Researchers from Citadel developed a deep learning method to generate DNS amplification attacks. Deep learning algorithms have lately been shown to be quite effective at identifying and preventing cybersecurity assaults. Various deep learning techniques, such as those used for image classification and natural language processing, have been the target of numerous cybercriminals’ recent development of new attacks.
The most common of these strategies are adversarial attacks, which use altered data to deceive deep learning algorithms into categorizing it incorrectly. This could lead to the failure of numerous deep learning-based apps, biometric systems, and other systems. Researchers are creating new cybersecurity measures every day but protecting data has never been harder. For instance, a “tech NATO” is needed to guard cyber borders, according to Darktrace CEO.
Detecting DDoS DNS amplification attacks
Numerous earlier studies have demonstrated how various adversarial assaults can cause deep neural networks (DNNs) to deliver erroneous and inaccurate predictions. These assaults consist of the Elastic-Net attack, the fast gradient sign technique (FGSM), the Carlini & Wagner attack, and the Deepfool attack (ENA).
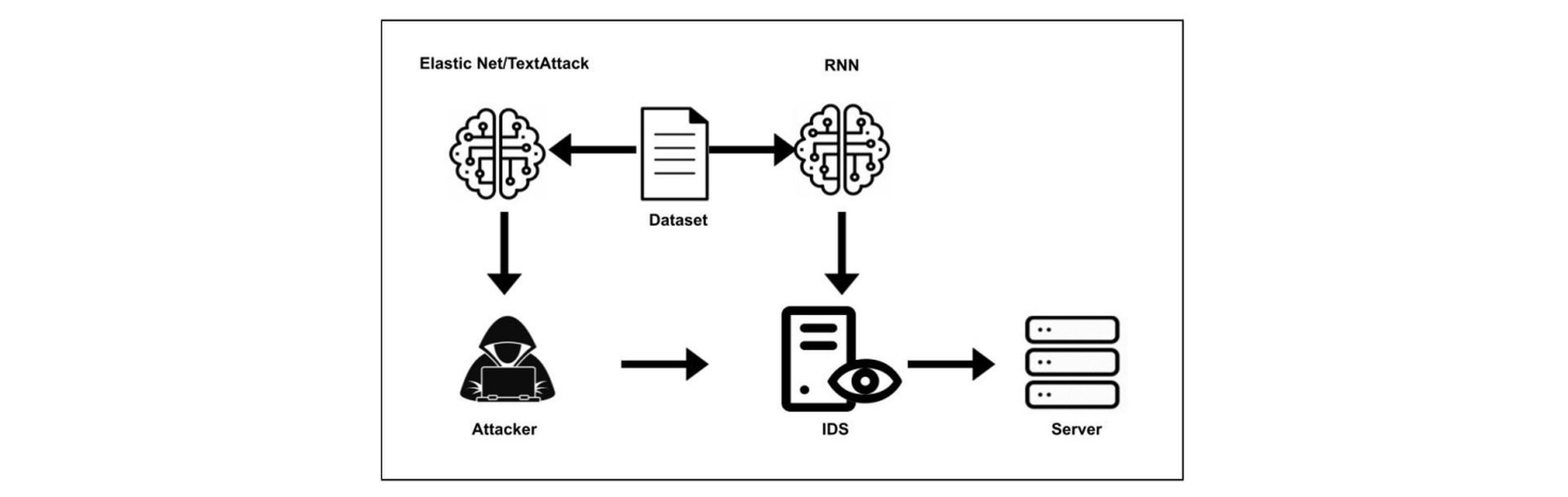
Recently, Citadel researchers built a DNN that can recognize a specific type of cyberattack called distributed denial of service (DDoS) DNS amplification, and they then used two distinct ways to create adversarial samples that can trick their DNN. Their findings, which were preliminary published on arXiv, show how inadequate deep learning systems are at detecting DNS intrusions and how vulnerable they are to adversarial attacks.
DDoS DNS amplification attacks amplify requests sent to DNS servers by exploiting their vulnerabilities in order to overwhelm the systems with data and bring them down. These attacks could seriously impede the internet services offered by big and small international companies alike.
Recent years have seen the development of deep learning systems for detecting DDoS DNS amplification attacks. However, the Citadel team showed that by using adversarial networks, these strategies might be avoided.
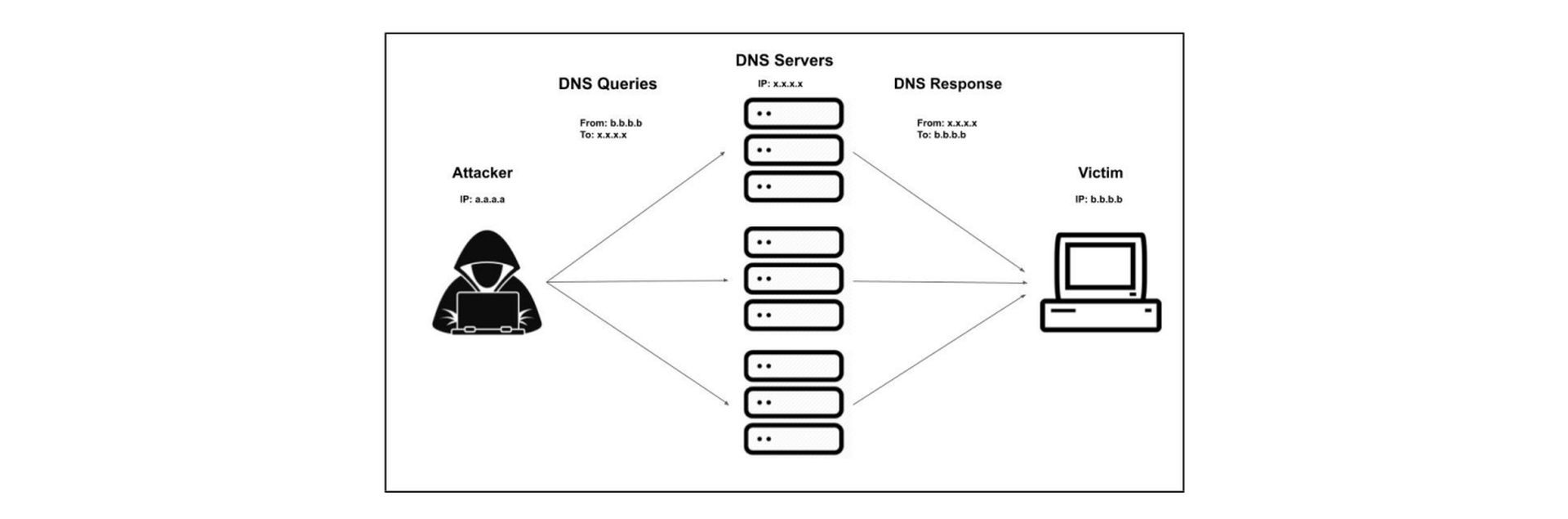
“Much of the current work in the field of adversarial learning has been conducted in image processing and natural language processing with a wide variety of algorithms. Two algorithms of interest are the Elastic-Net Attack on Deep Neural Networks (EAD) and TextAttack,” Mathews and his colleagues stated in their study.
Techniques like Elastic-Net Attack (EAD) and TextAttack have proven to be quite successful in generating corrupted data that DNNs would mistakenly classify. Using adversarial data generated by the EAD and TextAttack methods, Jared Mathews and his colleagues tried to fool a system by spotting DDoS DNS amplification attacks.
“In our experiment the EAD and TextAttack algorithms are applied to a Domain Name System amplification classifier. The algorithms are used to generate malicious DDoS adversarial examples to then feed as inputs to the network intrusion detection systems neural network to classify as valid traffic,” the researchers stated.
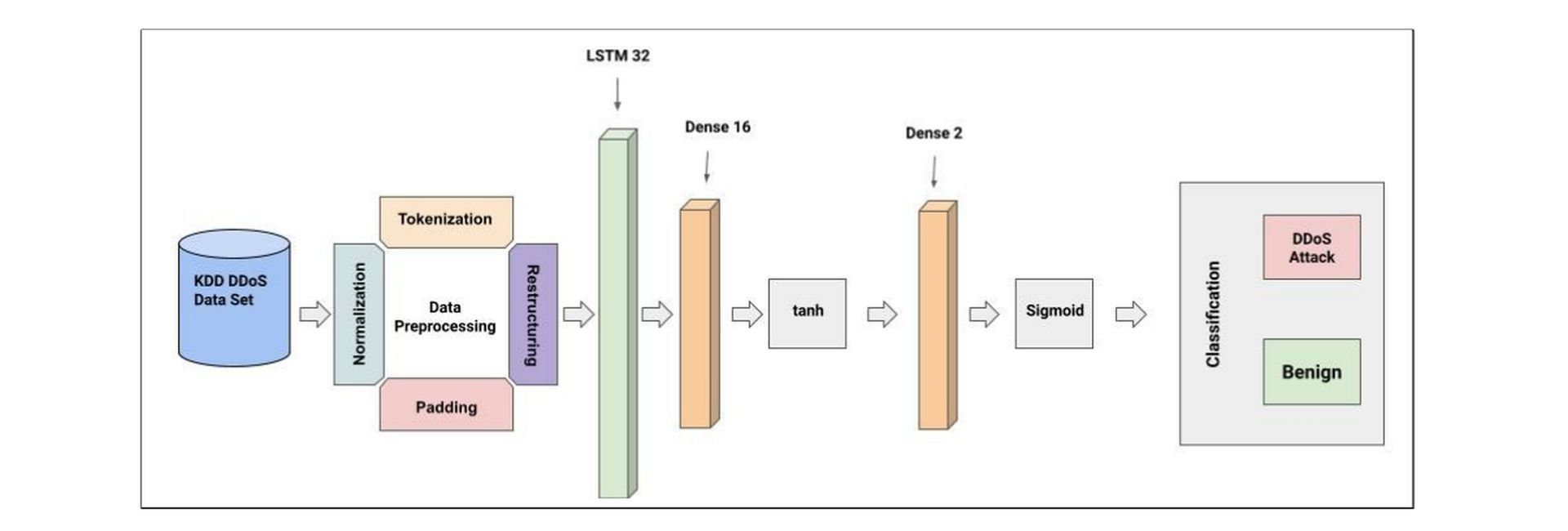
In their trials, Mathews and his coworkers found that the adversarial data provided by EAD and TextAttack could fool their DNN for DDoS DNS amplification attack detection 100% of the time and 67.63% of the time, respectively. These results highlight significant shortcomings and vulnerabilities in the current deep learning-based systems for detecting these intrusions.
“We show that both image processing and natural language processing adversarial learning algorithms can be applied against a network intrusion detection neural network,” the researchers stated.
Future systems for detecting DDoS DNS amplification attacks that can identify and classify hostile material may be inspired by the work of this Citadel team of researchers. In their further investigations, the researchers plan to assess the effectiveness of adversarial attacks on a particular method for recognizing DNS amplification attacks targeting the so-called limited application protocol (CoAP) used by IoT devices. Before leaving, don’t forget to check out the best cybersecurity practices for staying safe against today’s digital perils!