
On paper, once the model demonstrates satisfactory performance, it can be deployed in real-world applications to make predictions or automate tasks based on new, previously unseen data.
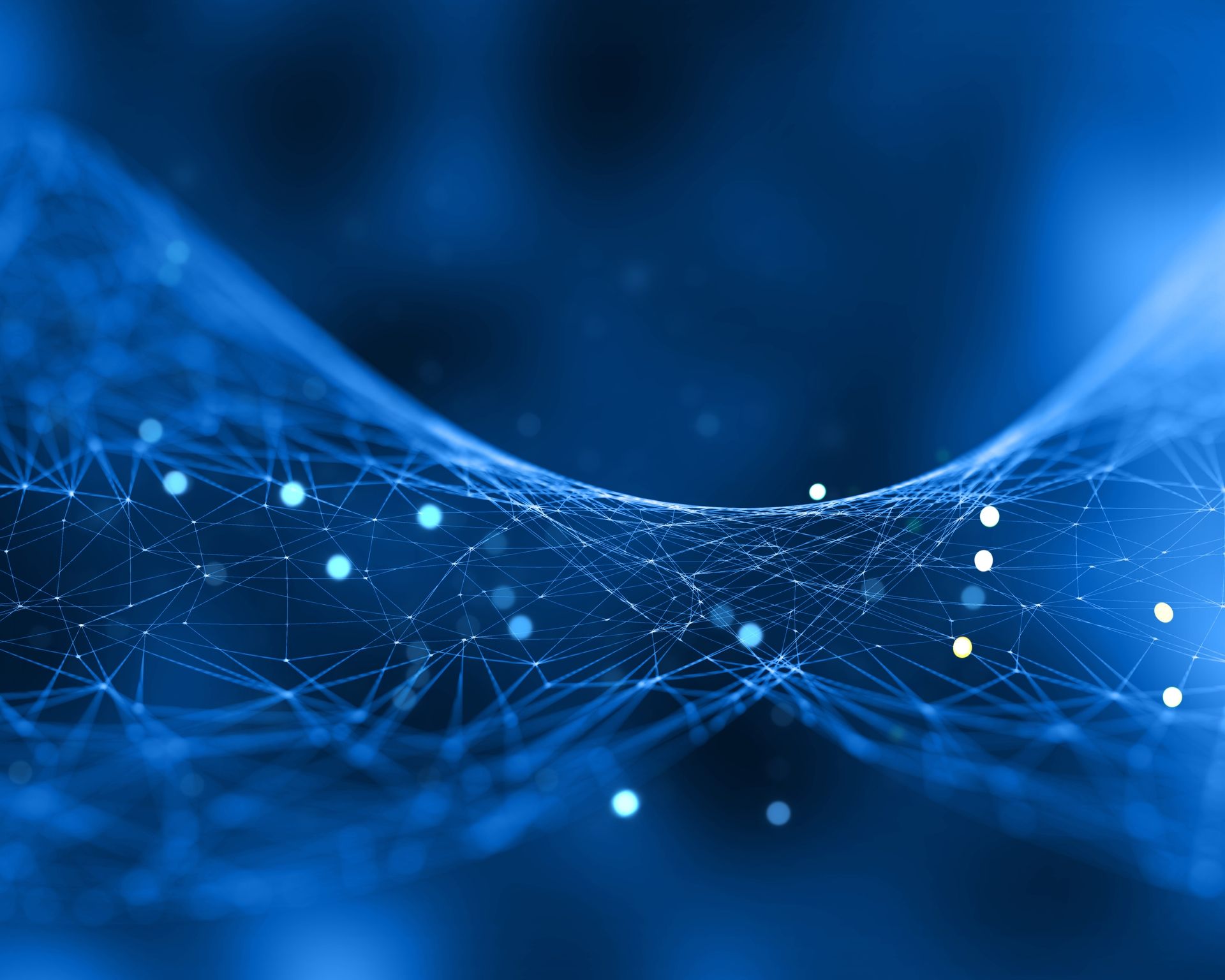
There are several ways that AI technologies can learn from data but the most common approach is supervised learning, where the AI algorithm is trained on labeled data, meaning that the correct output is already known. The algorithm learns to map inputs to outputs by making predictions and comparing them to the true labels. Over time, the algorithm improves its accuracy and can make better predictions on new, unseen data.
So, while data labeling is crucial in supervised learning, can we ever be completely certain of its accuracy? The answer is no, because let’s face it – humans aren’t perfect. We’ve all had moments where we questioned our own abilities, like doubting a medical diagnosis or wondering if a criminal case outcome was truly just. And yet, we’re expected to trust ourselves and the data we label without hesitation. It’s tough to swallow, but the reality is that even with the best intentions, we’re all prone to making mistakes.
Another form of machine learning algorithm is known as unsupervised learning. This involves the AI system being trained on a collection of unlabeled data which means that the algorithm does not possess the correct output for each data point. Therefore, it must independently recognize patterns and relationships within the data. For instance, unsupervised learning could be exploited to recognize customer groups with comparable spending habits.
Additionally, AI technologies have the capability to learn from data in real time. This is known as reinforcement learning. In this process, the AI system receives rewards for actions taken leading to favorable outcomes and punishments for actions taken leading to unfavorable outcomes.
Despite seeming logical, this methodology remains far from perfect and is not fully ready for a harsh world.
Is predictive AI ready for a such complex world?
A recent study published in Wired found that predictive AI technologies and their software applications, like Geolitica, are not as effective as one might hope. In fact, the study found that the AI software was only accurate about 0.6% of the time, which is no better than flipping a coin and hoping it lands on its side. This raises concerns about the use of AI technologies by police departments across the world.
To be more specific, the New Jersey Police Department used Geolitica, previously known as PredPol, between February 25 and December 18, 2018, to examine robbery occurrence rates in areas where no police officers were on duty, but it was found to have an accuracy of less than 100 out of 23,631 cases.
Plainfield PD captain David Guarino stated that the functional use of AI technologies is actually not that extensive by saying:
“Why did we get PredPol? I guess we wanted to be more effective when it came to reducing crime. And having a prediction where we should be would help us to do that. I don’t know that it did that,”
“I don’t believe we really used it that often, if at all. That’s why we ended up getting rid of it”.
So where was the problem? Well, one of the main issues with predictive AI software is that it relies on the flawed assumption that life is predictable. Life is a complex phenomenon influenced by numerous variables, many of which are unpredictable. As such, it’s difficult to rely on software to accurately forecast where and when an event is set to occur.
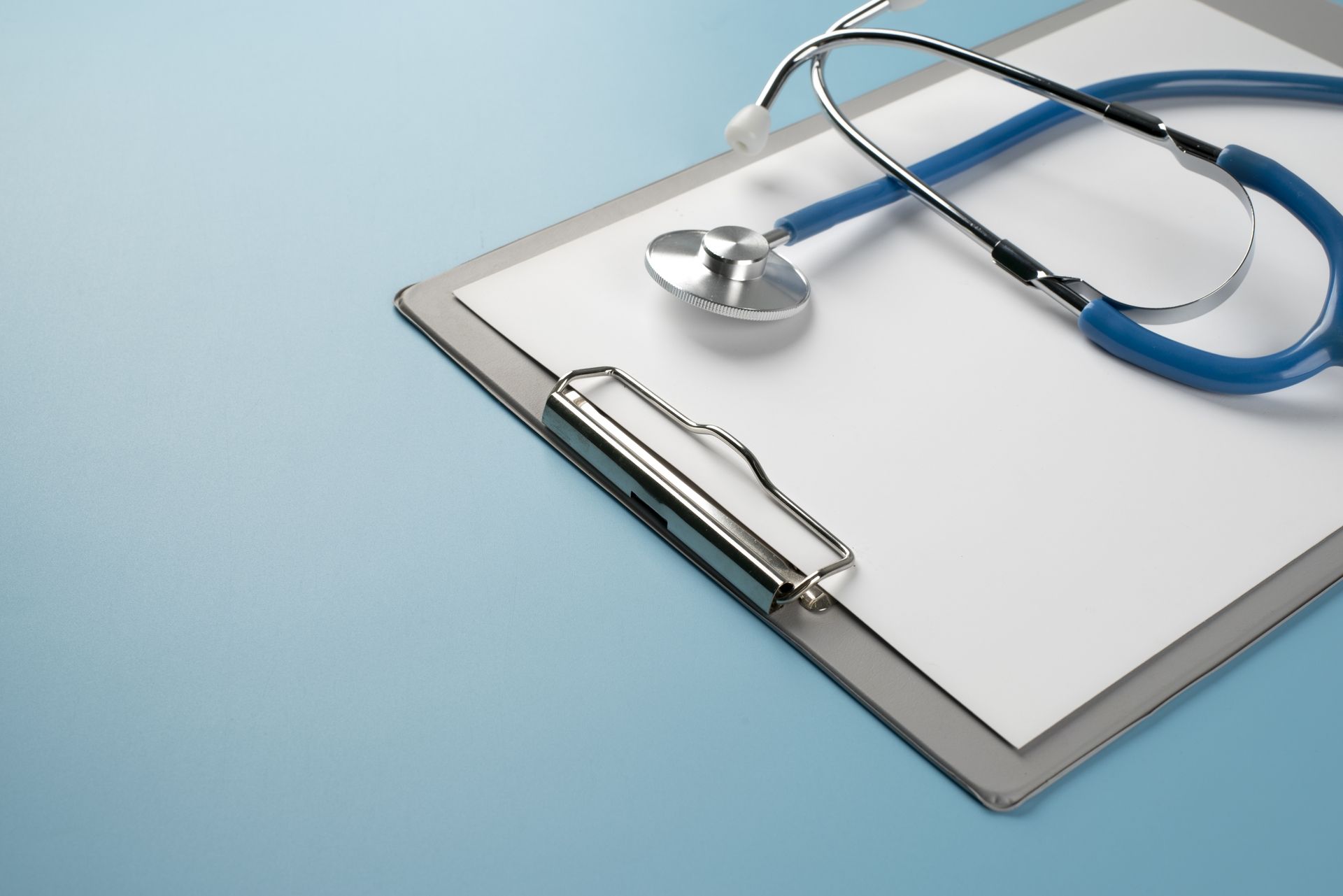
The situation for AI technologies is not yet very favorable in the field of medicine either. ChatGPT, which is widely used and is generally considered to be the most powerful large language model (LLM), is very misleading and inadequate for medical research and accurate diagnosis.
The study by Maryam Buholayka, Rama Zouabi, and Aditya Tadinada evaluated the ability of ChatGPT to write scientific case reports independently. The study compared ChatGPT’s performance with that of human oral and maxillofacial radiologists in writing case reports.
Quote on quote from the study, the drafted case report discussed a central hemangioma in a 65-year-old female and focused on the imaging features seen in a panoramic radiograph, cone beam computed tomography (CBCT), and magnetic resonance imaging (MRI).
ChatGPT was prompted in five separate chats. The format of the first question was structured depending on the outcome of the previous chat and the results were as follows:
Chat number | Case report provided | Deviation | Target audience | Imaging parameters/ technique | Key findings |
1 | Yes | Not specified | Not specified | Not specified | Inaccurate final diagnosis |
2 | Yes | No | Not specified | Not specified | Failure to comprehend patient confidentiality |
3 | Yes | No | Medical and dental radiologists | Not specified | Conversation discontinuity |
4 | Yes | No | Medical and dental radiologists | CBCT and MRI* | Subsection on limitations |
5 | No | No | Medical and dental radiologists | CBCT and MRI* | Fabricated references |
They found that ChatGPT was able to produce case reports of similar quality to those written by human radiologists. However, ChatGPT case reports were less likely to include certain important elements, such as a discussion of differential diagnosis and a review of the literature.
The comprehensive study concluded that ChatGPT is not yet ready to write scientific case reports on its own. So what do all these practices mean to us? Although AI technologies have wowed everyone with their magic, they are still far from perfection. Many unpredictable components, such as human control of the source and incorrect or incomplete operation of the algorithm, are slowing down the integration of AI technologies into everyday life.
But let’s not forget, even doors with sensors once seemed like a figment of our imagination.
Featured image credit: jcomp/Freepik.