The AI created by the researchers will make it easier for doctors ask clinical questions to discover data in a patient’s health record.
Electronic health records are frequently consulted by doctors for data that aids in treatment choices, however the burdensome nature of these records makes this process difficult. According to research, even when a doctor has been taught to utilize an electronic health record (EHR), it can still take more than eight minutes to locate the answer to just one query. Thus, using the instruments provided by technology can be vital for the future of healthcare sector. For example, lately it is discovered that AI can tell what doctors can’t. Now it can determine the race.
The dataset is created with more than 2,000 clinical questions
Physicians have less time to connect with patients and administer care the longer they must spend navigating a frequently cumbersome EHR interface.
Machine learning algorithms are being developed by researchers to speed up the process by automatically locating the data that doctors require in an EHR. Effective models must be trained on large datasets containing pertinent medical questions, but these might be difficult to find because of privacy laws. Existing models frequently fail to produce accurate responses and have trouble coming up with real questions that a human doctor would ask.
To address this data gap, MIT researchers collaborated with medical professionals to explore the inquiries doctors make while looking over electronic health records (EHRs). Then, they created a dataset that is open to the public and contains more than 2,000 clinical questions created by these medical professionals.

They discovered that the model asked high-quality and authentic questions more than 60% of the time when compared to actual inquiries from medical specialists after using their dataset to train a machine-learning model to create clinical questions.
They want to utilize this dataset to produce a sizable number of real medical queries, which will be used to train a machine-learning model that can aid clinicians in more quickly finding pertinent data in patient records.
“Two thousand questions may sound like a lot, but when you look at machine-learning models being trained nowadays, they have so much data, maybe billions of data points. When you train machine-learning models to work in health care settings, you have to be really creative because there is such a lack of data,” explained the lead author Eric Lehman, a graduate student in the Computer Science and Artificial Intelligence Laboratory (CSAIL).
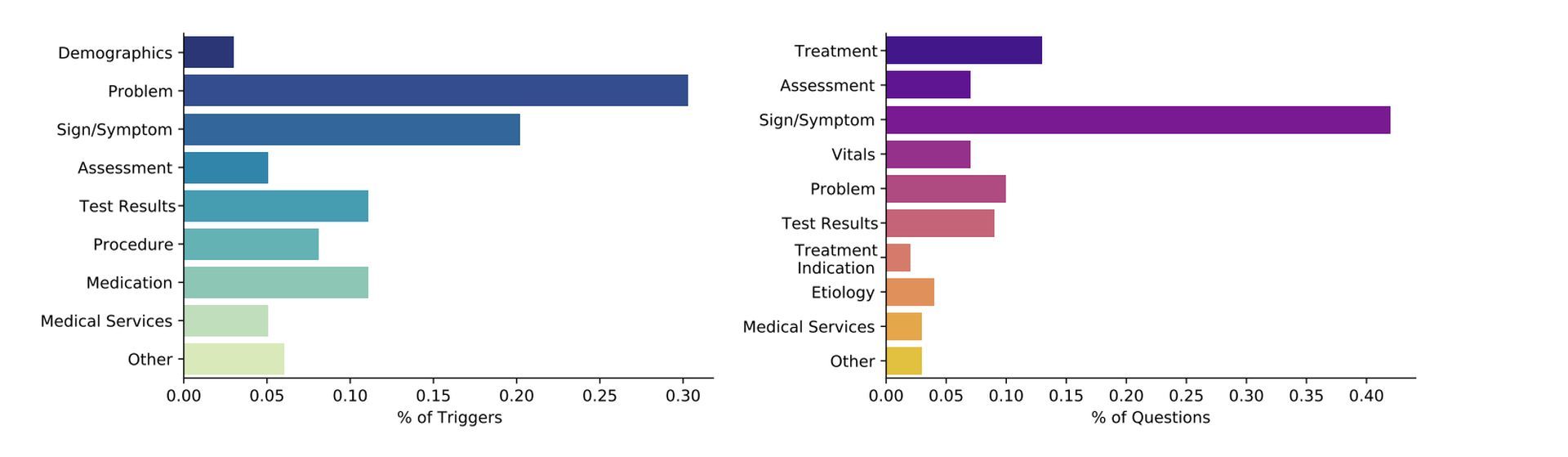
Professor Peter Szolovits, leader of the Clinical Decision-Making Group in CSAIL and a member of the MIT-IBM Watson AI Lab, is the senior author and a professor in the Department of Electrical Engineering and Computer Science (EECS). The study will be presented at the annual conference of the North American Chapter of the Association for Computational Linguistics. Its co-authors at MIT, the MIT-IBM Watson AI Lab, IBM Research, and the doctors and medical experts who helped develop questions and participate in the study worked together on the paper.
“Realistic data is critical for training models that are relevant to the task yet difficult to find or create. The value of this work is in carefully collecting questions asked by clinicians about patient cases, from which we are able to develop methods that use these data and general language models to ask further plausible questions,” said Szolovits.
Lehman says that there were a number of problems with the few sizable datasets of clinical questions the researchers were able to locate. Some of them were made up of patient-posted medical queries from online discussion boards, which are very different from queries for doctors. Other datasets have questions that were generated using templates, which makes many of the questions implausible.
“Collecting high-quality data is really important for doing machine-learning tasks, especially in a health care context, and we’ve shown that it can be done,” said Lehman.
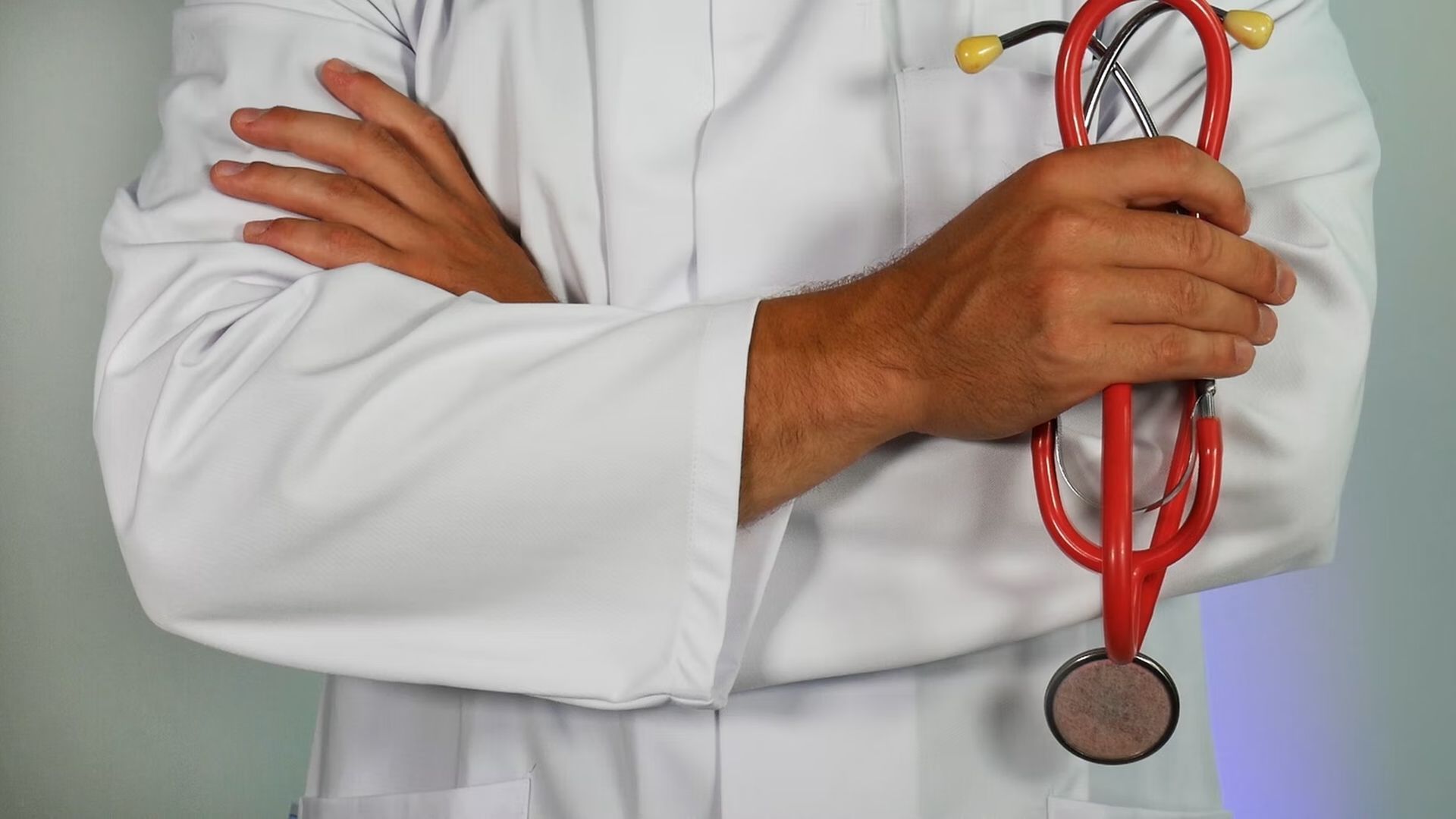
The MIT researchers collaborated with practicing physicians and final-year medical students to develop their dataset. More than 100 EHR discharge summaries were sent to these medical professionals, and they were instructed to look through each one and ask any concerns they might have. In an effort to obtain organic questions, the researchers didn’t impose any constraints on question forms or structures. Additionally, they asked the medical professionals to point out the specific “trigger language” from the EHR that prompted each query.
An expert in medicine may, for instance, read a note in the EHR that states that a patient’s prior medical history is important for prostate cancer and hypothyroidism. The expert could inquire about the expert’s date of diagnosis if the trigger word “prostate cancer” is used. perhaps “any interventions made?”
They discovered that the majority of queries were centered on the patient’s symptoms, medications, or test findings. Even if these results weren’t a surprise, Lehman believes measuring the quantity of inquiries about each major subject will enable them to create a useful dataset for use in a genuine, clinical environment.
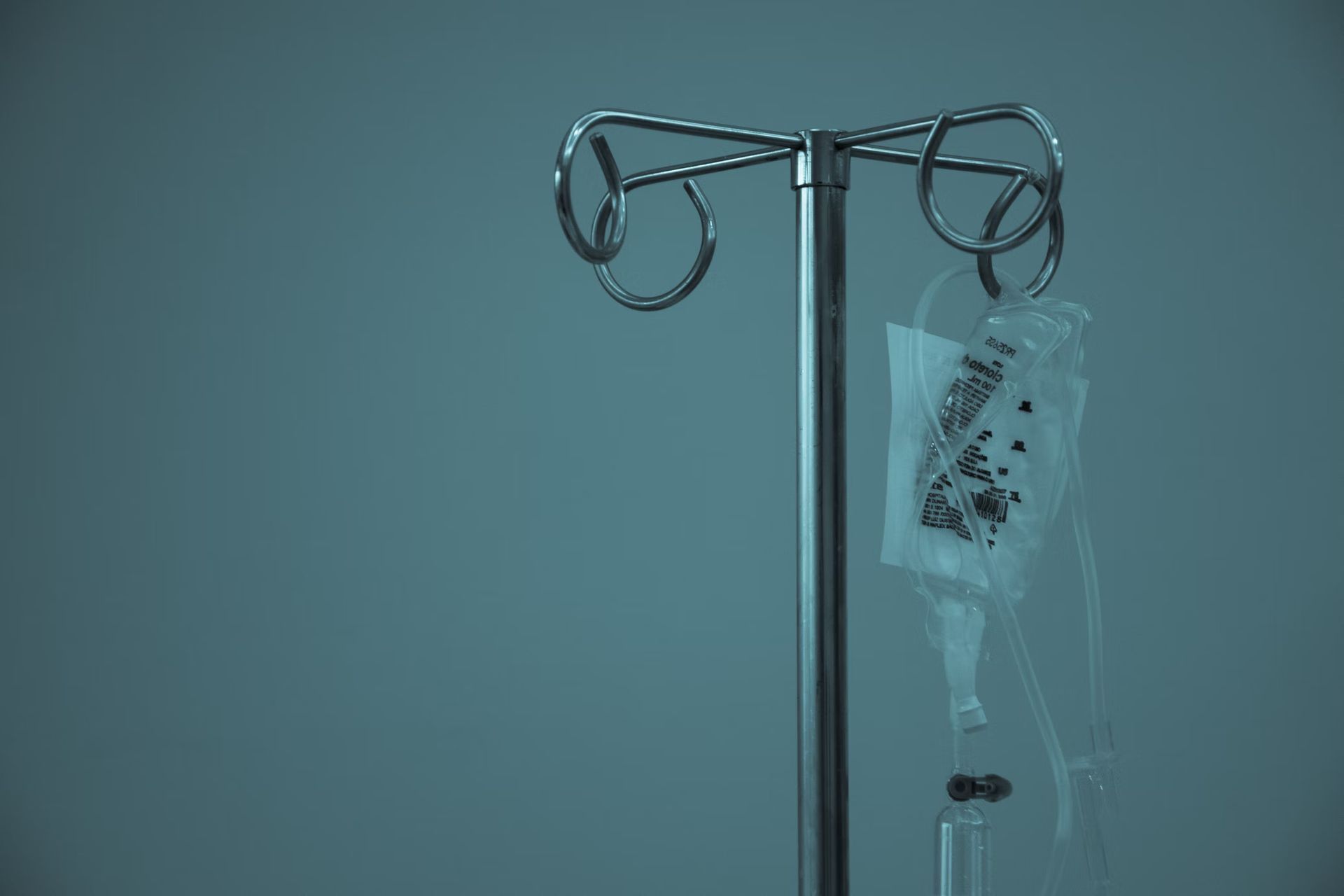
They utilized their dataset of test questions and related trigger text to train machine learning models that would generate new questions depending on the trigger text.
Then, based on four criteria, the medical experts determined whether those questions were “good” or not: understandability (Does the question make sense to a human physician? ), triviality (Can the question be answered by simply reading the trigger text? ), medical relevance (Does it make sense to ask this question given the context? ), and relevancy to the trigger (Is the trigger related to the question?
Researchers also trained models to retrieve answers to clinical questions
When a model was given trigger language, the researchers discovered that it could come up with a decent question 63 percent of the time, but a human doctor would ask a good question 80 percent of the time.
Using the publicly accessible datasets they had discovered at the beginning of this study, they also trained models to retrieve answers to clinical questions. They then put these trained models to the test to see if they could respond to “excellent” queries posed by real medical professionals.
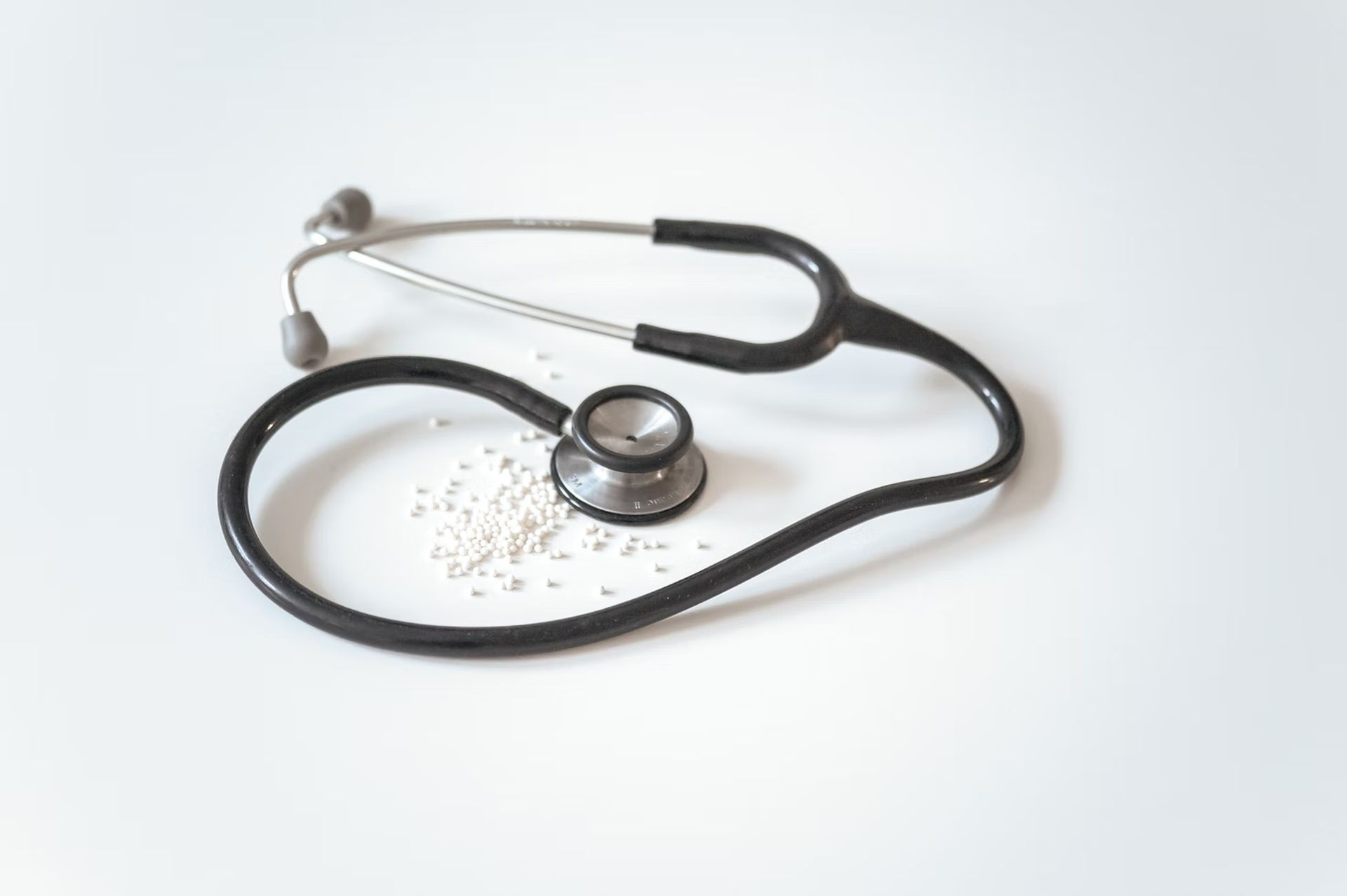
The models were only able to recover roughly 25% of the responses to the queries that the doctors came up with.
“That result is really concerning. What people thought were good-performing models were, in practice, just awful because the evaluation questions they were testing on were not good to begin with,” explained Lehman.
The team is now putting this work to use in order to achieve their original objective: developing a model that can automatically respond to physicians’ inquiries in an EHR. The following phase will include using their dataset to train a machine-learning model that can automatically produce tens of thousands or millions of high-quality clinical questions. This model can then be used to build a new model for automatic question-answering.
Lehman is inspired by the promising first findings the team showed with this dataset, even if there is still more work to be done before that model might become a reality. Healthcare is a sector where AI is highly utilized, for instance, did you know that neural network-based visual stimuli classification paves the way for early Alzheimer’s diagnosis?