Researchers have now trained a machine learning system to detect earthquakes using tiny gravitational signals, showing how they may be used to pinpoint the position and extent of a major quake in near-real-time. Earthquakes have the power to alter Earth’s gravitational field at a colossal scale.
Scientists say this is a significant step towards developing a warning system for the world’s most powerful earthquakes, according to May 11 research published in Nature.
Gravity signals are used to train machine learning
A system like this might be abletect earthquakes and could help solve a massive problem in seismology: Determining the correct magnitude of a massive quake as soon as it happens, according to Andrea Licciardi, a geophysicist at the Université Côte d’Azur in Nice, France. It’s considerably more difficult to deliver timely and effective hazard warnings if you don’t have that ability which is vital because it could save thousands of lives.
The shaking and quivering transmit seismic waves through the ground, which are detected as large wiggles on seismometers after large earthquakes occur. However, current seismic wave-based systems that we use to detect earthquakes have difficulty distinguishing between magnitudes 7.5 and 9 in the few seconds following an occurrence. Geophysicists are not the only ones to utilize artificial intelligence in their research, chemists developed a new ML framework to improve catalysts.
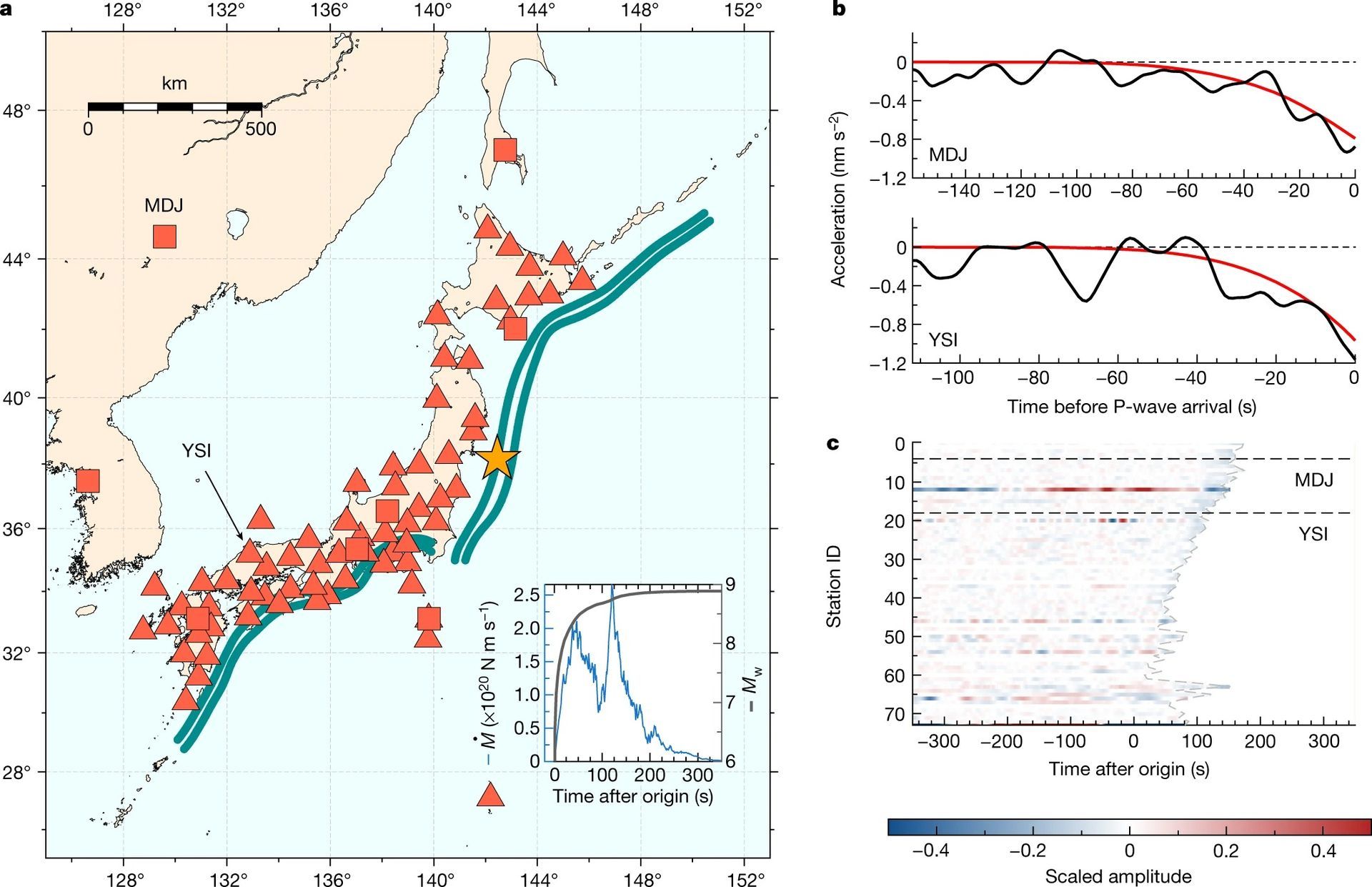
Currently, scientists detect earthquakes based on the P wave heights
This is the case because the P wave heights of seismic waves, which are the first to arrive at monitoring stations, are used in the initial estimates of the magnitude. However, for strong earthquakes, those initial P wave amplitudes peak out when they reach their maximum amplitude, making it difficult to distinguish between quakes of various magnitudes.
The earliest indications of a quake aren’t seismic waves. All of that mass shifting in a major earthquake also changes the surrounding rocks’ density. The vibrations from these waves are what seismologists use to detect earthquake epicenters. They don’t arrive immediately before the quake; instead, they’re generated by tectonic plates moving against one another or shifting due to earthquakes. These tiny changes in gravity manifest as “elastogravity” waves that travel through the ground at the speed of light — even faster than seismic waves — caused by plate movement.
Small, subtle signals known as elastogravity waves were once thought to be impossible to detect, according to seismologist Martin Vallée of the Institut de Physique du Globe de Paris, who was not part of the new study. In 2017, Vallée and his colleagues became the first group to identify these elastogravity signals in seismic station data. “You have a window in between the start of the earthquake and the time you receive the [seismic] waves,” Vallée explained.
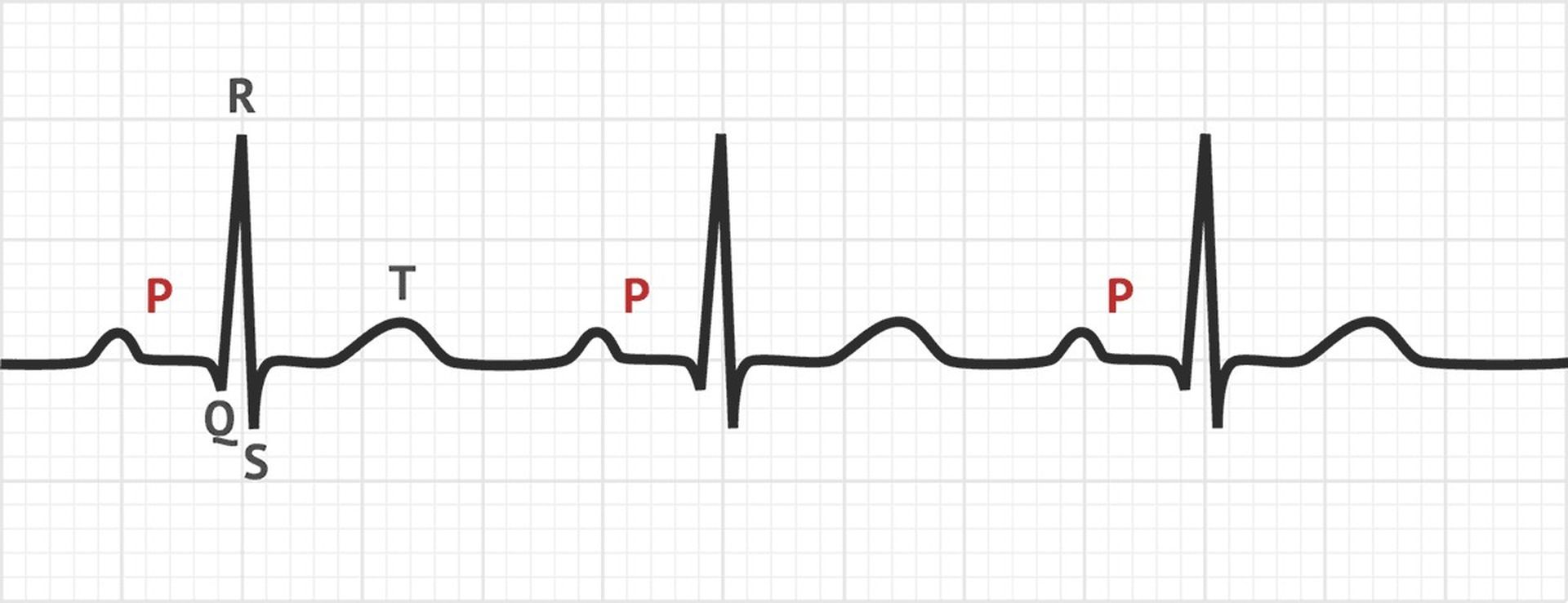
How do elastogravity signals turn into a useful set of data?
Researchers still wanted to figure out how to convert these elastogravity signals to a system that can detect earthquakes. Because the wiggles in gravity are so tiny, they’re tough to spot amid background noise in seismic data. When researchers examined the records later, they discovered that just six mega-earthquakes over the previous 30 years had been identified by elastogravity signals, including the magnitude 9 Tohoku-Oki earthquake of 2011, which triggered a widespread tsunami that flooded two nuclear power plants in Fukushima, Japan.
According to Licciardi, this is where computers come into play. A new machine learning network called PEGSNet is designed to identify “Prompt ElastoGravity Signals.” The algorithms were trained on a mix of actual seismic data from Japan and 500,000 simulated gravity signals for earthquakes in the same area. Because real data are so rare, synthetic gravity data are critical for training machine learning systems to detect earthquakes. Any input is vital. If you want to learn more about training machine learning, check out the science of building reliable AI systems.
Then, the machine learning system was put to the test. The aim was to simulate the 2011 Tohoku earthquake in real-time and keep track of its origins and progress. The result was promising, Licciardi says. The algorithm accurately identified both the magnitude and location of the quake five to 10 seconds earlier than other methods.
According to Licciardi, this test is a proof of concept, and it may serve as the foundation for a prototype early warning system. According to the researchers, PEGSNet has the potential to be a highly useful tool for detecting earthquakes, especially when combined with other earthquake-detection technologies.
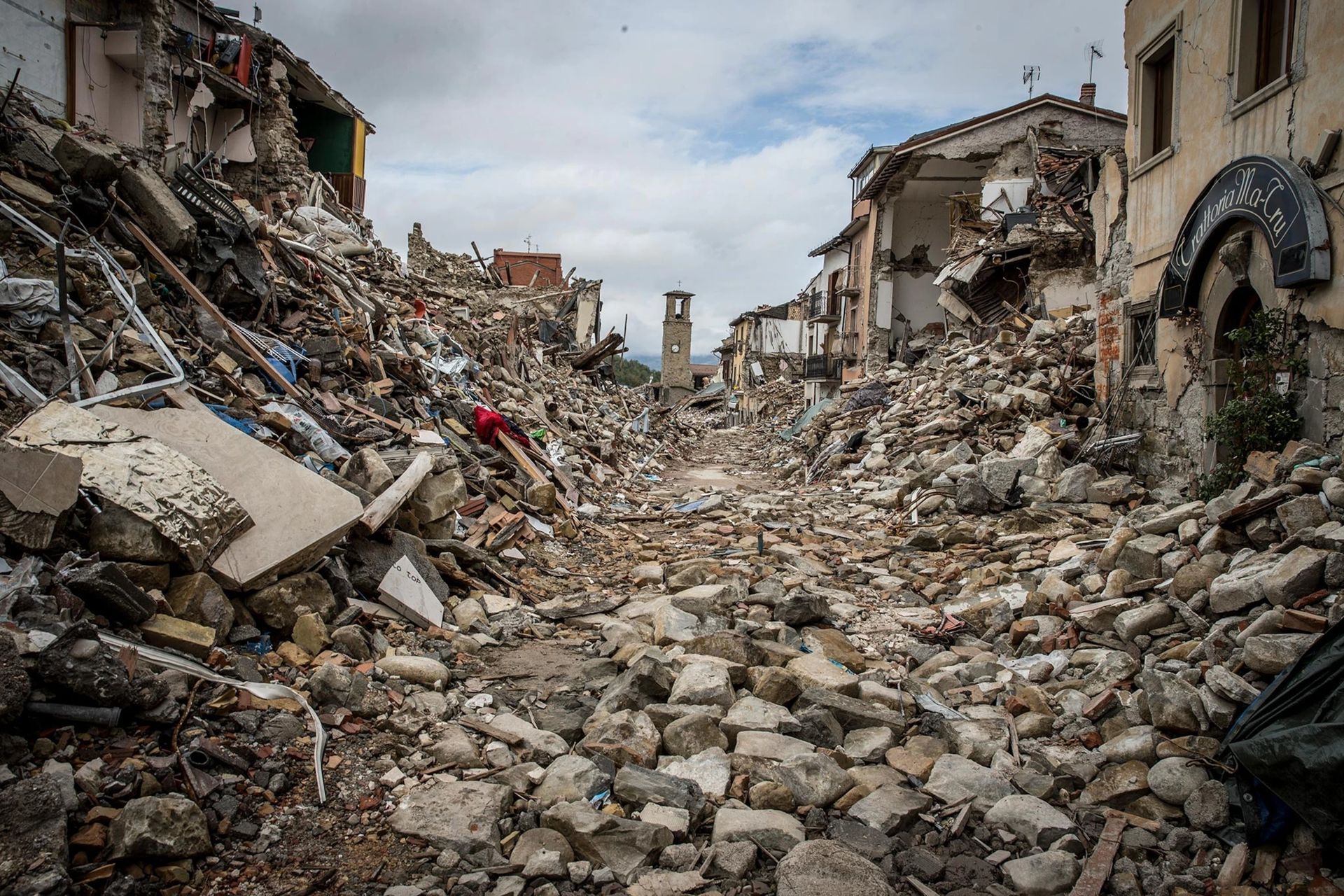
Nonetheless, there is still more work to be done. One issue is that the algorithm was trained to seek a single point for an earthquake’s origin, which is a reasonable assumption if you’re far away. But when you look close up, the origin of a quake no longer appears to be a point; it’s a larger area that has fractured. If scientists want accurate knowledge of where a fracture will occur in the future, machine learning systems must search for areas rather than points.
Larger advances may be on the way as scientists develop more sensitive instruments to detect earthquakes. Even tinier earthquake-caused waves change the gravitational field while filtering out other noise sources. Earth, according to Vallée, is a noisy place, from its seas to its atmosphere.
“It’s a bit the same as the challenge that physicists face when they try to observe gravitational waves,” Vallée explained. If you are into machine learning and artificial intelligence, check the history of Machine Learning, it dates back to the 17th century.