Stephan Schnieber, Sales Leader IBM Cloud Pak for Data – DACH at IBM Deutschland, explains four ways to gain more trust in AI in our interview.
You and your team monitored a workshop on the topic of trustworthy AI today – at Europe’s biggest data science and AI EVENT, the Data Natives Conference in Berlin, DN22. How was that?
It was very, very good- I think it was really successful overall. Successful in the sense that collaboration can be extremely difficult in a workshop setting. We had a lot of interaction with participants, a full house, so to speak. The room was completely booked, occupied and everyone really participated.
We had an interactive session at the beginning to collect peoples’ points of view. There were some important ground rules that we had to set together as a group- How do we define trust? How do participants touch base if they need to? And once we established that, the workshop became a dynamic, flexible environment to collaborate within. What’s more is that we were actually fully subscribed, which I didn’t expect at all. The workshop was run by three of us from IBM and we were all excited by what we saw.
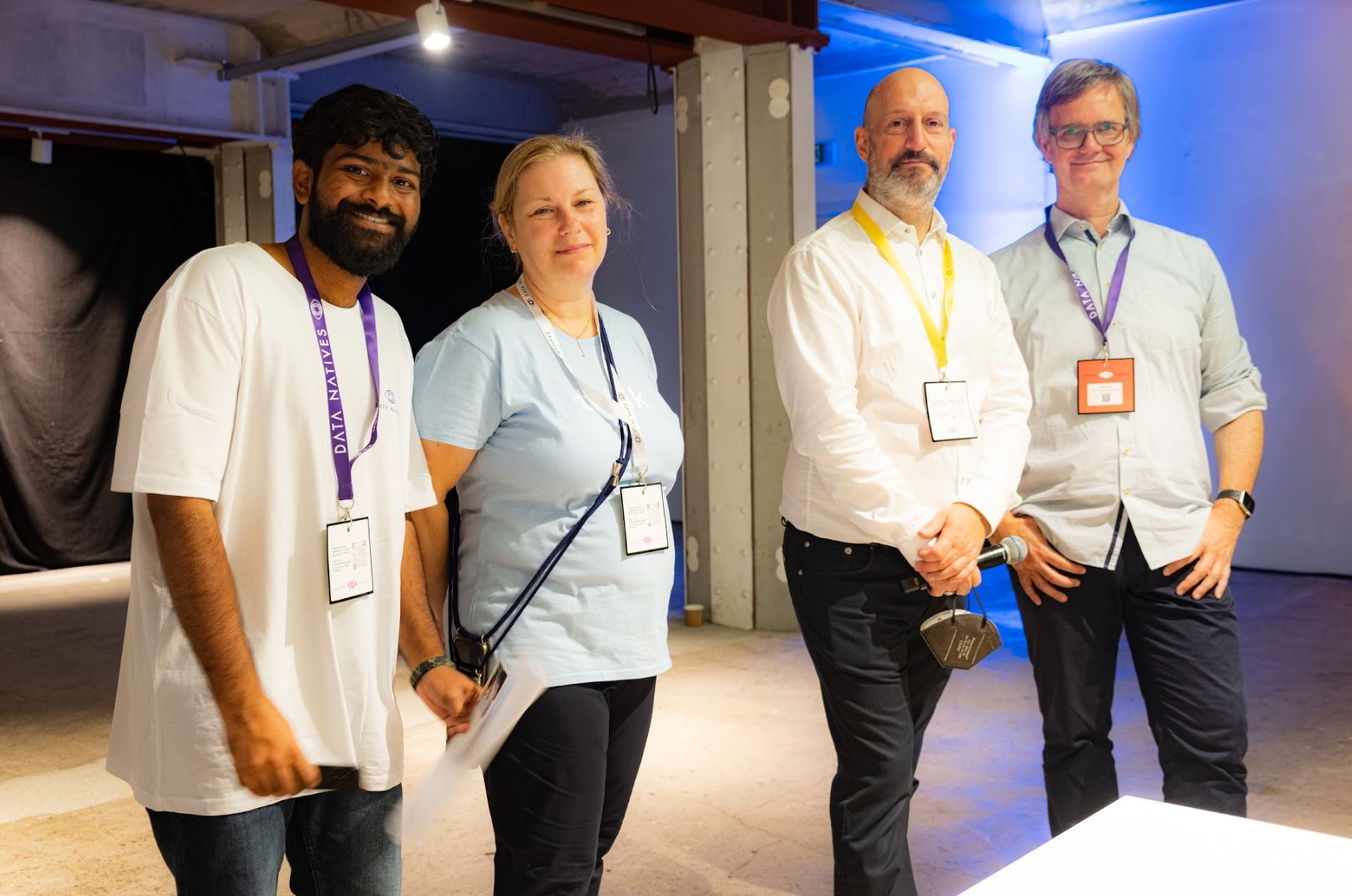
Afterwards, we held another meeting so we could explore a few of the themes we unearthed during the workshop. There were a lot of questions that participants still wanted answers for. So we really facilitated a forum for that. What are their views on trustworthy AI? What are their challenges, their encounters with it? We also used the opportunity to discuss a few recurring ideas that we picked up on; how do we understand our participants’ answers? What solutions can we offer? Because many of the points they raised have been ongoing conversations around AI for some time.
As well as this, IBM has a product offering that supports trustworthy AI, and we were able to present some of it during the workshop and at the same time collect feedback for it. So it was a really exciting, interesting 90 minutes.
What was the workshop participant structure like?
What I like about Data Natives is the diversity of attendees, which is actually better than what I experience in some client meetings. It’s that same lack of diversity that can make the IT sector boring. And of course, the relaxed atmosphere at the conference really helped. In terms of age structure, we had a lot of younger participants, Generation X and even Gen Z, which was something else I found pretty interesting.
‘Trustworthy AI’ means different things to different people. What does trustworthy AI mean at IBM?
My personal opinion happens to align with IBM. So the objective of Trustworthy AI is to integrate AI into business, to make sure that IBM supports their decision making with AI methods, both automated and partially automated. That’s the basic idea. The real question is how might we achieve this? The most important thing we can do is to build “trust” in AI as a decision maker or stakeholder. It’s ultimately about proving AI trustworthiness, so that when a decision is made, you don’t feel the need to second-guess it and say, “Hm, help, why do I feel unsure about this?” So that means I have to be able to say confidently that I’d be willing to allow an increasing amount of automatisms in my work and have my business processes supported by AI. That’s the real idea behind it.
And that’s why, as IBM sees it, AI begins with data. So first and foremost, we need to ensure the quality of data flowing into the AI systems. Meaning that we don’t just look at AI and the model, the modeling and subsequently the distribution of the models, but that the success of all of these prior steps begins with the data and the quality of said data. That’s why collaboration is so important in the field of AI.
As I said before, diversity massively enriched Data Natives as a conference, and my workshop really depended on a wide variety of influences within our audience, listeners, and participants to broaden the conversation around AI. As you might imagine, my team found that stimulating discussion during the workshop was directly impacted by these diverse backgrounds and ideas.
So in the end, the very foundations upon which our workshop was conducted came from understanding the value of a truly collaborative platform.
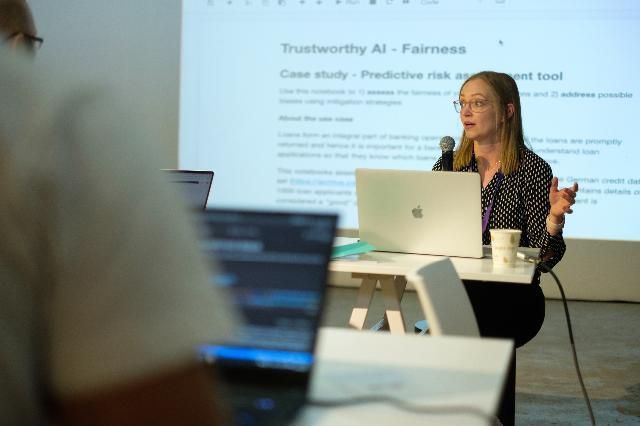
I believe that collaboration is one of our most important tools for supporting the development and acceptance of AI. There are many approaches to AI within the sector that tend to run in a stand-alone format and fail to utilise the innovation offered by teamwork. So for us at IBM, collaboration is an essential component to AI advancement. In team-based projects, you can bring people in, take them away. I can control who sees which data on which project, or who can see which AI models, and so on. So collaboration is really key to everything. Of course, collaboration across roles is equally important, because, if you think about all the different job titles involved in checking the quality of data- I have to prepare my data, develop it, maybe even transform it. And that’s just the beginning. We have to work really closely with data scientists, so it’s crucial that their attitudes to inter-role collaboration are just the same as ours- he or she has to get accurate preliminary work and be able to coordinate with colleagues. It’s these human skills that dictate the success of AI. And then, of course, we’ve got our new tool, IBM’s Cloud Pack for Data Platforms. The Pack enables you to collect, organise and analyse data no matter where it’s stored, so it really supports the collaborative environment we’ve worked hard to create at IBM.
Auto AI: This is a tool we use for a platform approach, dealing not only with data science, but data transformations too. Data science is pretty much a catch-all term, used lovingly by those amongst us who are what I like to call “no code people”. Which is basically anyone who isn’t a data scientist. Of course, “no-code people” need AI too, so for them, we’ve created a software feature called Auto AI. Our competitors call it Auto ML, but we call it Auto AI.
Auto AI makes it very, very easy to input data. I simply tell it what I need it to do, give it my target values, and the Auto AI automatically formulates a model for me, which, as research suggests, is highly likely to be accurate. After using the initiative myself, I’ve seen firsthand that the results generated by the AI are so reliable that I’d be confident in using them in a productive setting. We’ve also had major customers, mainly DAX companies, who have enjoyed remarkable successes with our Auto AI.
We also deal with Low-Code, which is obviously well-known as a component of graphic design. Our SPSS software can be considered a part of this platform, and it enables people to work graphically and develop stronger data and AI models. Of course, this fits into the broader idea of coding as a programmatic approach, seen in recent years with Teyssen, Jupyter Notebooks, AR and CoR Studio. Visual Code means we can improve data integration and API to enhance our flexibility and interconnect the different spheres of data. What’s more, being able to demystify concepts like Low-Code gives more people individual access to machine learning.
Trustworthy development models
This creates an environment for development which allows me to collaborate with other people, which is really crucial in the data science sector. Of course, it’s all about controlled collaboration: when it comes to data, privacy is everything. Whilst collaboration can enrich our understanding of data, it’s equally important that I can control which data I should mask, and who should or shouldn’t have access to it.
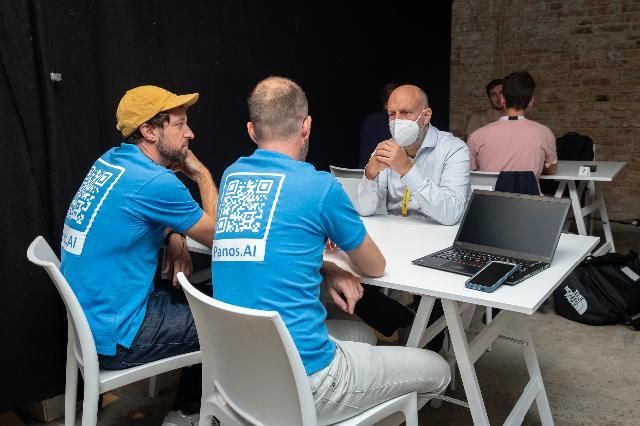
Creating a trustworthy development model means that I can protect personal data like e-mail addresses and telephone numbers in a secure way. That I’m able to control everything in a clean, reliable manner is essential, in turn, for establishing trust in the AI systems we use at IBM. When it comes to operations, I have to be confident in deploying my models. There’s been times where I’ve asked customers, “Have you started with machine learning? How many of the models are integrated into processes?” And it’s very few. Several companies are on their way, but in operation it’s a big construction site.. That’s where we can really start developing our processes.
What are the most important points to gain more trust in AI?
There are 4 points: Bias Detection, Data Drift, Transparency, Explainability.
Bias Detection: I’ll start with the topic of bias detection. Bias is ultimately the question: Are women and men treated identically? Are young people treated the same as old people? We also discussed this in the workshop. For example, with car insurance, it’s always the case that women get cheaper car rates. This has to be reflected and I have to ensure fairness in my modelling, and make sure that I get usable models and results that reflect reality. I have to build trust and acceptance in such a machine learning model.
Data Drift: After I created the model and put it into production, I had to factor into my machine learning model events for which we could never have planned. Take Covid, or the war in Ukraine, for example. Consumer behaviour changes completely. My entire machine learning model may become unusable because the behaviour of the users has changed. So I have to retrain my models and adapt them to the corresponding framework conditions. Detecting this automatically is done via data drift. I can set threshold values and then there is an alarm signal, which we can then monitor- nowadays, I can even ensure that I have automatic re-modelling and redeployment. So I can operationalise that too, if you like.
Transparency and explainability go hand in hand. I need transparency. Which decisions were made when? On the basis of which model, for example? So if I’m talking about data drift, how can I find out which machine learning model was used the day before yesterday? What data was used? How was the decision made? I need to know all of these things. It has to be transparent and sustainable. We always need to know what values were inputted, what model was used, what came out, in order to be able to trace it. There’s no use in saying, “Oh, something happened and I don’t know what to do.” We need to have total transparency in what we’re doing, and everything needs to be traceable. I always have to be able to explain the decisions I’ve made.
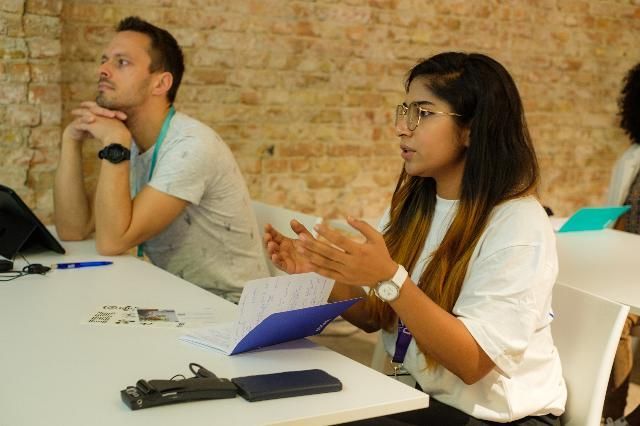
Do you have an example of this?
Say I apply for a bank loan and they say no because I’m just too old. The bank advisor who makes the decision thinks I won’t live long enough to pay back the 5 million I asked for. If I disagree with him, however, there’s a chance that I might be able to get the decision reversed.
With an AI model, I have to be able to map similar things. This means that, in principle, when I make a call, I don’t have to talk to the AI within the machine, but I do have to talk to the human at the end. But my friend at the other end of the line has to be able to understand the system, Mr Schnieber was not allowed to take out the bank loan due to his age. These are things that system transparency allows us to deliver. In principle, our system gives an indication that if the parameter of age is changed, then it’s very likely that different values would have emerged and that our bank manager would have reached a different conclusion.
With neural networks, it’s now quite important to have the ability to explain processes. Meaning that it’s something we have to factor in. And the bank advisor would then be able to tell me that the result on my loan was decided by a machine, that he decided against it, but that age is the main factor. And there’s nothing the bank can do about my age. It’s difficult. But if I offered them more equity now, for example, or if I were willing to reduce my loan amount, then the bank could reduce five million to three and a half. And we both make compromises. So I can use this example to present the issue of transparency, the need to understand AI systems, and how this applies to customer interactions with our software.
Of course, I have to be careful with data. What I’m really saying is that, if I build a model, I have to create something that I’m able to trust. And trust takes a lifetime to build but a second to lose. And that’s why I have to create a stable environment for innovation and development. Because when we do that, that’s when we can really create a secure platform for democratising and expanding knowledge around AI, as well as increasing software accuracy.