Researchers developed a new model in order to enable AI to detect occluded objects. These systems must make assumptions based only on the parts of things that are visible in situations where objects are not entirely visible. This partial information causes detection mistakes, and large training data is required to accurately recognize such settings. Researchers at the Gwangju Institute of Science and Technology have now devised a method for robot vision to spot similar items in the same way that we do.
Robotic vision needs to be trained in order to detect occluded objects
When AI systems encounter situations where objects are only partially visible, they must rely on just the visible portions of the items. This limited knowledge leads to detection mistakes and necessitates a large amount of training data to correctly recognize such items.
Robotic vision has progressed a long way in recent years, with applications in complex and demanding roles such as autonomous driving and object manipulation. It still has trouble recognizing distinct items in cluttered scenes where some objects are partially or completely hidden by others, however. When confronted with such environments, typical robotic vision systems are taught to detect occluded objects based only on their visible features. However, this method consumes substantial amounts of data and is time-consuming.
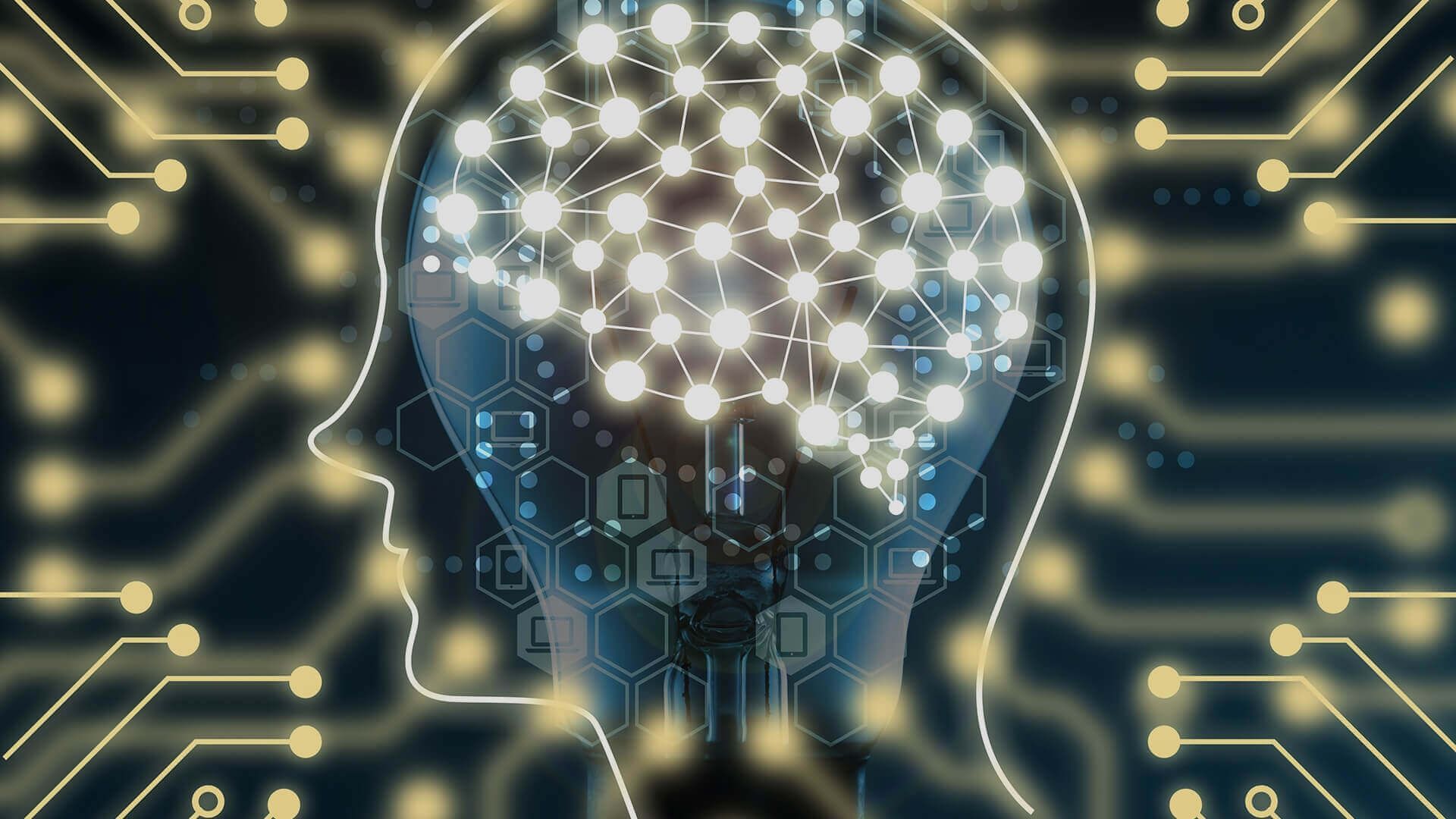
When Associate Professor Kyoobin Lee and Ph.D. student Seunghyeok Back from the Gwangju Institute of Science and Technology (GIST) in Korea were building an artificial intelligence system to categorize and sort objects in cluttered scenes, they hit a snag.
The researcher team is really excited about the project. “We expect a robot to recognize and manipulate objects they have not encountered before or been trained to recognize. In reality, however, we need to manually collect and label data one by one as the generalizability of deep neural networks depends highly on the quality and quantity of the training dataset,” Mr. Back explained.
UOAIS method could serve as a baseline on this front
Prof. Lee and Mr. Back with their research team presented a method called “unseen object amodal instance segmentation” (UOAIS) in order to detect occluded objects in cluttered settings in a new study accepted at the 2022 IEEE International Conference on Robotics and Automation.
To teach the model to recognize three-dimensional form, they generated a database of over 40,000 photorealistic synthetic images with depth data. The model was able to detect occluded objects after being trained with limited data. It first identified the main topic and then determined whether it is obscured by partitioning the object into a “visible mask” and an “amodal mask.”
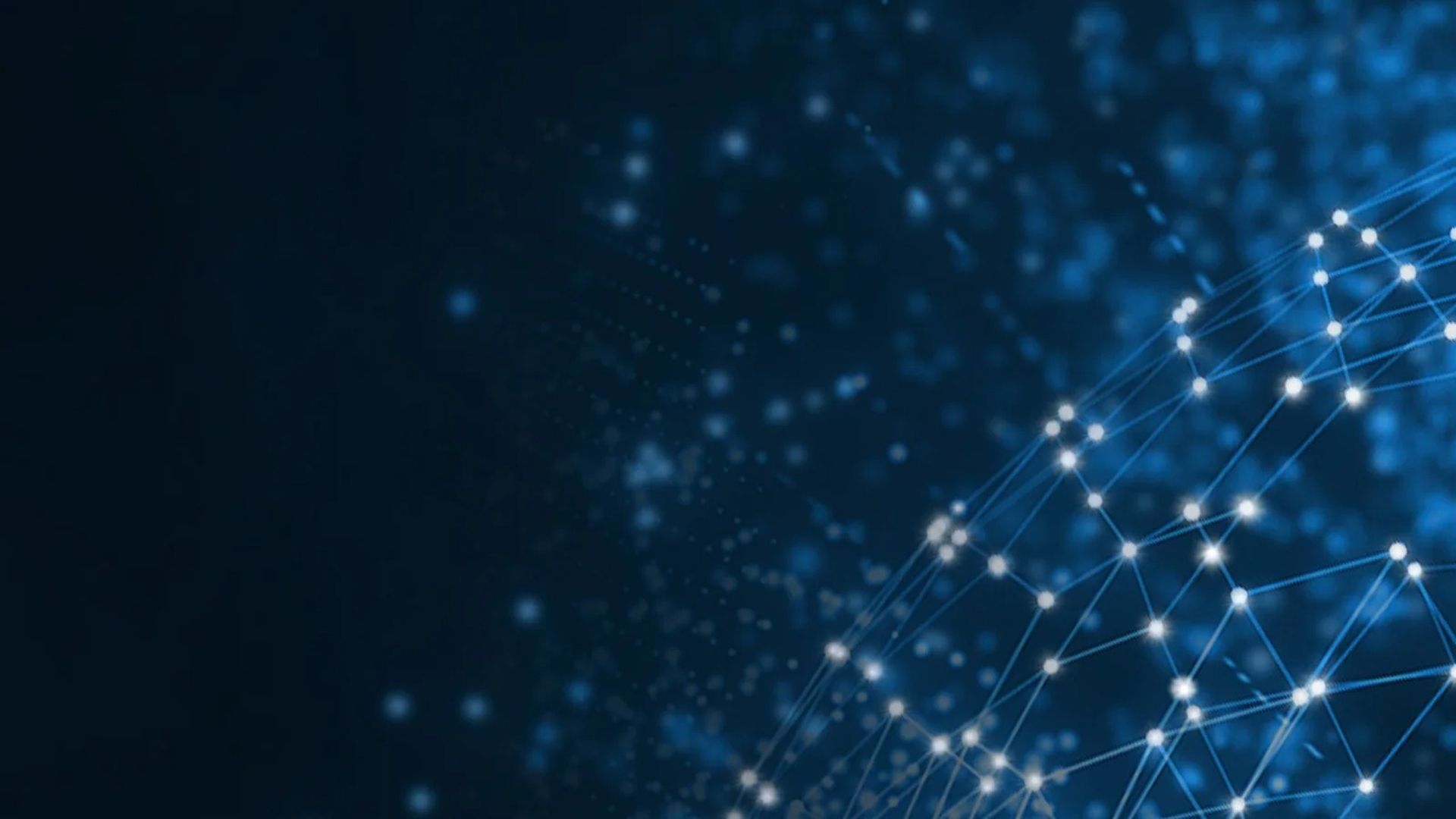
“Previous methods are limited to either detecting only specific types of objects or detecting only the visible regions without explicitly reasoning over occluded areas. By contrast, our method can infer the hidden regions of occluded objects like a human vision system. This enables a reduction in data collection efforts while improving performance in a complex environment,” Mr. Back said.
In their system, the researchers incorporated a “hierarchical occlusion modeling” (HOM) method to handle “occlusion reasoning,” which assigned a hierarchy to the mix of numerous extracted features and their prediction order. The HOM scheme was validated through three performance benchmarks by testing their model against them.
They believe that the approach may have future applications and pave the way for more interesting ideas. “Perceiving unseen objects in a cluttered environment is essential for amodal robotic manipulation. Our UOAIS method could serve as a baseline on this front,” Mr. Back explained.