- Yellow AI, a conversational AI platform, recently released DynamicNLP, a solution that aims to do away with the need for NLP model training.
- Businesses don’t need to manually classify data to train the AI bot because the tool’s zero-shot learning (ZSL) architecture does it for them.
- Instead, it allows dynamic AI agents to pick up new skills as they go, enabling them to swiftly set up conversational AI flows while using less training data, resources, and time.
Yellow AI says that issues related to NLP training can be dealt with conversational AI flows. Consumers can converse with computer programs in the same manner they would with other people, thanks to conversational artificial intelligence (AI). Advanced chatbots, or AI chatbots that differ from traditional chatbots, are the most common form that conversational AI has taken.
Training agents manually is one of the major setup problems that artificial intelligence (AI) teams encounter. Because all training data for current supervised methods must be manually labeled, it takes time and money. 96% of respondents to a poll by Dimensional Research and AIegion report having dealt with training-related problems such as poor data quality, labeling needed to train the model, and developing model confidence.
This problem has taken center stage for various language-based use cases as the field of natural language processing (NLP) expands gradually thanks to developments in deep neural networks and sizable training datasets. Yellow AI, a conversational artificial intelligence platform, recently announced the release of DynamicNLP, a solution intended to do away with the requirement for NLP model training to address this issue.
Establishing conversational AI flows saves time and money
Because DynamicNLP is a pre-trained NLP model, businesses can benefit from not having to waste time continuously training the NLP model. The tool’s zero-shot learning (ZSL) architecture eliminates the need for businesses to spend time manually classifying data to train the AI bot. Instead, it enables dynamic AI agents to learn as they go, quickly establishing conversational AI flows while using less training data, money, and time.
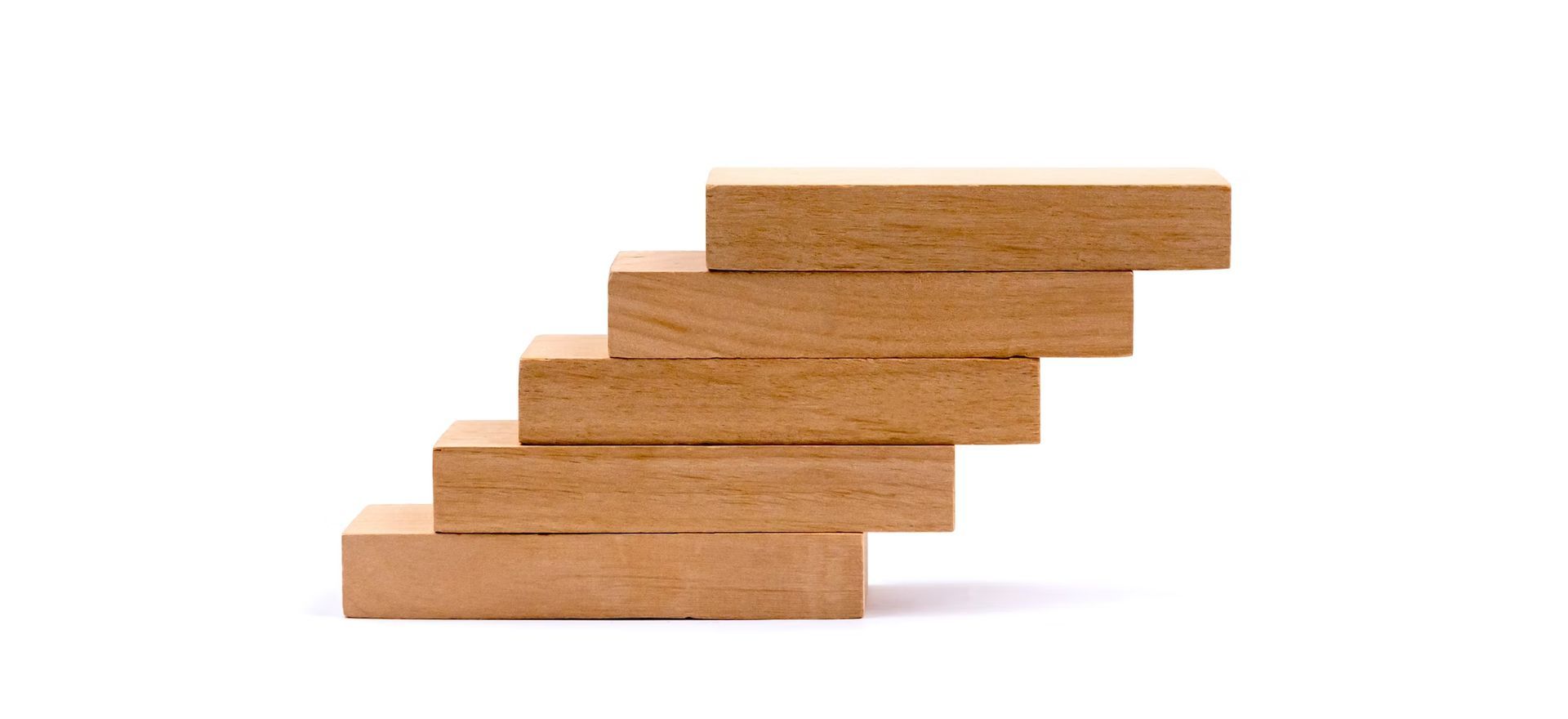
“Zero-shot learning offers a way to circumvent this issue by allowing the model to learn from the intent name. This means that the model can learn without needing to be trained on each new domain,” explained the CEO and co-founder of Yellow AI, Raghu Ravinutala.
He added that the zero-shot approach could lessen the requirement for gathering and annotating data to improve accuracy.
What are the obstacles to training conversational AI?
Intensive training is required for conversational AI platforms to deliver human-like conversations successfully. The chatbot model cannot provide the appropriate response unless new and updated utterances are continuously introduced. Hundreds to thousands of distinct data points must be manually used to train NLP to sustain the process for numerous use cases.
Natural language processing fosters new protein designs
It’s essential to continuously observe how users type utterances when using supervised learning algorithms to add utterances (a chatbot user’s input), slowly and iteratively identifying the ones that didn’t get identified. The missing utterances must be reintroduced into training after being tagged. Throughout the procedure, some inquiries might not be identified.
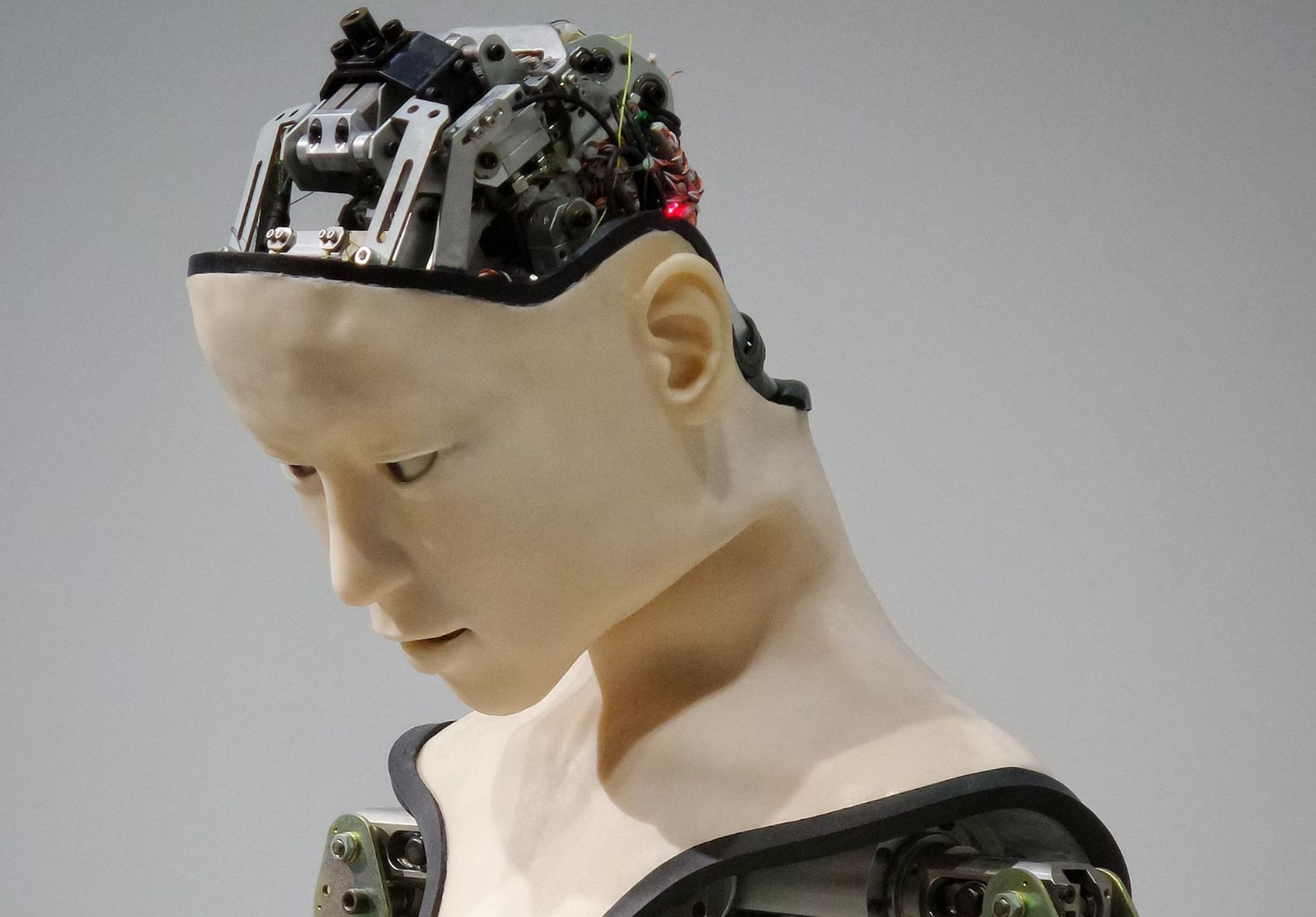
How utterances can be introduced presents yet another important challenge. There is still the issue of how many the chatbot will be able to recognize even after taking into account all the methods that user input can be registered.
To that goal, Yellow AI’s DynamicNLP platform was created to increase the precision of both visible and invisible utterance intents. In addition to reducing errors, removing manual labeling makes natural language processing stronger and more reliable, with higher intent coverage for all sorts of topics.
Google AI: Pathways Language Model can explain a joke
According to Yellow AI, the model agility of DynamicNLP enables businesses to maximize productivity and effectiveness across a wider range of use cases, including customer service, customer interaction, conversational commerce, HR, and ITSM automation.
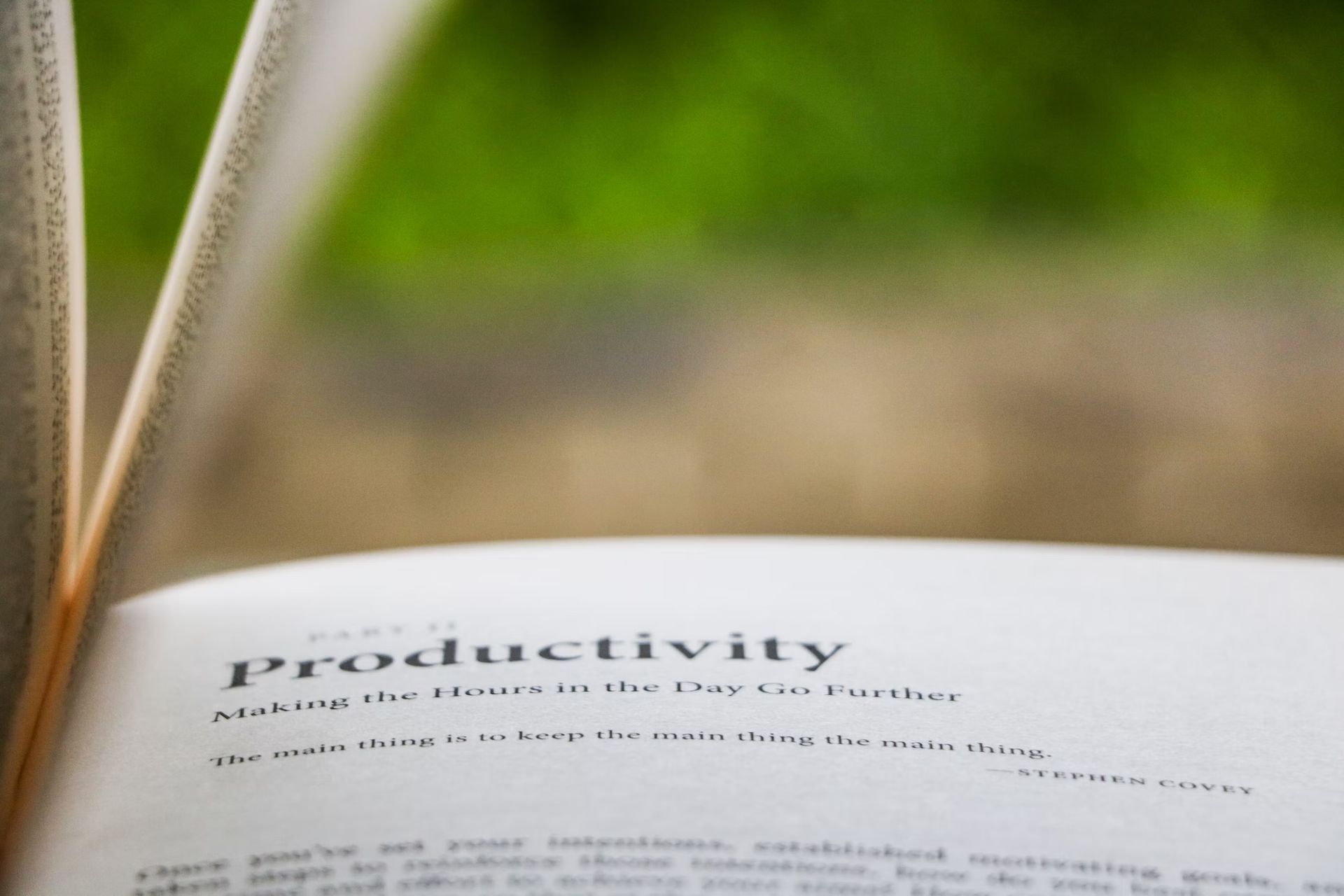
“Our platform comes with a pre-trained model with unsupervised learning that allows businesses to bypass the tedious, complex, and error-prone process of model training,” stated Ravinutala.
The pre-trained model is created from billions of anonymized interactions. According to Ravinutala, this reduces unidentified utterances by as much as 60%, giving AI agents a more human-like quality and making them more adaptable to different sectors and use cases.
Creating a human-like AI with metamemory skills is possible
“The platform has also been exposed to a lot of domain-related utterances. This means the subsequent sentence embeddings generated are much stronger, with 97%+ intent accuracy,” he said.
So what does the future look like?
The usage of pre-trained models to improve conversational AI development, according to Ravintula, will surely rise. These modalities include text, speech, video, and images.
“Enterprises across industries would require even lesser efforts to tune and create their unique use cases since they would have access to larger pre-trained models that would deliver an elevated customer and employee experience,” he said.
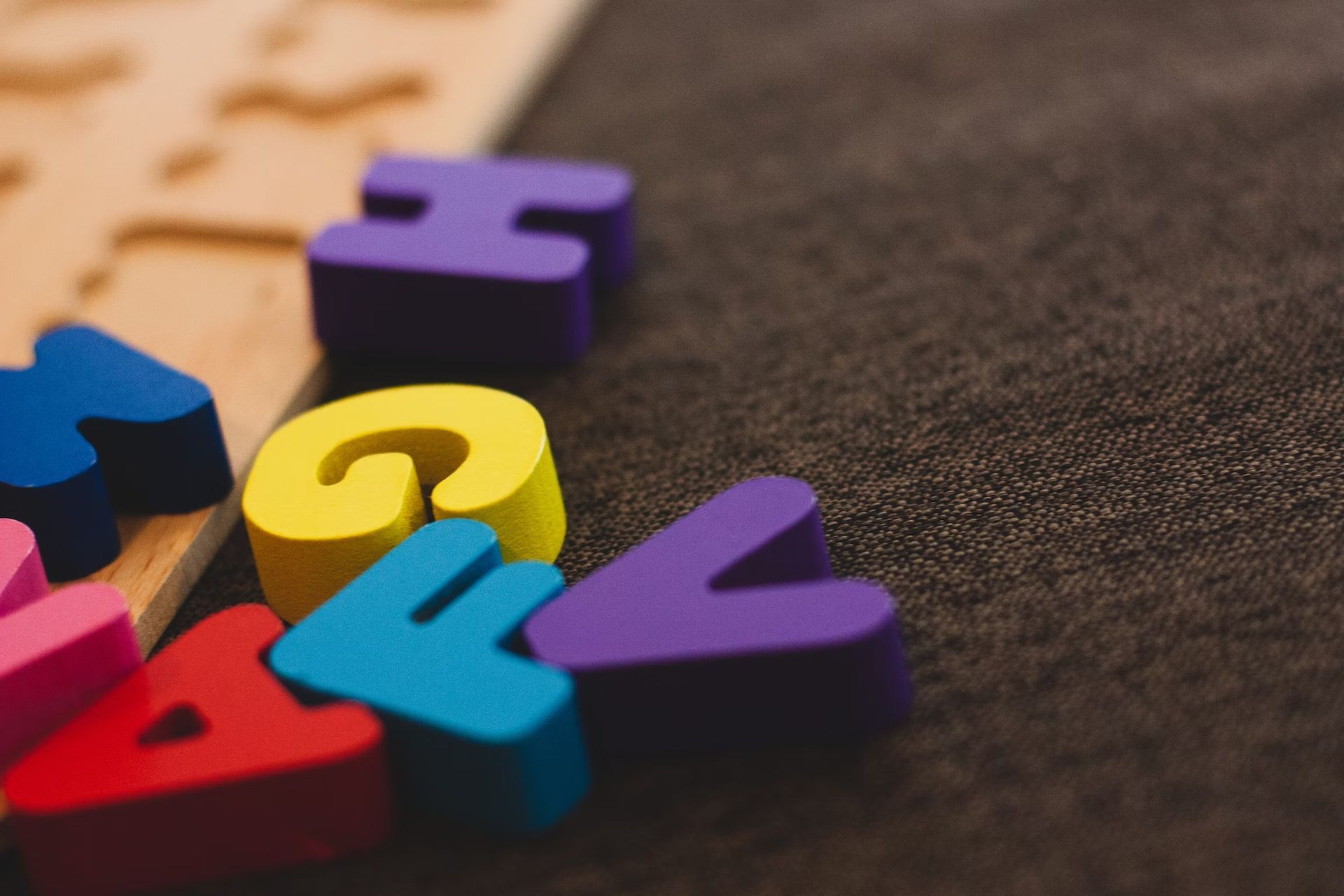
He noted that making models more context-aware is a contemporary problem, as language is ambiguous by its own nature.
“Models being able to understand audio inputs that comprise multiple speakers, background noise, accent, tone, etc., would require a different approach to deliver human-like natural conversations with users effectively,” he added.
Conclusion
In the end, conversational AI enables effective and automated human-like communication.
In recent years, this technology, the brains behind many virtual chatbots and online assistance systems, has gained popularity for enhancing customer service, sales, marketing, and the overall customer experience.
Businesses may quickly and effectively address common consumer inquiries and resolve difficulties with a conversational AI application.
While there are still some difficulties with discovery, adoption, and ever-evolving communication for conversational AI, these issues will eventually be resolved thanks to improvements in AI.