While many people think of abstract ideas regarding artificial general intelligence (AGI), this technology has arrived at an important crossroads today. In fact, scientists stunned by its incredible potential agree to disagree on how the future of AGI should be shaped.
Disagreements about the future of technologies, especially the ones that affect other technologies with convergence and share the digital transformation burden of the world, usually end with finding efficient and cost-effective options. There are many reasons why this is not the case in artificial intelligence. At the heart of all, we humans have been dreaming of this for a very, very long time. My previous article examined the fantastic precursors of artificial intelligence that date back centuries and cover many magnificent ideas, from giant smart robots to attempts to create willful beings in bell jars.
Tug of war
For many centuries, artificial intelligence research has revolved around the human desire to create smart things as smart as the smartest creature they know, you guessed it right, they meant themselves. The contemporary concept of artificial intelligence is built on the idea that human thought can be mechanized. However, at this point, some of the brightest minds of our time think that the ideal route for artificial intelligence might not be replicating the human mind. And these differences are not limited to the theoretical ideas: Many contemporary schools of thought are accomplishing concrete scientific progress to make future AI what they think will be most beneficial to humanity.
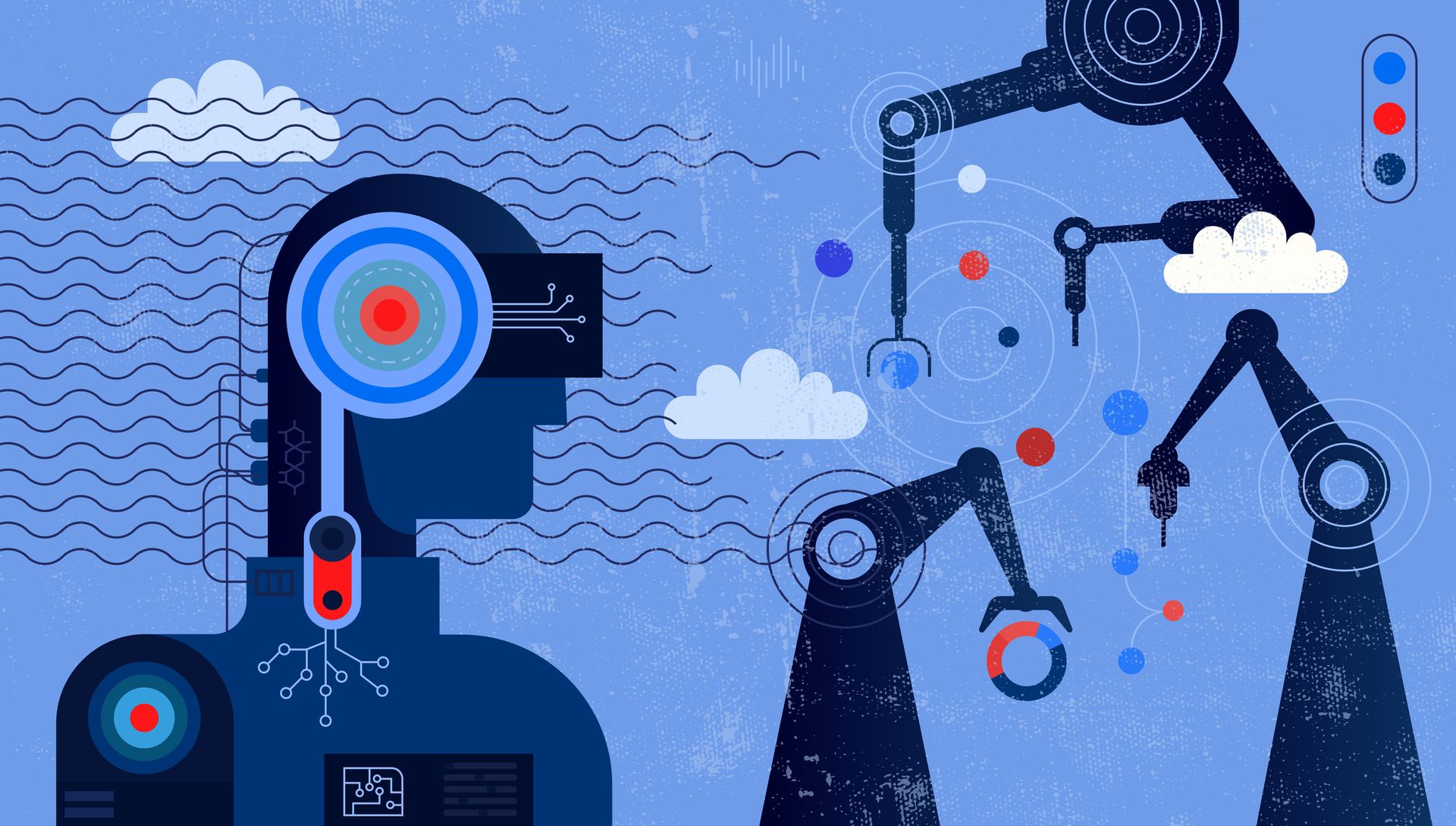
Even today, we have not come close to the goal of “Artificial General Intelligence” (AGI), which theoretically possesses all the human mind’s capabilities. Now, there are difficult but vital questions about artificial intelligence, such as how much more time is needed for artificial general intelligence to become a reality at the current pace of development? Will the AIs of the future work similarly to the human brain, or will we find a better way to build smart machines by then?
Starting from the 14th century, theorists assumed that smart machines could one day think in much the same way as we do. The main reason for adopting this idealistic goal is that we do not recognize a greater cognitive power than the human brain. The human mind is an amazing device for achieving high levels of cognitive processing. Recently, however, considerable debates and schools of thought have emerged about achieving artificial general intelligence and the best way to achieve this goal. Significant advances in deep learning, particularly inspired by the human brain but diverging from it in some key points, support new ideas that there may be other ways to achieve artificial general intelligence and much more than that.
What is Artificial General Intelligence (AGI)?
Artificial general intelligence idea envisions machines that can think and learn the same way as humans. Such a machine could understand situational contexts and apply what it has learned to complete an experience to completely different tasks.
Since the beginning of artificial intelligence as a positive research discipline in the 50s, engineers have designed many intelligent robots that can complete any task and easily switch from one to another. Ever since the first primitive examples of artificial intelligence they came up with, their dream was to one day develop machines that could understand human language, reason, plan, understand, and show common sense.
What have we achieved so far?
Think about it, we want to create virtual entities with all the mental abilities of a human, but at this point, the world’s smartest artificial intelligence cannot even match wits with a 3-year-old child. For example, while an infant can instinctively apply his experience to other areas without an ordeal, modern artificial intelligence samples, one of the most advanced products of human intelligence, often turn into fish out of water when faced with a task they are not exclusively trained in.
Researchers are on top of this and working on challenges undermining the development of artificial general intelligence. Several approaches aiming to replicate some aspects of human intelligence, mostly focusing on deep learning, seem in vogue. Foremost among these, neural networks are considered the most advanced technology for learning correlations in training datasets.
Reinforcement learning is a powerful tool for machines to learn to complete a task with clear rules independently. At the same time, productive competing networks enable computers to take more creative approaches to problem-solving. But only a few approaches combine some or all of these techniques. This causes today’s AI applications to be able to solve only constrained tasks, and this is the biggest obstacle to artificial general intelligence.
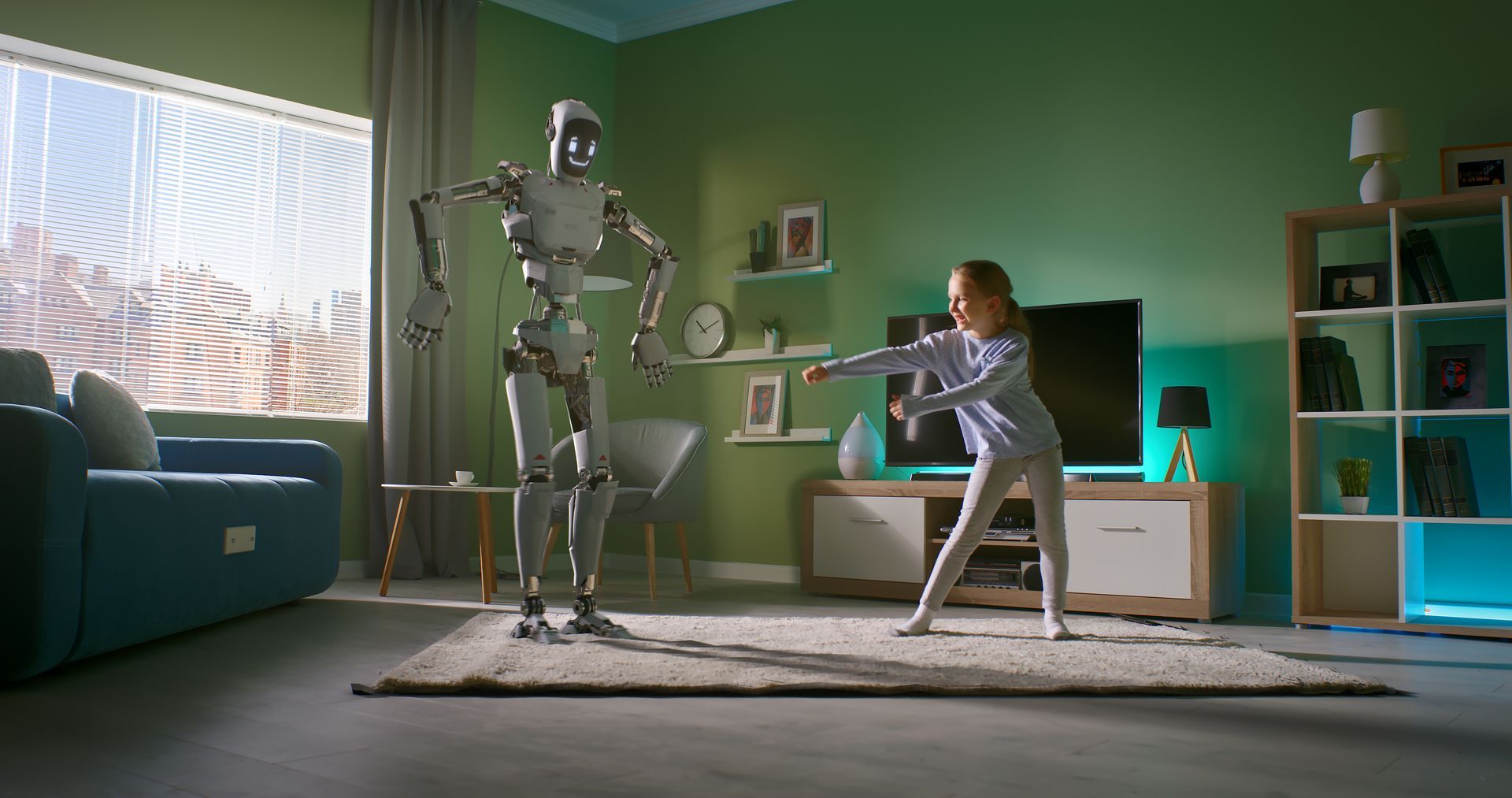
Scientific crossroads: Human-like or not, that is the question
Today’s deep learning algorithms cannot contextualize and generalize information, some of the greatest requirements for human-like thinking. Those who doubt that deep learning capabilities can lead humanity to artificial general intelligence argue that machines should not strictly try to copy the human brain’s neuron system. This school of thought believes that it is important and achievable to impart only certain aspects of the human mind to machines, such as using the symbolic representation of information to make predictions by spreading knowledge over a wider set of problems.
The biggest barriers to deep learning techniques reaching artificial general intelligence are their inability to add reasoning and advanced language processing capabilities to machines. While deep learning allows training algorithms with labeled data, it cannot fetch the deep knowledge needed for artificial general intelligence to machines.
Deep learning has difficulty reasoning or generalizing information because algorithms only know what is shown. It takes thousands or even millions of tagged photos to train an image recognition model. But even after feeding all this training data, the AI model cannot perform different tasks such as natural language understanding.
This school of thought does not advocate moving away from deep learning despite its limitations. Instead, they believe inventors should look for ways to combine deep learning with classical approaches to artificial intelligence. These include using more symbolic interpretations of data, such as knowledge graphs. Knowledge graphs use deep learning models to understand how people interact with information and improve over time while contextualizing data that connects semantically related pieces of data.
The idea of artificial general intelligence envisages that technology will ultimately benefit people and make a difference in the world. This school of thought advocates that today’s productized development of artificial intelligence is far from contributing to the great idea of artificial general intelligence. According to them, it is necessary to focus on building systems with deep knowledge, not deep learning to achieve artificial general intelligence.
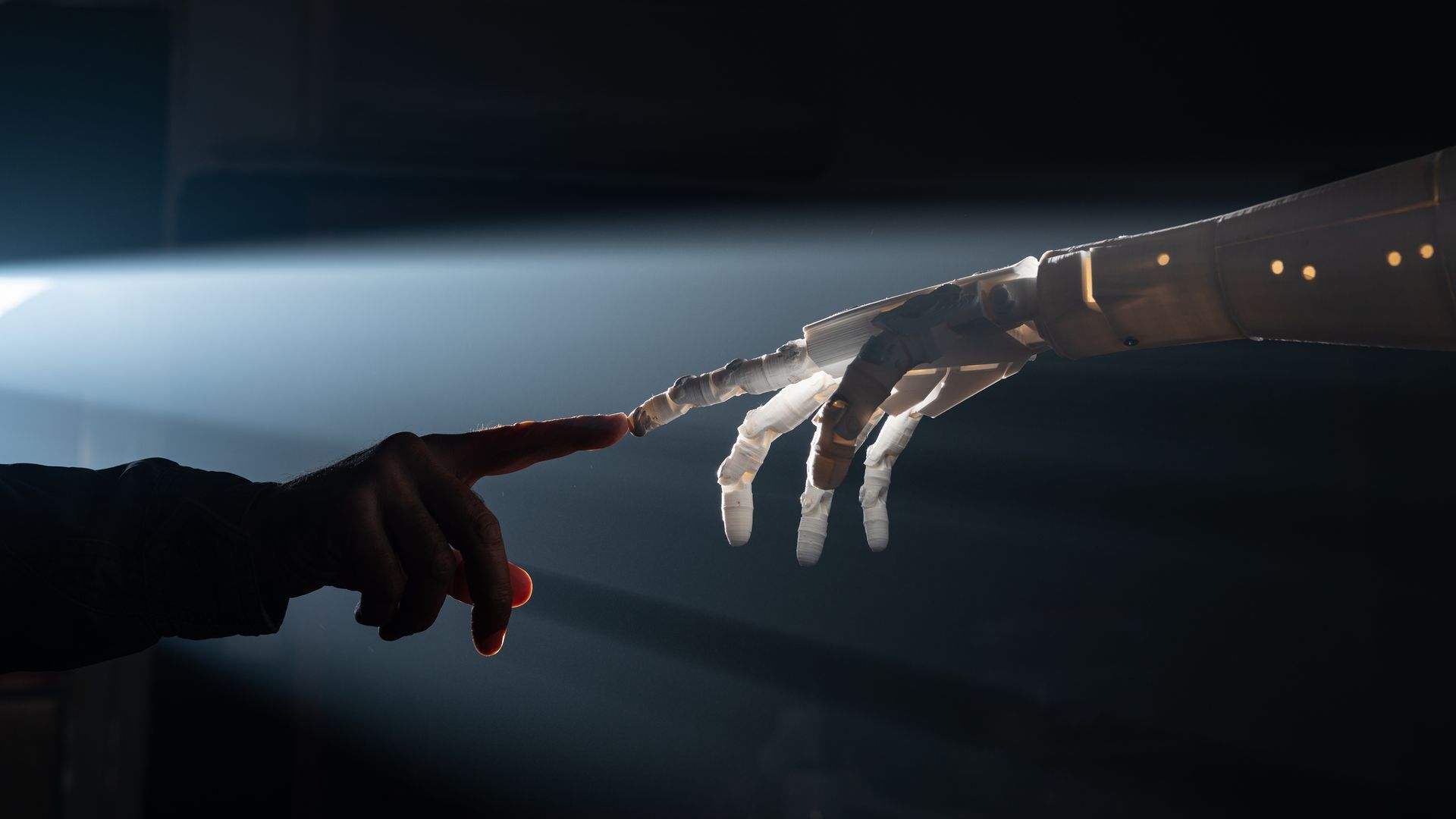
Why not godlike?
The idea that deep learning can give machines superhuman abilities opposes the idea of the school of thought we have explored. According to the opponent school, efforts to replicate human-like thinking might inadvertently limit the future capabilities of machines. Deep learning models work on different tracks than the human brain; given enough data and computational power, it is impossible to say how far they can go.
Some scientists argue that the ability of deep learning techniques to impart superhuman abilities to artificial intelligence models should not be overlooked. They point out that machines can learn abstractions that humans cannot interpret when fed with enough data.
Reinforcement learning, a discipline of deep learning, could be a promising path to improving general intelligence. These algorithms work similarly to the human mind when learning new tasks. Excitingly, the findings suggest that machines can demonstrate the ability to generalize what they have learned from one task to another in experiments in synthetic environments.
According to this current of thought, the biggest obstacle to artificial general intelligence is the speed of the training processes of deep learning models. Still, it is believed that innovators can overcome this problem. This school thinks it will be key in efforts to optimize the datasets the models are working on so that algorithms don’t need to see millions of instances to find out what is going on. However, we have limited data and processing power today, and deep learning has not yet reached its maturity stage.
As you can see, the future of artificial intelligence is so bright; humanity which once tried to raise humans in a jar, now believes that it is possible to create more intelligent and advanced beings than ourselves. I want to believe this idea because I highly doubt that more human thinking will make the world a better place.
Although the schools of thought we have examined today offer some hypotheses about the future of artificial intelligence, the actual decider will be what will be seen as more useful and needed by decision-makers at the time. And that will be determined by the level of progress of our not-so-great civilization.