Although machine learning (ML) has helped make blood cell counts more accurate and less costly, it has nevertheless been time-consuming because of the need for a lot of manual annotation by humans to train the model. Researchers at Benihang University, on the other hand, have developed a new training method that eliminates much of this work.
The ML method capable of counting cells aims to eliminate human involvement
On April 9, Ambionic’s new training program researchers published their findings in the journal Cyborg and Bionic Systems.
The number and type of blood cells and their count are so important in predicting disease. However, cell analysis techniques for counting blood cells that utilize the detection and measurement of physical and chemical characteristics of cells suspended in a fluid are time-consuming and need complex preparations. Worse still, cell analyzer machines have limited accuracy due to external factors such as temperature, pH, voltage, and magnetic field that may interfere with the equipment. Long story short, researchers have aimed to develop an ML method capable of counting cells. This way, both human involvement will decrease, and estimates will be much more accurate.
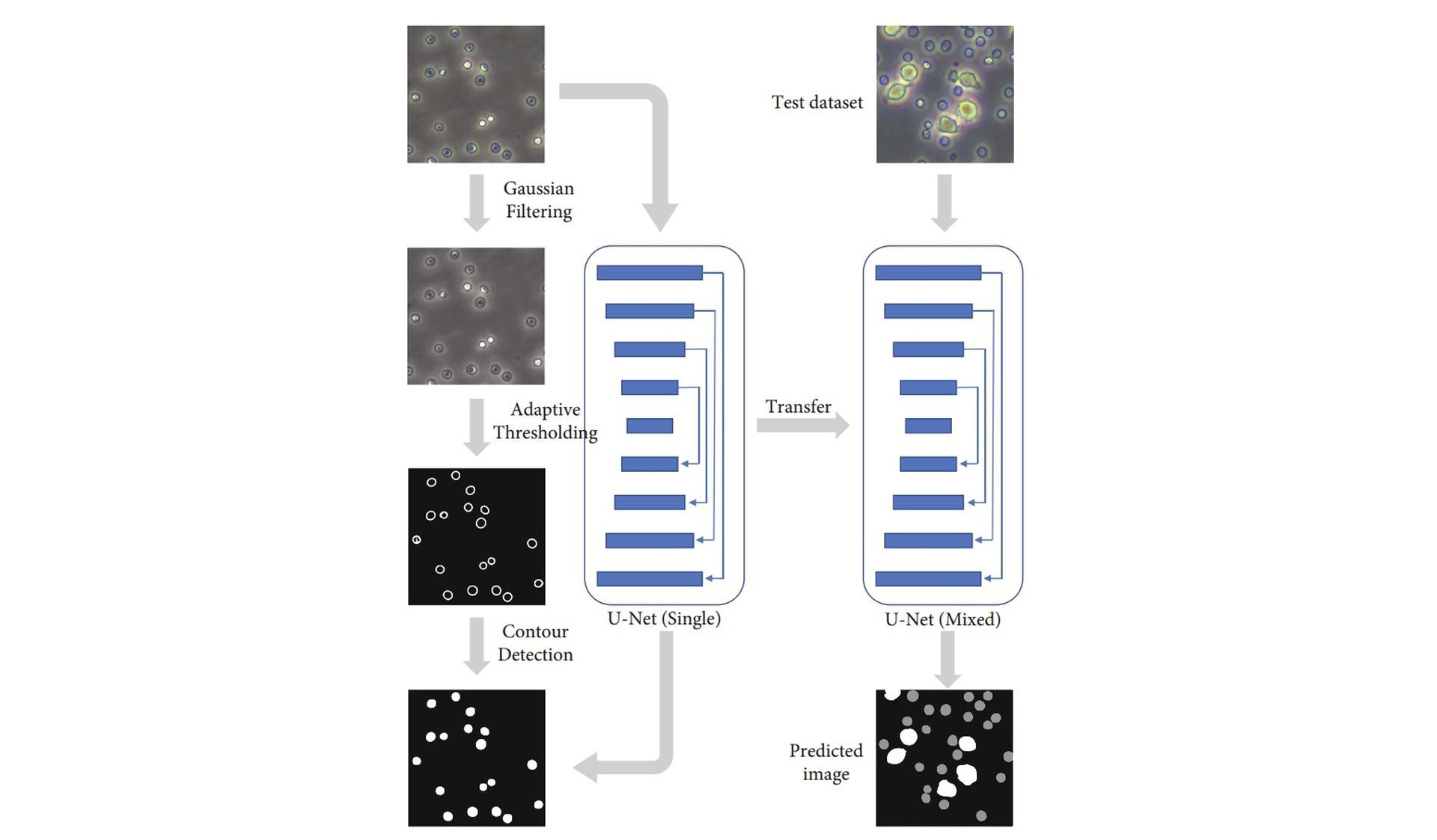
Much research into alternatives to replace the current blood testing technique has lately focused on “segmentation” of photographs taken by a high-definition camera connected to a microscope using computer programs. Segmentation entails algorithms that count the number of cells in an image, known as counting pixels.
Convolutional neural networks (CNNs) have been used to identify cells in photos that include only a single type of cell. However, when applied to pictures with many types of cells, they perform rather poorly. So, to address the issue, researchers have turned to CNNs, a kind of machine learning that replicates the human visual cortex’s connection architecture.
To identify a cell, the CNN must first be “trained” to recognize what is and isn’t one on thousands of images of cells that humans have labeled. It then recognizes and can count the cells in a new, unidentified picture.
“But such manual labeling is laborious and expensive, even when done with the assistance of experts,” explained Guangdong Zhan, a co-author of the paper and professor with the Department of Mechanical Engineering and Automation at Beihang University, “which defeats the purpose of an alternative that is supposed to be simpler and cheaper than cell analyzers.”
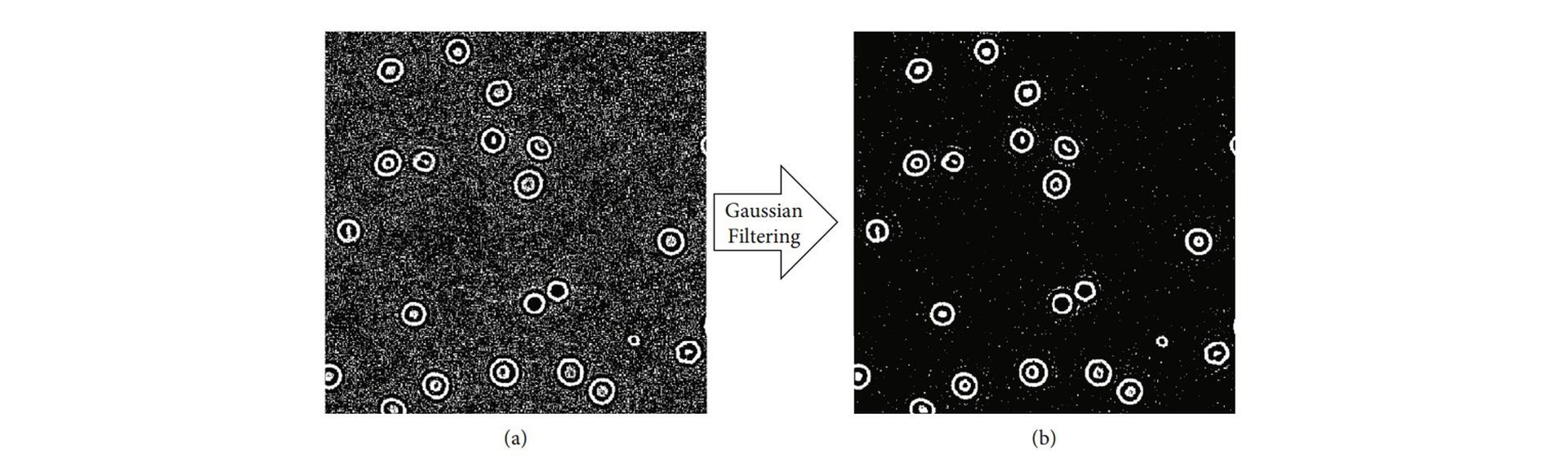
So the researchers at Beihang University sought to improve the U-Net model, which is a fully convolutional network segmentation model that has been widely utilized in medical image segmentation since it was first developed in 2015. Lately, we’ve learned that ML systems could detect deadly earthquakes swiftly.
The CNN is first trained on a large set of thousands of images containing only one type of cell (mice blood).
Conventional algorithms automatically “preprocess” single-cell-type pictures by reducing noise, improving quality, and detecting the shapes of objects in the photos. After that, they execute adaptive image segmentation. This latter technique determines the various shades of gray in a black and white picture and separates anything that falls outside a certain gray level as a distinct object if it has more than half of its area within this threshold. The adaptive aspect of this approach is that instead of dividing the image into pieces based on a specific gray threshold, it does so based on local features.
The U-Net model is fine-tuned after the single-cell-type training set is fed, using a small collection of manually labeled pictures of various cell types. In contrast, compared with the previous version, which required thousands of images to be labeled by humans, this version only requires 600 instead.
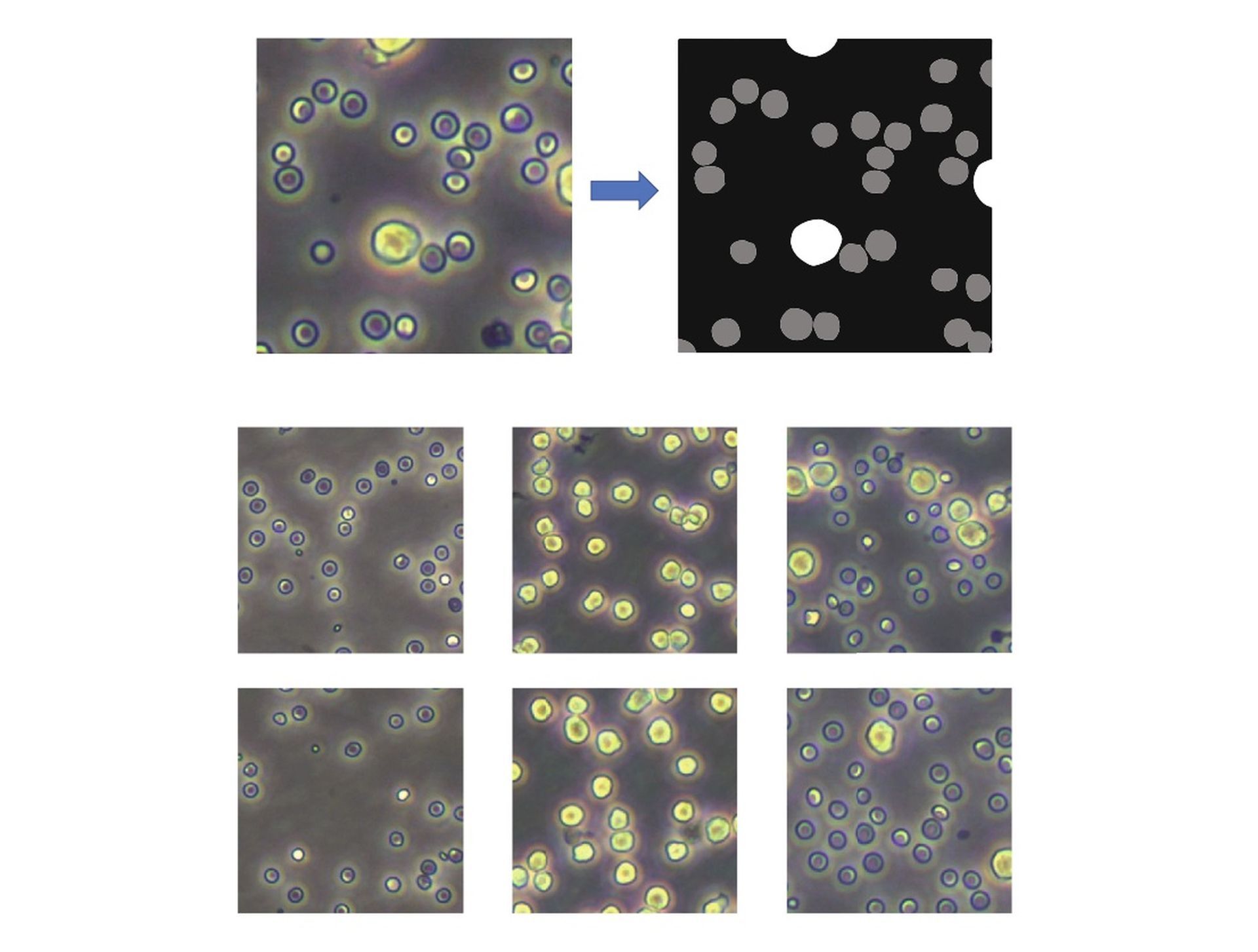
The researchers used a conventional cell analyzer on the same mouse blood samples to evaluate their training strategy. They performed an independent cell count to compare it with their new method. They discovered that their segmentation of multiple-cell-type images training scheme had a 94.85 percent accuracy, which was comparable to that obtained by learning from manually labeled multi-cell-type pictures.
The method may be used on more sophisticated models to tackle complicated segmentation issues. The researchers aim to develop a completely automated algorithm for training machine learning models based on the new training technique, which still involves some degree of manual annotation. The medical industry is not the only benefit of machine learning systems. For instance, researchers used ML to detect Professional Malicious User (PMU) reviews.