Deep science related efforts are enabling new initiatives, this new AI simulates economies and predicts which kind of startups receive funding. The breadth of research in the field of machine learning and AI, which is now a crucial technology in almost every sector and company, is simply too big for anyone to comprehend. In this article we intend to gather some of the most significant recent discoveries and papers – particularly those pertaining to artificial intelligence – and explain why they are important.
Researchers trained systems to understand the influence of interactions between platforms and consumers
Scientists conducted an intriguing research this week to forecast how “market-driven” platforms like food delivery and ride-hailing businesses would effect the entire economy when optimized for various goals, such as maximizing profit. In addition to this the researchers at ETH Zurich have developed the first high-resolution global vegetation height map for 2020 from satellite photos using an artificial neural network.
This market-driven platform is built on AI Economist of Salesforce which is an open search research community aiming to understand how artificial intelligence could cultivate economic policies. In fact, some of the AI Economist’s creators were part of the new research, which was documented in a March paper.
The goal of the study, according to the coauthors, was to look at two-sided marketplaces like Amazon, DoorDash, Uber and TaskRabbit that have more market power as a result of increasing demand and supply. The researchers trained a system to understand the influence of interactions between platforms and consumers using reinforcement learning — a method of artificial intelligence that learns by trial and error to solve complex problems.
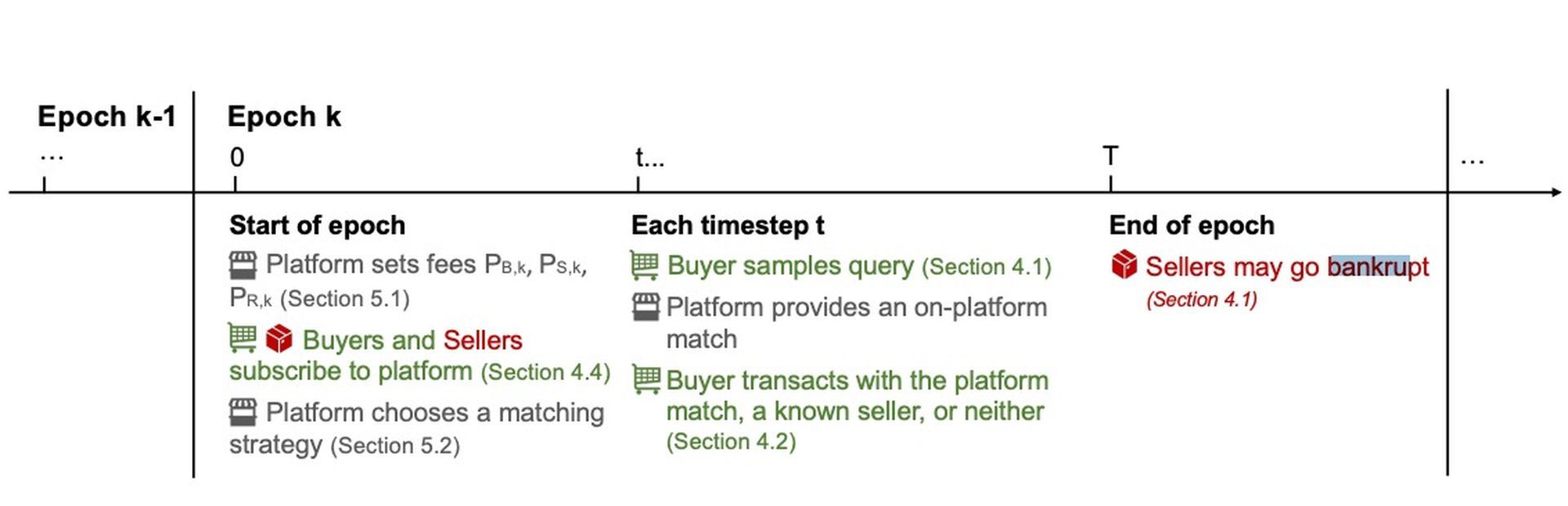
“We use reinforcement learning to reason about how a platform would operate under different design objectives … [Our] simulator enables evaluating reinforcement learning policies in diverse settings under different objectives and model assumptions. We explored a total of 15 different market settings — i.e., a combination of market structure, buyer knowledge about sellers, [economic] shock intensity and design objective,” the coauthors told TechCrunch.
AI simulates economies and gives accurate outcomes, but how?
The researchers reached the conclusion that a platform designed to maximize earnings increases charges and takes more money from buyers and sellers during economic downturns at the expense of social well-being. When platform costs are set by regulation, they discovered that a platform’s revenue-maximizing incentive usually coincides with the overall economy’s welfare considerations.
The study’s authors suggest that the method — which they intend to make public – might be used by a business or a policymaker to analyze a platform economy under various conditions, designs, and regulatory restrictions.
“We adopt reinforcement learning as a methodology to describe strategic operations of platform businesses that optimize their pricing and matching in response to changes in the environment, either the economic shock or some regulation. This may give new insights about platform economies that go beyond this work or those that can be generated analytically,” they explained.
Researchers from Skopai, a startup that uses AI to evaluate businesses based on factors like technology, market, and finances, claim to be able to predict the capacity of a firm to attract investments using freely available data. The authors claim that they are able to obtain prediction results “comparable to the ones making also use of structured data available in private databases.”
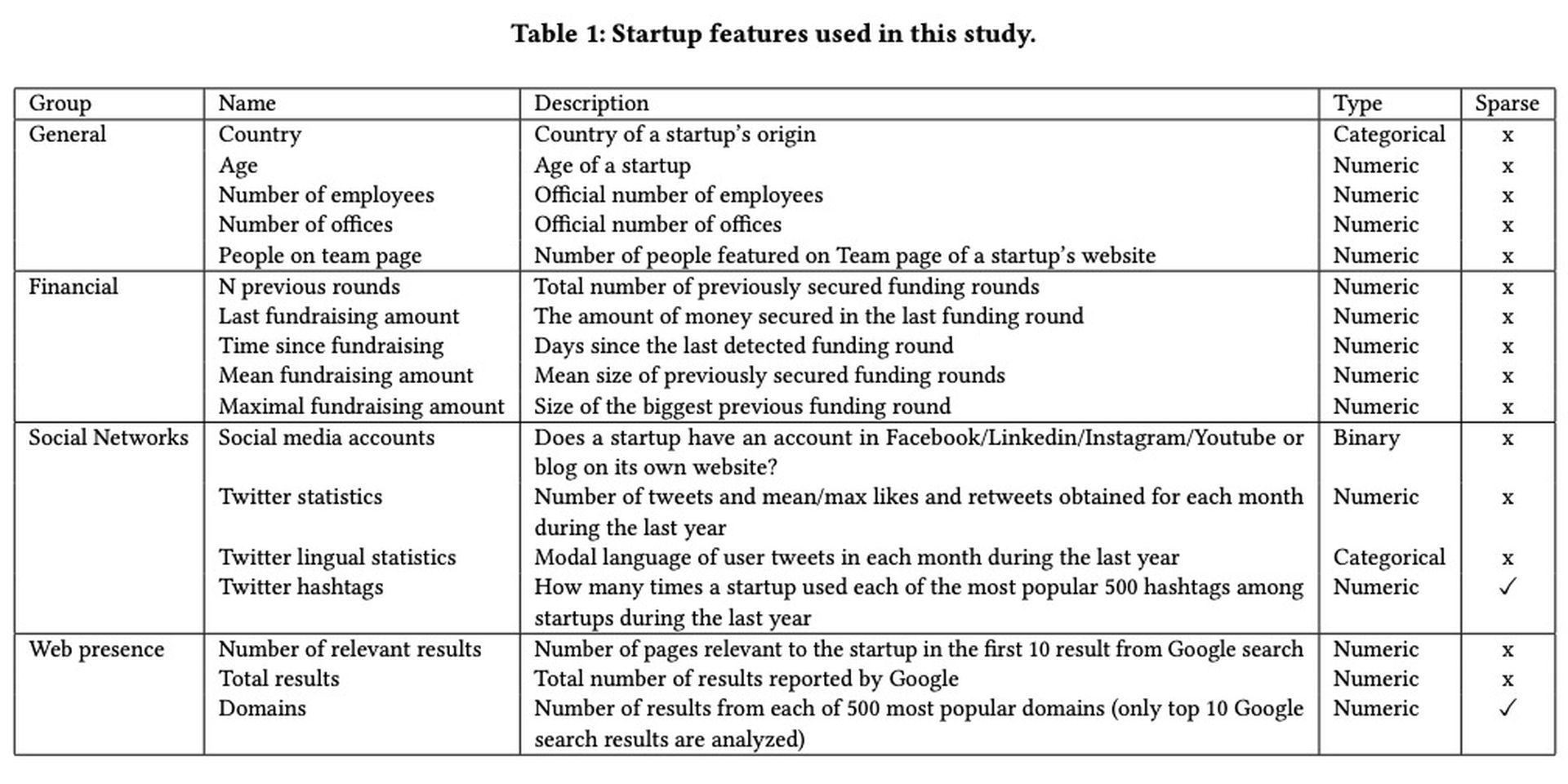
AI has been used to supplement due diligence for some time. Correlation Ventures, EQT Ventures, and Signalfire are among the firms that are presently employing algorithms in their investments. According to Gartner, 75% of venture capitalists will employ AI in decision-making by 2025, up from less than 5% now.
While some see the potential of the technology, others do take risks seriously. An investment algorithm outperformed novice investors but had biases, according to Harvard Business Review (HBR) in 2020. The bias reflects reality, HBR says, calling attention to AI’s propensity to exacerbate existing prejudices.
Traditionally, computer vision algorithms have been trained using many examples of the objects that have been categorized by people. STEGO eliminates the time-consuming, labor-intensive process outlined above by applying a class label to each pixel in the image rather than relying on human labels. The technology isn’t flawless but STEGO can successfully separate objects like roads, people, and street signs, according to the study authors.
Besides that, scientists at MIT, Cornell, and Microsoft have created a computer vision algorithm called STEGO (Self-supervised Transformer with Energy-based Graph Optimization) that can recognize images down to the pixel level. While this may not sound like much, it is an improvement over the traditional method of teaching an algorithm to recognize and categorize objects in photographs and videos.
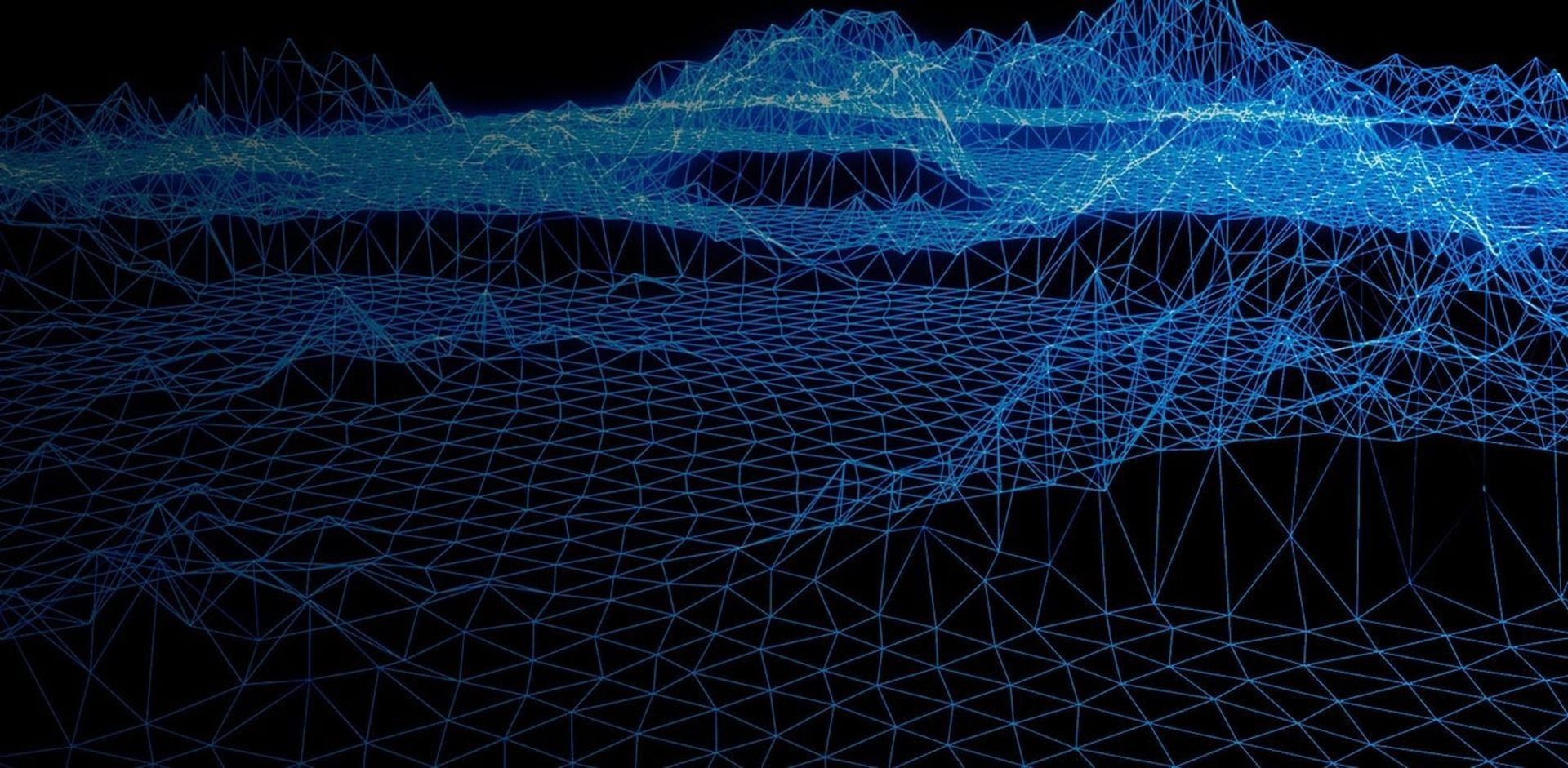
On the subject of object recognition, it appears we’re approaching the day when academic research like DALL-E 2, OpenAI’s image-generating mechanism, becomes productized. For example, researchers at the Gwangju Institute of Science and Technology also developed the UOAIS method that enables AI to detect occluded objects. Another study from Columbia University shows a technology called Opal that produces highlighted images for news stories based on textual descriptions and uses visual cues to guide users through the process.
When academics tested Opal with a group of participants, they found that those who used it were “more efficient” in generating featured images for articles, creating almost twice as many “usable” results as users who didn’t use it. It’s easy to picture something like Opal finding its way into content management systems like WordPress, perhaps as a plugin or extension.
“Given an article text, Opal guides users through a structured search for visual concepts and provides pipelines allowing users to illustrate based on an article’s tone, subjects and intended illustration style, Opal generates diverse sets of editorial illustrations, graphic assets and concept ideas,” the coauthors wrote.